Abstract
Metabolic syndrome (MetS) is a complex disorder characterized by a set of interrelated metabolic abnormalities, such as central obesity, hypertension, dyslipidemia, and insulin resistance. It constitutes a major public health problem worldwide due to its association with an increased risk of cardiovascular disease, type 2 diabetes mellitus (T2DM) and other chronic diseases. Biomedical engineering (BME), through its interdisciplinary nature, has contributed significantly to the understanding, diagnosis, and treatment of MetS. The aim of this review article is to provide a comprehensive overview of the current state of research and advances in BME approaches to the study and management of MetS. The article will delve into diverse approaches, including computational and omics models, that have been used to improve our understanding of MetS. In addition, it will provide an overview of specialized devices that have been designed for the non-invasive assessment of individuals with MetS.
Keywords
1. Introduction
Metabolic syndrome (MetS) is a complex and increasingly prevalent disease that encompasses a set of interconnected metabolic risk factors[1]. This multisystem syndrome significantly increases the risk of developing severe health complications, such as cardiovascular disease (CVD)[2,3], type 2 diabetes mellitus (T2DM)[4] and even certain types of cancer[5,6]. The diagnostic criteria for MetS typically include abdominal obesity, as defined by waist circumference measurements, combined with specific thresholds for other components such as elevated blood pressure, altered glucose metabolism (e.g., elevated fasting glucose or insulin resistance (IR), and abnormal lipid levels (e.g., elevated triglycerides and low HDL-cholesterol)[7]. Specific cut-off values for each component may vary slightly depending on the guidelines used, including those provided by organizations such as the National Cholesterol Education Program Adult Treatment Panel III (NCEP ATP III) or the International Diabetes Federation (IDF) (Table 1)[7]. The increasing global prevalence of MetS has become a major public health problem, primarily promoted by sedentary lifestyles, unhealthy dietary habits and genetic predisposition[8].
Criteria | NCEP ATP III | IDF |
Abdominal obesity | Waist circumference Men: ≥ 102 cm (> 40 in) Women: ≥ 88 cm (> 35 in) | Waist circumference Men: ≥ 94 cm Women: ≥ 80 cm |
Elevated blood pressure | ≥ 130 mmHg systolic or ≥ 85 mmHg diastolic Or Pharmacological treatment | ≥ 130 mmHg systolic or ≥ 85 mmHg diastolic Or Pharmacological treatment |
Altered glucose metabolism | Fasting glucose ≥ 100 mg/dL Or Pharmacological treatment | Fastin glucose ≥ 100 mg/dl |
Abnormal lipid levels | Triglycerides > 150 mg/dL Or Pharmacological treatment | Triglycerides > 150 mg/dl Or Pharmacological treatment |
HDL cholesterol Men: < 40 mg/dL Women: < 50 mg/dL Or Pharmacological treatment | HDL cholesterol Men: < 40 mg/dl Women: < 50 mg/dl Or Pharmacological treatment | |
Required criteria | Any three of the five criteria | Abdominal obesity plus any two of the four criteria |
IDF: International Diabetes Federation; MetS: metabolic syndrome.
BME has a profound impact on MetS by revolutionizing the diagnosis, treatment, and understanding of this complex condition. Through the development of advanced imaging techniques, such as magnetic resonance imaging and computed tomography (CT), biomedical engineers enable accurate and non-invasive assessment of MetS components Overall, BME advancements have the potential to transform the understanding, diagnosis, and management of MetS, leading to more effective and personalized healthcare strategies for individuals affected by this condition[9-11]. Understanding the underlying mechanisms, risk factors and potential interventions against MetS is crucial to address this burgeoning public health problem and promote global wellness.
Recent reviews in the field of BME have highlighted innovative data integration approaches that hold great promise for advancing our understanding of MetS. These reviews highlight the importance of integrating data from diverse sources, such as genomics, metabolomics, proteomics, and clinical parameters, to gain a comprehensive view of the complex interactions underlying the MetS. Using advanced computational techniques, machine learning algorithms, and systems biology approaches, these studies highlight the potential to unravel intricate metabolic pathways and identify new biomarkers that could improve early detection and personalized treatment strategies for MetS (Table 2)[9,10,12-15].
Approach | Summary | Reference |
Development of prediction models for MetS based on whole genome sequencing data. | The relationship between SNP of circadian clock genes and the development of MetS was studied. A prediction model for metabolic syndrome was constructed using logistic regression, random forest, adaboost and neural network. | [16] |
Use of computational models to describe long-term development of MetS in APOE3L. CETP mice fed with a high-fat diet. | Computational modelling of energy balance in individuals with Metabolic Syndrome. MINGLeD was utilized in combination with analysis of ADAPT to achieve a model library describing various phenotypes to analyze energy expenditure and energy balance. | [17] |
Validation of artificial AI-based scores for MetS prediction. | Different ML algorithms were trained to predict the MetS status of each subject according to clinical and biochemical data. Subsequently, a module was used to explain the developed models and thus extract new scores for MetS detection. | [18] |
Combination of multi-omics and clinical information to characterize metabolic diseases molecularly and clinically. | Integration of metabolomics, proteomics, peptidomics and clinical information to elucidate latent molecular hallmarks and interrelationships of multiple metabolic diseases, including MetS, hyperglycemia, hypertension and T2DM. | [19] |
Use of genetic and metabolomic analysis to identify metabolite biomarkers of MetS. | Multi-stage metabolomics analysis was used to identify metabolite biomarkers and subsequently construct a metabolite risk score for MetS. Subsequently, two-stage GWAS analyses were used to identify SNPs associated with the metabolites. | [20] |
MetS: metabolic syndrome; SNP: single nucleotide polymorphism; MINGLeD: model INtegrating glucose and lipid dynamics; ADAPT: analysis of dynamic adaptations in parameter trajectories; ML: machine learning; T2DM: type 2 diabetes mellitus; GWAS: genome-wide association studies.
2. Biomedical Engineering Approaches for Understanding Metabolic Syndrome
BME employs computational modeling and simulation techniques to enhance the understanding of MetS. Through the integration of data from genetics, omics technologies and clinical parameters, computational models can effectively capture the intricate interactions and dynamics occurring in the MetS (Figure 1)[21,22]. The development of MetS is characterized by complex interactions and feedback loops encompassing multiple time and space scales, from cellular to organismal levels[14]. Computational modeling techniques, such as constraint-based modeling and kinetic modeling, can be applied to these metabolic networks to simulate and analyze the metabolic perturbations associated with MetS[23]. These models make possible the simulation of the impact of genetic variations, lifestyle modifications and pharmaceutical treatments on MetS-related outcomes.
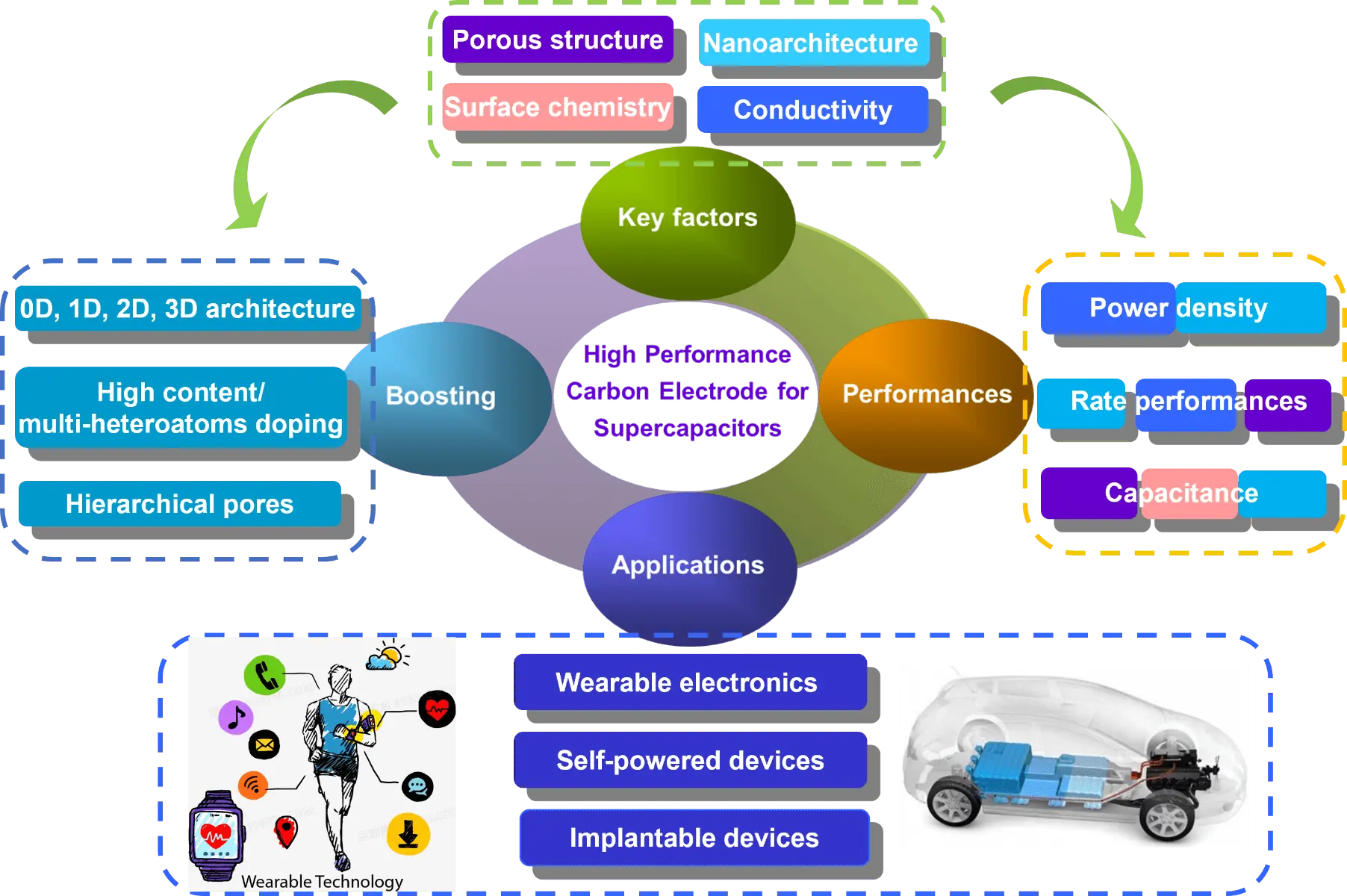
Figure 1. Computational models for the understanding of MetS. MetS: metabolic syndrome.
Computational models are promising tools for understanding the complex dynamics underlying MetS. These models employ mathematical and computational techniques to simulate and analyze the intricate interactions between various molecular and physiological components that contribute to the development and progression of MetS.
2.1 Constraint-based models
In the 1980s, several data analysis approaches were developed to cope with the increasing amount of biological data available at that time, one of these approaches was the constraint-based models (CBM)[24]. Initially, the CBMs used mathematical algorithms to determine theoretical yields of metabolic pathways and metabolite overflows[25]. Studies conducted at that time showed a good concordance between CBM predictions and measured cell behavior[26]. Leading the way for predicting phenotypes from a biochemically reconstructed network. Decades later, CBMs started to be used in the analysis of genotype-phenotype relationships, based on the principle that biological organisms function within the limits and constraints imposed by their genetic and environmental components[27]. This approach facilitated the study of the global organization of cellular behavior, such as the structure of metabolic pathways[28] and metabolic fluxes[29]. CBMs can be utilized to study metabolic pathways and analyze how perturbations in these pathways might contribute to metabolic disorders. Several models have been designed to explain the development of metabolic dysfunction in multiple diseases[30-32].
2.2 Kinetic models
The kinetic models were designed with the intention of addressing the limitations of CBMs[33]. They provide a distinct advantage in predicting the actions of living organisms under conditions far away from equilibrium. As a result, they extend the computational capacity to encompass cellular physiology, going beyond the steady-state assumption of CBMs[34]. Kinetic models are focused on describing the dynamic behavior of metabolic reactions using detailed mathematical equations that incorporate enzyme kinetics, metabolite concentrations, and regulatory factors[35]. Through simulating the kinetics of specific enzymes and reactions, kinetic models can elucidate the underlying mechanisms that contribute to metabolic dysregulation in MetS[36].
2.3 Model INtegrating Glucose and Lipid Dynamics (MINGLeD)
Recently, a new model has been designed, combining both in vivo and in silico models. A new data-driven physiological model called MINGLeD has been developed to describe glucose, lipid and cholesterol metabolism. This model allowed the identification of distinct phenotypes inside the MetS and provided information on the underlying metabolic dysregulations by describing the development and long-term progression of the MetS[17,37].
Computational models, including the MINGLeD model, present an innovative approach to understanding MetS, demonstrating several remarkable novelties and advantages in contrast to conventional methods[13,17]. Firstly, these computational models work dynamically and comprehensively, capturing the intricate interplay of diverse metabolic pathways and their effects on the MetS[27,34,36]. Unlike traditional static approaches, these models consider the dynamic nature of metabolic pathways, allowing for a more accurate representation of the underlying physiological dynamics in MetS[27,33]. Furthermore, the MINGLeD model specifically introduces a new dimension by integrating multiple omics data sources, such as genomics, metabolomics and lipidomics. This integration enhances a holistic understanding of MS by elucidating the complex relationships between genetic factors, metabolic intermediates, and lipid profiles. This approach facilitates the identification of previously missed biomarkers, potential therapeutic targets, and mechanisms insights that would be hidden by traditional methods of single data analysis[17,37,38].
2.4 Omics
In addition to the previously mentioned models, a new field of research has been added. The omics refers to a set of comprehensive techniques used to study various biological molecules, including genomics (study of genes)[39], transcriptomics (study of gene expression)[40], proteomics (study of proteins)[41], metabolomics (study of small molecules)[42] and epigenomics (study of chemical modifications of DNA)[43]. They provide a holistic view of the molecular alterations and interactions that occur in a biological system, shedding light on the underlying pathophysiology of diseases such as MetS[44]. Scientists have identified numerous genetic variants (FTO, TCF7L2, MC4R, etc.) associated with the development and progression of MetS disease through large-scale genomic studies and advanced sequencing technologies[45]. These gene variants are implicated in the regulation of body weight, energy balance and glucose metabolism. The study of these genetic markers provides a better understanding of the molecular mechanisms underlying MetS and allows researchers to identify potential therapeutic targets[19].
2.5 Metabolomics
Another outstanding field that has improved the understanding of MetS is metabolomics. Metabolomic studies have revealed distinct metabolic profiles in individuals with MetS compared to healthy individuals. These profiles often show alterations in metabolites involved in lipid metabolism, glucose, aminoacids and oxidative stress[46]. One of the objectives of metabolomics is to identify metabolites that could function as biomarkers of MetS. Recently a study identified metabolites associated with MetS, using LysoPC (14:0), LysoPC (15:0), propionyl carnitine, phenylalanine, and docosapentaenoic acid to construct a metabolite risk score to assess the risk of MetS. This score showed to have a dose-response relationship with MetS and metabolic abnormalities[20]. Globally, the use of omics approaches in MetS research provided valuable information on the genetic, molecular, and metabolic fundamentals of this complex disease. Further advancement of these technologies will hold significant potential for improving risk prediction, diagnosis, treatment, and prevention strategies, leading eventually to enhanced health outcomes for people affected by MetS.
3. Biomedical Engineering Tools for Assessing Metabolic Syndrome
BME tools have a crucial role in the assessment of MetS. They cover a wide range of technologies and techniques designed to measure and assess various parameters associated with MetS. For example, body composition analyzers (BCAs), such as bioelectrical impedance analysis (BIA)[47] and dual-energy X-ray absorptiometry (DEXA)[48], provide information on body fat percentage, muscle mass and visceral fat levels. Furthermore, continuous glucose monitoring (CGM)[49] systems allow blood glucose levels to be monitored over time, providing valuable data on glucose metabolism and diabetes risk. Beyond glucose monitoring, blood pressure monitoring devices and cholesterol analysis tools support the assessment of hypertension and lipid abnormalities[50]. Finally, metabolic rate measurement tools, such as indirect calorimetry devices, estimate energy expenditure and metabolic rate[51]. These BME tools contribute to the early detection, diagnosis and management of MetS, allowing healthcare professionals making more informed decisions and providing personalized care to individuals at risk.
3.1 Body composition analyzers
During weight gain, body composition undergoes a transformation characterized by a higher accumulation of fat compared to lean mass. This composition change is linked to an increase in insulin resistance and the onset of MetS. Nevertheless, excessive fat accumulation is not the main metabolic risk factor, the location where fat is stored determines the risk[52]. Abdominal adiposity is a pivotal component of MetS because it contributes to the development of insulin resistance and cardiometabolic diseases through visceral adipose tissue (VAT) dysfunction and adipokine dysregulation. Anthropometric measurements do not accurately reflect visceral adiposity and cannot differentiate between subcutaneous and visceral obesity. In the last few decades, new technologies have been developed to accurately determine the internal composition of the body. BCAs provide a comprehensive body composition analysis, including measurements of fat mass, muscle mass, bone density and hydration levels[53].
One of the most used methods is BIA. Through the measurement of the impedance of electrical currents passing through the body, BIA provides valuable information on the distribution of body fat, muscle mass and water content[54]. BIA devices send a harmless electrical signal through the body and measure the resistance encountered. Using algorithms and mathematical models, these devices can estimate body fat percentage, muscle mass and other relevant parameters[55]. Multiple studies have evaluated the use of BIA in MetS assessment. These studies have demonstrated that the measurement of VAT using BIA is a useful method for predicting the risk of MetS. In addition, it has been observed that total body fat and visceral fat levels are higher in subjects with MetS[47,53,56,57]. BIA offers a convenient and accessible way to monitor changes in body composition over time, making it a valuable tool for people who want to improve their health and fitness.
Individuals with MetS are at increased risk for osteoporosis and fractures because this condition is commonly associated with hormonal imbalances, reduced physical activity and vitamin D deficiencies[58]. DEXA a non-invasive imaging technique has proven to be valuable in assessing body composition and bone density. DEXA scans can detect changes in bone density, which can help in identifying individuals who may require intervention for preventing fractures and maintaining bone health[59]. While initially utilized for measuring bone mineral density, DEXA has increasingly become recognized for its role in the assessment of MetS[60]. DEXA provides accurate measurements of total body fat, lean mass, regional fat distribution and VAT quantification, factors that have been strongly associated with IR and metabolic abnormalities of MetS[61]. In addition, DEXA scanners provide an assessment of sarcopenia, an important feature of individuals with MetS, through the measurement of appendicular lean mass[62].
3.2 Glucose sensing devices
Abnormalities in glucose metabolism play a key role in the development and progression of MetS. One of the main abnormalities is insulin resistance, in individuals with IR, the cells are less responsive to the effects of insulin, resulting in elevated levels of glucose in the bloodstream. This condition typically preludes the development of T2DM[63]. IR is strongly related to another key abnormality of glucose metabolism observed in MetS: glucose intolerance. Glucose intolerance is the inability of the body to effectively metabolize glucose after consuming a meal. This leads to elevated blood glucose levels, but not high enough to fulfill the criteria for T2DM[64]. In addition to IR and glucose intolerance, individuals with MetS often have elevated fasting blood glucose levels, commonly described as hyperglycemia. Chronic elevated blood glucose levels contribute to the development of oxidative stress, inflammation and endothelial dysfunction, factors involved in the pathogenesis of CVD[65].
As mentioned previously, alterations in glucose metabolism have a major impact on the health of individuals with MetS. CGM devices provide valuable real-time information on blood glucose levels, CGM systems consist in a small sensor that is inserted under the skin and measures glucose levels in the interstitial fluid. These devices provide real-time glucose readings at regular time intervals, usually every few minutes, allowing users to monitor their glucose levels continuously throughout the day[66]. The continuous monitorization of glucose levels is particularly useful for controlling IR. Individuals with IR frequently report fluctuations in their blood glucose levels, making it difficult to achieve stable glycemic control. CGM devices provide real-time information, allowing individuals to quickly identify and effectively manage episodes of hyperglycemia or hypoglycemia[67]. The use of CGM devices must be performed in collaboration with healthcare professionals to guarantee proper interpretation of glucose readings and adequate adaptations of treatment regimens.
3.3 Non-invasive blood pressure monitoring
The IR is a key factor in the development of other components of the MetS. An example is the development of alterations in blood pressure. IR triggers impaired nitric oxide production and endothelial dysfunction. Resulting in a reduction of vasodilation and an increase in peripheral vascular resistance, contributing to elevated blood pressure levels. Furthermore, the abnormal lipid profile and abdominal obesity also contribute to these alterations by activating proinflammatory pathways, oxidative stress and release of adipokines and inflammatory mediators, respectively[65,68]. Additionally, the renin-angiotensin-aldosterone system (RAAS) is commonly dysregulated in MetS, contributing to hypertension. Activation of the RAAS pathway leads to increased production of angiotensin II and aldosterone, which promotes sodium and water retention. These mechanisms further elevate blood pressure levels in individuals with MetS[69,70].
The presence of hypertension in MetS increases considerably the risk of cardiovascular complications, such as cardiopathies, strokes and renal diseases. Therefore, it is essential to effectively control blood pressure in individuals with MetS to minimize these risks. Continuous blood pressure monitoring with noninvasive devices supports healthcare professionals in the assessment and control of blood pressure fluctuations[71]. The most commonly used methods include automatic arm cuff devices, wrist monitors and ambulatory blood pressure monitoring (ABPM). Automated arm cuff devices are frequently used in clinics and health centers. They consist in placing an inflatable cuff around the arm, which is connected to an electronic device. The cuff inflates and deflates automatically, while sensors detect blood pressure oscillations. The devices provide accurate and reliable measurements of systolic and diastolic blood pressure[72-74]. On the other side, wrist blood pressure monitors use an inflatable cuff placed over the radial artery that automatically inflates and deflates to measure blood pressure. Although wrist blood pressure monitors are convenient, it is important to consider that their accuracy can be compromised by body position, movement and individual variations[75-77]. Ultimately, ABPM is a useful tool for assessing blood pressure variations over a 24-hour period. ABPM consists of wearing a portable device that automatically measures blood pressure at regular intervals throughout the day and night. This method provides a complete profile of blood pressure variations during daily activities, sleep, and a variety of conditions, enabling a more accurate assessment of blood pressure control in individuals with MetS[78,79]. Through the periodical monitoring of blood pressure levels, healthcare professionals are able to detect and follow-up changes in blood pressure, determine the effectiveness of lifestyle modifications and medications, and take informed decisions about treatment[71].
3.4 The role of wearables in real-time
In the last decade, wearable devices have become powerful tools in healthcare, enabling real-time monitoring and assessment of various physiological parameters. These devices, which range from fitness trackers to advanced health monitoring wearables, offer several key advantages in assessing MetS[80]. The first advantage is that wearables allow continuous monitoring of essential parameters such as physical activity levels, heart rate, blood pressure, glucose levels and sleep patterns. This real-time data provides a complete and dynamic view of an individual's metabolic health, allowing for a more accurate assessment compared to a one-time measurement[81]. Moving further into MetS treatment, wearables generate personalized data profiles based on each individual's unique physiological responses. This information enables personalized interventions and lifestyle modifications, which are the cornerstone of MetS treatment[82,83]. It is noteworthy that the information obtained with these devices should be analyzed in conjunction with the clinical information of each individual[84]. Nevertheless, in order to widespread and effectively use wearables for MetS assessment, a number of challenges need to be addressed. These include ensuring the accuracy and reliability of the data, by conducting larger studies. Additionally, privacy and security issues must be addressed, and data generated by wearables must be integrated into existing healthcare systems[85,86].
4. Biomedical Engineering Interventions for Managing Metabolic Syndrome
Previously we have discussed how BME contributed to enrich the understanding of the pathophysiology and assessment of MetS. Nevertheless, BME interventions have emerged as promising approaches for the management of MetS. For instance, we have previously discussed that the development of wearable devices equipped with sensors can continuously monitor physiological parameters such as blood glucose levels, heart rate and physical activity. These devices provide real-time data that can aid in personalized management and facilitate timely interventions.
Targeted drug delivery systems have emerged as a promising approach to treat MetS by taking a more comprehensive approach and directly addressing the underlying molecular pathways and mechanisms involved in the development and progression of MetS. Targeted drug delivery systems refer to methods or technologies designed to deliver drugs specifically to the affected tissues or cells while minimizing their exposure to healthy tissues. This approach allows for higher drug concentrations at the target site, which can lead to improved therapeutic outcomes and reduced adverse effect. One of the most widely studied strategies for the targeted delivery of drugs against MetS is nanoparticles[10]. Several types of nanoparticles have been explored for targeted drug delivery in MetS, including liposomes. Liposomes, a unique type of nanodrug delivery system, are spherical vesicles composed of one or more concentric phospholipid bilayers enclosing an aqueous core. There are several advantages of liposomes, such as reduced drug toxicity compared to free drugs and enhancement of immune system of the organism[87]. One of the key advantages of liposomes in the context of MetS is their ability to improve the solubility and stability of drugs. Many drugs used in the treatment of MetS, such as anti-inflammatory drugs or lipid-lowering drugs, often have low water solubility. By encapsulating these drugs within liposomes, their solubility can be enhanced, resulting in improved drug delivery and bioavailability[88-90]. Nevertheless, it has been observed that other types of nanoparticles can also be useful in the treatment of MetS, such as polymeric nanoparticles[91], exosomes[90,92], nanoemulsion[93], and others. Nanoparticles have demonstrated a great potential for targeted drug delivery systems in the treatment of MetS. With the continued advances in nanotechnology, these innovative approaches may revolutionize the treatment of MetS and improve patient outcomes. Continuous research and investment in this field is crucial to unlock the full potential of nanoparticles in the fight against MetS and its associated complications.
5. The Use of Artificial Intelligence and Machine Learning
In recent years, the integration of technological advancements, particularly artificial intelligence (AI) and machine learning (ML), has emerged as a promising approach for understanding and managing MetS[16,94]. AI refers to the use of computers and technology to stimulate intelligent behavior and critical thinking comparable to a human being[95], while ML is an implementation of AI and computer science, employing algorithms that refine accuracy through experiential learning[96]. ML approaches have been noted for their use in MetS risk assessment, where anthropometric, clinical and histological data are used to construct risk models[53,97,98].These models have demonstrated efficient performance in predicting MetS and have facilitated the evaluation of how lifestyle changes, genetics and environmental factors impact such prediction[16,18,99,100].
The future perspectives regarding the use of AI and ML for the assessment and treatment of MetS are immensely promising and herald a transformative era in healthcare. As these technologies move forward, they are primed to transform the way MetS is understood, diagnosed, and treated, offering a multitude of exciting possibilities[101,102]. AI and ML algorithms can be exploited to predict an individual's risk of developing MetS based on a constellation of factors. These predictions enable healthcare professionals to implement targeted preventive measures, allowing individuals to make informed lifestyle changes before the onset of MetS[103,104]. Going beyond MetS prediction, AI-based simulations can accelerate the discovery and development of new pharmaceutical interventions for MetS. By modeling interactions between potential drug candidates and metabolic pathways, AI can identify promising compounds for further exploration. This could lead to the creation of innovative treatments that target specific components of MetS more effectively[105,106].
6. Discussion
Throughout this review we have discussed the role that BME plays in the understanding and assessment of MetS (Figure 2). Starting with the introduction of CBMs in the 1980s and continuing into modern era with the use of AI and ML, BME has evolved along with our understanding of the MetS. Furthermore, progress has been reflected in the introduction of devices that allow continuous assessment of individuals with MetS and have also proven useful in assessing the risk of other MetS-associated diseases. Although BME interventions offer great potential for treating MetS, several challenges need to be addressed to maximize their potential and drive future advances in this field [107]. One of the main challenges is the complexity and heterogeneity of MetS itself. MetS encompasses a wide range of interconnected metabolic abnormalities, each with its own characteristics and underlying mechanisms. The development of interventions that can effectively address multiple aspects of MetS simultaneously remains a major challenge [108]. Another challenge is the translation of BME innovations from the laboratory to clinical practice. While there have been exciting developments in diagnostics and drug delivery systems, many of these advancements are still in the early stages of research or limited to preclinical studies [109]. In addition, the widespread implementation of BME solutions faces barriers related to accessibility and cost. Many advanced diagnostic tools, portable devices and computational models require sophisticated technology and infrastructure, which may not be available in all healthcare settings, especially in resource-constrained regions [110]. Finally, as BME continues to evolve, interdisciplinary collaboration will be critical. Researchers, clinicians, engineers, and data scientists must work together to refine existing technologies and develop new solutions that address the multifaceted nature of MS. Long-term clinical studies are needed to evaluate the efficacy and safety of BME interventions in diverse populations, ensuring that these technologies lead to sustainable improvements in patient outcomes [111]. By addressing the challenges and focusing on personalized approaches, targeted therapies, digital health integration, collaboration, and long-term assessment, BME can pave the way for transformative interventions that enhance the lives of individuals affected by MetS.
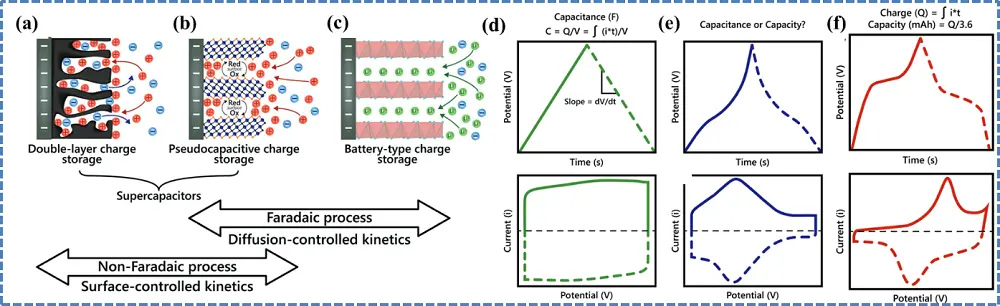
Figure 2. Biomedical engineering approaches addressing MetS. MetS: metabolic syndrome; BCAs: body composition analyzers; CGM: continuous glucose monitoring.
7. Conclusions
In conclusion, the integration of BME has significantly advanced our understanding of MetS by providing new tools and approaches for both diagnosis and treatment. Nevertheless, challenges remain, such as the complexity of MetS and the need to translate laboratory innovations into clinical practice. Continued research and collaboration will be essential to fully harness the potential of BME in improving diagnosis, treatment, and long-term outcomes for people affected by MetS.
Authors contribution
Ramírez-Mejía MM: Investigation, visualization, writing-original draft, review & editing.
Méndez-Sanchez N: Conceptualization, supervision, writing-original draft, review & editing.
All authors approved the final version of the manuscript.
Conflict of interest
The authors declare no conflict of interest.
Ethical approval
Not applicable.
Consent to participate
Not applicable.
Consent for publication
Not applicable.
Availability of data and materials
Not applicable
Funding information
None.
Copyright
© The Author(s) 2023.
References
-
1. Samson SL, Garber AJ. Metabolic syndrome. Endocrinol Metab Clin North Am. 2014;43(1):1-23.
[DOI] -
2. Mottillo S, Filion KB, Genest J, Joseph L, Pilote L, Poirier P, et al. The metabolic syndrome and cardiovascular risk a systematic review and meta-analysis. J Am Coll Cardiol. 2010;56(14):1113-1132.
[DOI] -
3. Silveira Rossi JL, Barbalho SM, de Araujo RR, Bechara MD, Sloan KP, Sloan LA. Metabolic syndrome and cardiovascular diseases: Going beyond traditional risk factors. Diabetes Metab Res Rev. 2022;38(3):e3502.
[DOI] -
4. James M, Varghese TP, Sharma R, Chand S. Association between metabolic syndrome and diabetes mellitus according to international diabetic federation and national cholesterol education program adult treatment panel III criteria: a cross-sectional study. J Diabetes Metab Disord. 2020;19(1):437-443.
[DOI] -
5. Battelli MG, Bortolotti M, Polito L, Bolognesi A. Metabolic syndrome and cancer risk: the role of xanthine oxidoreductase. Redox Biol. 2019;21:101070.
[DOI] -
6. Yu Y, Gong L, Ye J. The role of aberrant metabolism in cancer: insights into the interplay between cell metabolic reprogramming, metabolic syndrome, and cancer. Front Oncol. 2020;10:942.
[DOI] -
7. Huang PL. A comprehensive definition for metabolic syndrome. Dis Model Mech. 2009;2(5-6):231-237.
[DOI] -
8. Saklayen MG. The global epidemic of the metabolic syndrome. Curr Hypertens Rep. 2018;20(2):12.
[DOI] -
9. Kao TW, Huang CC. Recent progress in metabolic syndrome research and therapeutics. Int J Mol Sci. 2021;22(13):6862.
[DOI] -
10. Bahadori E, Farjami Z, Rezayi M, Lngari H, Darroudi M, Avan A, et al. Recent advances in nanotechnology for the treatment of metabolic syndrome. Diabetes Metab Syndr. 2019;13(2):1561-1568.
[DOI] -
11. Yu Q, Huang S, Xu TT, Wang YC, Ju S. Measuring brown fat using MRI and implications in the metabolic syndrome. J Magn Reson Imaging. 2021;54(5):1377-1392.
[DOI] -
12. Ambroselli D, Masciulli F, Romano E, Catanzaro G, Besharat ZM, Massari MC, et al. New advances in metabolic syndrome, from prevention to treatment: the role of diet and food. Nutrients. 2023;15(3):640.
[DOI] -
13. Aurich MK, Thiele I. Computational modeling of human metabolism and its application to systems biomedicine. Methods Mol Biol. 2016;1386:253-281.
[DOI] -
14. Lusis AJ, Attie AD, Reue K. Metabolic syndrome: from epidemiology to systems biology. Nat Rev Genet. 2008;9(11):819-830.
[DOI] -
15. Ismail SNA, Nayan NA, Jaafar R, May Z. Recent advances in non-invasive blood pressure monitoring and prediction using a machine learning approach. Sensors. 2022;22(16):6195.
[DOI] -
16. Hsu NW, Chou KC, Wang YT, Hung CL, Kuo CF, Tsai SY. Building a model for predicting metabolic syndrome using artificial intelligence based on an investigation of whole-genome sequencing. J Transl Med. 2022;20(1):190.
[DOI] -
17. Rozendaal YJW, Wang Y, Hilbers PAJ, van Riel NAW. Computational modelling of energy balance in individuals with metabolic syndrome. BMC Syst Biol. 2019;13(1):24.
[DOI] -
18. Benmohammed K, Valensi P, Omri N, Al Masry, Zerhouni N. Metabolic syndrome screening in adolescents: new scores AI_METS based on artificial intelligence techniques. Nutr Metab Cardiovasc Dis. 2022;32(12):2890-2899.
[DOI] -
19. Chen D, Zhao X, Sui Z, Niu H, Chen L, Hu C, et al. A multi-omics investigation of the molecular characteristics and classification of six metabolic syndrome relevant diseases. Theranostics. 2020;10(5):2029-2046.
[DOI] -
20. Wu Q, Li J, Sun X, He D, Cheng Z, Li J, et al. Multi-stage metabolomics and genetic analyses identified metabolite biomarkers of metabolic syndrome and their genetic determinants. eBioMedicine. 2021;74:103707.
[DOI] -
21. Mott R, FabbianoS S, Levinson R, Emambokus N. Bioengineering and Metabolism Voices. Cell Metab. 2019;29(3):506-512.
[DOI] -
22. Volkova S, Matos MRA, Mattanovich M, de Mas IM. Metabolic modelling as a framework for metabolomics data integration and analysis. Metabolites. 2020;10(8):303.
[DOI] -
23. Bordbar A, Monk JM, King ZA, Palsson BO. Constraint-based models predict metabolic and associated cellular functions. Nat Rev Genet. 2014;15(2):107-120.
[DOI] -
24. Fell DA, Small JR. Fat synthesis in adipose tissue. An examination of stoichiometric constraints. Biochem J. 1986;238(3):781-786.
[DOI] -
25. Lewis NE, Nagarajan H, Palsson BO. Constraining the metabolic genotype-phenotype relationship using a phylogeny of in silico methods. Nat Rev Microbiol. 2012;10(4):291-305.
[DOI] -
26. Dandekar T, Schuster S, Snel B, Huynen M, Bork P. Pathway alignment: application to the comparative analysis of glycolytic enzymes. Biochem J. 1999;343:115-124.
[PubMed] -
27. Moulin C, Tournier L, Peres S. Combining kinetic and constraint-based modelling to better understand metabolism dynamics. Processes. 2021;9(10):1701.
[DOI] -
28. Stelling J, Klamt S, Bettenbrock K, Schuster S, Gilles ED. Metabolic network structure determines key aspects of functionality and regulation. Nature. 2002;420(6912):190-193.
[DOI] -
29. Almaas E, Kovács B, Vicsek T, Oltvai ZN, Barabási AL. Global organization of metabolic fluxes in the bacterium Escherichia coli. Nature. 2004;427(6977):839-843.
[DOI] -
30. Yu H, Blair RH. Integration of probabilistic regulatory networks into constraint-based models of metabolism with applications to Alzheimer's disease. BMC Bioinformatics. 2019;20(1):386.
[DOI] -
31. Meeson KE, Schwartz JM. Constraint-based modeling predicts metabolic signatures of low and high-grade serous ovarian cancer. npj Syst Biol Appl. 2024;96:10.
[DOI] -
32. Mao L, Nicolae A, Oliveira MAP, He F, Hachi S, Fleming RMT. A constraint-based modelling approach to metabolic dysfunction in Parkinson's disease. Comput Struct Biotechnol J. 2015;13:484-491.
[DOI] -
33. Strutz J, Martin J, Greene J, Broadbelt L, Tyo K. Metabolic kinetic modeling provides insight into complex biological questions, but hurdles remain. Curr Opin Biotechnol. 2019;59:24-30.
[DOI] -
34. Islam MM, Schroeder WL, Saha R. Kinetic modeling of metabolism: present and future. Curr Opin Syst Biol. 2021;26:72-78.
[DOI] -
35. Saa PA, Nielsen LK. Formulation, construction and analysis of kinetic models of metabolism: a review of modelling frameworks. Biotechnol Adv. 2017;35(8):981-1003.
[DOI] -
36. Lu H, Chen Y, Nielsen J, Kerkhoven EJ. Kinetic Models of Metabolism. In: Nielsen J, Stephanopoulos G, Lee SY, editors. Metabolic Engineering: Concepts and Applications. Weinheim: Wiley-VCH; 2021. p. 153-170.
[DOI] -
37. Rozendaal YJW, Wang Y, Paalvast Y, Tambyrajah LL, Li Z, van Dijk KW, et al. In vivo and in silico dynamics of the development of metabolic syndrome. PLoS Comput Biol. 2018;14(6):e1006145.
[DOI] -
38. Paalvast Y, Zhou E, Rozendaal YJW, Wang Y, Gerding A, van Dijk TH, et al. A systems analysis of phenotype heterogeneity in APOE*3Leiden.CETP mice induced by long-term high-fat high-cholesterol diet feeding. Nutrients. 2022;14(22):4936.
[DOI] -
39. Del Giacco L, Cattaneo C. Introduction to genomics. In: Espina V, Liotta L, editors. Molecular Profiling. Totowa: Humana Press; 2011. p. 79-88.
[DOI] -
40. Williams CG, Lee HJ, Asatsuma T, Vento-Tormo R, Haque A. An introduction to spatial transcriptomics for biomedical research. Genome Med. 2022;14(1):68.
[DOI] -
41. Al-Amrani S, Al-Jabri Z, Al-Zaabi A, Alshekaili J, Al-Khabori M. Proteomics: concepts and applications in human medicine. World J Biol Chem. 2021;12(5):57-69.
[DOI] -
42. Clish CB. Metabolomics: an emerging but powerful tool for precision medicine. Cold Spring Harb Mol Case Stud. 2015;1(1):a000588.
[DOI] -
43. Wang KC, Chang HY. Epigenomics: technologies and applications. Circ Res. 2018;122(9):1191-1199.
[DOI] -
44. Hu C, Jia W. Multi-omics profiling: the way towards precision medicine in metabolic diseases. J Mol Cell Biol. 2021;13(8):576-593.
[DOI] -
45. Taylor JY, Kraja AT, de Las Fuentes L, Stanfill AG, Clark A, Cashion A. An overview of the genomics of metabolic syndrome. J Nurs Scholarsh. 2013;45(1):52-59.
[DOI] -
46. Mir FA, Mall R, Ullah E, Iskandarani A, Cyprian F, Samra TA, et al. An integrated multi-omic approach demonstrates distinct molecular signatures between human obesity with and without metabolic complications: a case-control study. J Transl Med. 2023;21(1):229.
[DOI] -
47. Ozhan H, Alemdar R, Caglar O, Ordu S, Kaya A, Albayrak S, et al. Performance of bioelectrical impedance analysis in the diagnosis of metabolic syndrome. J Investig Med. 2012;60(3):587-591.
[DOI] -
48. Stramaglia G, Greco A, Guglielmi G, De Matthaeis A, Vendemiale GL. Echocardiography and dual-energy x-ray absorptiometry in the elderly patients with metabolic syndrome: a comparison of two different tecniques to evaluate visceral fat distribution. J Nutr Health Aging. 2010;14(1):6-10.
[DOI] -
49. Buscemi S, Verga S, Cottone S, Azzolina V, Buscemi B, Gioia D, et al. Glycaemic variability and inflammation in subjects with metabolic syndrome. Acta Diabetol. 2009;46(1):55-61.
[DOI] -
50. Madhurantakam S, Babu KJ, Rayappan JBB, Krishnan UM. Nanotechnology-based electrochemical detection strategies for hypertension markers. Biosens Bioelectron. 2018;116:67-80.
[DOI] -
51. Bentes CM, da Silveira ALB, Di Masi F, Resende M, Netto C, Marinheiro LPF. Reliability of bioimpedance and indirect calorimetry to evaluate resting metabolic rate in Brazilian women with metabolic syndrome. Diabetes Metab Syndr. 2021;15(2):493-497.
[DOI] -
52. Pi-Sunyer X. Changes in body composition and metabolic disease risk. Eur J Clin Nutr. 2019;73(2):231-235.
[DOI] -
53. Lee YC, Lee YH, Chuang PN, Kuo CS, Lu CW, Yang KC. The utility of visceral fat level measured by bioelectrical impedance analysis in predicting metabolic syndrome. Obes Res Clin Pract. 2020;14(6):519-523.
[DOI] -
54. Mulasi U, Kuchnia AJ, Cole AJ, Earthman CP. Bioimpedance at the bedside: current applications, limitations, and opportunities. Nutr Clin Pract. 2015;30(2):180-193.
[DOI] -
55. Jaffrin MY, Morel H. Body fluid volumes measurements by impedance: a review of bioimpedance spectroscopy (BIS) and bioimpedance analysis (BIA) methods. Med Eng Phys. 2008;30(10):1257-1269.
[DOI] -
56. Pouragha H, Amiri M, Saraei M, Pouryaghoub G, Mehrdad R. Body impedance analyzer and anthropometric indicators; predictors of metabolic syndrome. J Diabetes Metab Disord. 2021;20(2):1169-1178.
[DOI] -
57. Kim SH, Kang HW, Jeong JB, Lee DS, Ahn DW, Kim JW, et al. Association of obesity, visceral adiposity, and sarcopenia with an increased risk of metabolic syndrome: a retrospective study. PLoS One. 2021;16(8):e0256083.
[DOI] -
58. Wong SK, Chin KY, Suhaimi FH, Ahmad F, Ima-Nirwana S. The relationship between metabolic syndrome and osteoporosis: a review. Nutrients. 2016;8(6):347.
[DOI] -
59. Xue P, Gao P, Li Y. The association between metabolic syndrome and bone mineral density: a meta-analysis. Endocrine. 2012;42(3):546-554.
[DOI] -
60. Kaul S, Rothney MP, Peters DM, Wacker WK, Davis CE, Shapiro MD, et al. Dual-energy X-ray absorptiometry for quantification of visceral fat. Obesity. 2012;20(6):1313-1318.
[DOI] -
61. Goh VH, Hart WG. Association of general and abdominal obesity with age, endocrine and metabolic factors in Asian men. Aging Male. 2016;19(1):27-33.
[DOI] -
62. Mesinovic J, McMillan LB, Shore-Lorenti C, De Courten B, Ebeling PR, Scott D. Metabolic syndrome and its associations with components of sarcopenia in overweight and obese older adults. J Clin Med. 2019;8(2):145.
[DOI] -
63. Fahed G, Aoun L, Zerdan MB, Allam S, Zerdan MB, Bouferraa Y, et al. Metabolic syndrome: updates on pathophysiology and management in 2021. Int J Mol Sci. 2022;23(2):786.
[DOI] -
64. Mendrick DL, Diehl AM, Topor LS, Dietert RR, Will Y, La Merrill, et al. Metabolic syndrome and associated diseases: from the bench to the clinic. Toxicol Sci. 2018;162(1):36-42.
[DOI] -
65. Bovolini A, Garcia J, Andrade MA, Duarte JA. Metabolic syndrome pathophysiology and predisposing factors. Int J Sports Med. 2021;42(3):199-214.
[DOI] -
66. Holzer R, Bloch W, Brinkmann C. Continuous glucose monitoring in healthy adults-possible applications in health care, wellness, and sports. Sensors. 2022;22(5):2030.
[DOI] -
67. Krakauer M, Botero JF, Lavalle-González FJ, Proietti A, Barbieri DE. A review of flash glucose monitoring in type 2 diabetes. Diabetol Metab Syndr. 2021;13(1):42.
[DOI] -
68. Zhou MS, Wang A, Yu H. Link between insulin resistance and hypertension: what is the evidence from evolutionary biology? Diabetol Metab Syndr. 2014;6(1):12.
[DOI] -
69. Kishi T, Hirooka Y. Sympathoexcitation associated with renin-angiotensin system in metabolic syndrome. Int J Hypertens. 2013;2013:406897.
[DOI] -
70. Stanciu S, Rusu E, Miricescu D, Radu AC, Axinia B, Vrabie AM, et al. Links between metabolic syndrome and hypertension: the relationship with the current antidiabetic drugs. Metabolites. 2023;13(1):87.
[DOI] -
71. Meidert AS, Saugel B. Techniques for non-invasive monitoring of arterial blood pressure. Front Med. 2018;4:231.
[DOI] -
72. Chen Y, Lei L, Wang JG. Methods of blood pressure assessment used in milestone hypertension tials. Pulse. 2018;6(1-2):112-123.
[DOI] -
73. Huang JF, Li Y, Shin J, Chia YC, Sukonthasarn A, Turana Y, et al. Characteristics and control of the 24-hour ambulatory blood pressure in patients with metabolic syndrome. J Clin Hypertens. 2021;23(3):450-456.
[DOI] -
74. Ukkola O, Vasunta RL, Kesäniemi YA. Non-dipping pattern in ambulatory blood pressure monitoring is associated with metabolic abnormalities in a random sample of middle-aged subjects. Hypertens Res. 2009;32(11):1022-1027.
[DOI] -
75. Casiglia E, Tikhonoff V, Albertini F, Palatini P. Poor reliability of wrist blood pressure self-measurement at home: a population-based study. Hypertension. 2016;68(4):896-903.
[DOI] -
76. Hoffmann U, Drey M, Thrun JM, Obermeier E, Weingart C, Hafner K, et al. The role of wrist monitors to measure blood pressure in older adults. Aging Clin Exp Res. 2019;31(9):1227-1231.
[DOI] -
77. Sayer G, Piper G, Vorovich E, Raikhelkar J, Kim GH, Rodgers D, et al. Continuous monitoring of blood pressure using a wrist-worn cuffless device. Am J Hypertens. 2022;35(5):407-413.
[DOI] -
78. O'Brien E, White WB, Parati G, Dolan E. Ambulatory blood pressure monitoring in the 21st century. J Clin Hypertens. 2018;20(7):1108-1111.
[DOI] -
79. Mengden T, Weisser B. Monitoring of treatment for arterial hypertension-the role of office, home, and 24 h ambulatory blood pressure measurement. Dtsch Arztebl Int. 2021;118(27-28):473-478.
[DOI] -
80. Yetisen AK, Martinez-Hurtado JL, Ünal B, Khademhosseini A, Butt H. Wearables in medicine. Adv Mater. 2018;30(33):e1706910.
[DOI] -
81. Keshet A, Reicher L, Bar N, Segal E. Wearable and digital devices to monitor and treat metabolic diseases. Nat Metab. 2023;5(4):563-571.
[DOI] -
82. Huh U, Tak YJ, Song S, Chung SW, Sung SM, Lee CW, et al. Feedback on physical activity through a wearable device connected to a mobile phone app in patients with metabolic syndrome: pilot study. JMIR Mhealth Uhealth. 2019;7(6):e13381.
[DOI] -
83. Yamaga Y, Svensson T, Chung UI, Svensson AK. Association between metabolic syndrome status and daily physical activity measured by a wearable device in japanese office workers. Int J Environ Res Public Health. 2023;20(5):4315.
[DOI] -
84. Reeder B, David A. Health at hand: a systematic review of smart watch uses for health and wellness. J Biomed Inform. 2016;63:269-276.
[DOI] -
85. Fagherazzi G, Ravaud P. Digital diabetes: perspectives for diabetes prevention, management and research. Diabetes Metab. 2019;45(4):322-329.
[DOI] -
86. Canali S, Schiaffonati V, Aliverti A. Challenges and recommendations for wearable devices in digital health: data quality, interoperability, health equity, fairness. PLoS Digit Health. 2022;1(10):e0000104.
[DOI] -
87. Guimarães D, Cavaco-Paulo A, Nogueira E. Design of liposomes as drug delivery system for therapeutic applications. Int J Pharm. 2021;601:120571.
[DOI] -
88. Chen Q, Guo C, Liu Z, Cao M, Wang W, Zhang D, et al. Multifunctional nanoparticles with anti-inflammatory effect for improving metabolic syndromes. J Drug Target. 2023;31(3):286-295.
[DOI] -
89. Hu F, Sun DS, Wang KL, Shang DY. Nanomedicine of plant origin for the treatment of metabolic disorders. Front Bioeng Biotechnol. 2022;9:811917.
[DOI] -
90. Li T, Zhu L, Zhu L, Wang P, Xu W, Huang J. Recent developments in delivery of microRNAs utilizing nanosystems for metabolic syndrome therapy. Int J Mol Sci. 2021;22(15):7855.
[DOI] -
91. Şaman E, Cebova M, Barta A, Koneracka M, Zavisova V, Eckstein-Andicsova A, et al. Combined therapy with simvastatin- and coenzyme-Q10-loaded nanoparticles upregulates the Akt-eNOS pathway in experimental metabolic syndrome. Int J Mol Sci. 2022;24(1):276.
[DOI] -
92. Beuzelin D, Kaeffer B. Exosomes and miRNA-loaded biomimetic nanovehicles, a focus on their potentials preventing type-2 diabetes linked to metabolic syndrome. Front Immunol. 2018;9:2711.
[DOI] -
93. El-Say KM, Felimban RI, Tayeb HH, Chaudhary AG, Omar AM, Rizg WY, et al. Pairing 3D-printing with nanotechnology to manage metabolic syndrome. Int J Nanomedicine. 2022;17:1783-1801.
[DOI] -
94. Sghaireen MG, Al-Smadi Y, Al-Qerem A, Srivastava KC, Ganji KK, Alam MK, et al. Machine learning approach for metabolic syndrome diagnosis using explainable data-augmentation-based classification. Diagnostics. 2022;12(12):3117.
[DOI] -
95. Amisha F, Malik P, Pathania M, Rathaur VK. Overview of artificial intelligence in medicine. J Family Med Prim Care. 2019;8(7):2328-2331.
[DOI] -
96. Obermeyer Z, Emanuel EJ. Predicting the future-big data, machine learning, and clinical medicine. N Engl J Med. 2016;375(13):1216-1219.
[DOI] -
97. Karimi-Alavijeh F, Jalili S, Sadeghi M. Predicting metabolic syndrome using decision tree and support vector machine methods. ARYA Atheroscler. 2016;12(3):146-152.
[PubMed] -
98. Olveres J, González G, Torres F, Moreno-Tagle JC, Carbajal-Degante E, Valencia-Rodríguez A, et al. What is new in computer vision and artificial intelligence in medical image analysis applications. Quant Imaging Med Surg. 2021;11(8):3830-3853.
[DOI] -
99. Tavares LD, Manoel A, Donato THR, Cesena F, Minanni CA, Kashiwagi NM, et al. Prediction of metabolic syndrome: a machine learning approach to help primary prevention. Diabetes Res Clin Pract. 2022;191:110047.
[DOI] -
100. Safaei M, Sundararajan EA, Driss M, Boulila W, Shapi'i A. A systematic literature review on obesity: understanding the causes & consequences of obesity and reviewing various machine learning approaches used to predict obesity. Comput Biol Med. 2021;136:104754.
[DOI] -
101. Javaid A, Zghyer F, Kim C, Spaulding EM, Isakadze N, Ding J, et al. Medicine 2032: The future of cardiovascular disease prevention with machine learning and digital health technology. Am J Prev Cardiol. 2022;12:100379.
[DOI] -
102. Ansari RM, Harris MF, Hosseinzadeh H, Zwar N. Application of artificial intelligence in assessing the self-management practices of patients with type 2 diabetes. Healthcare. 2023;11(6):903.
[DOI] -
103. Lee S, Lee SK, Kim JY, Cho N, Shin C. Sasang constitutional types for the risk prediction of metabolic syndrome: a 14-year longitudinal prospective cohort study. BMC Complement Altern Med. 2017;17(1):438.
[DOI] -
104. Li G, Esangbedo IC, Xu L, Fu J, Li L, Feng D, et al. Childhood retinol-binding protein 4 (RBP4) levels predicting the 10-year risk of insulin resistance and metabolic syndrome: the BCAMS study. Cardiovasc Diabetol. 2018;17(1):69.
[DOI] -
105. Khotimchenko M, Brunk NE, Hixon MS, Walden DM, Hou H, Chakravarty K, et al. Combinatorial approaches using an AI/ML-driven drug development platform targeting insulin resistance, lipid dysregulation, and inflammation for the amelioration of metabolic syndrome in patients. BioRxiv [Preprint]. 2021.
[DOI] -
106. Jiang X, Yang Z, Wang S, Deng S. "Big Data" approaches for prevention of the metabolic syndrome. Front Genet. 2022;13:810152.
[DOI] -
107. Singh S, Sarma DK, Verma V, Nagpal R, Kumar M. Unveiling the future of metabolic medicine: omics technologies driving personalized solutions for precision treatment of metabolic disorders. Biochem Biophys Res Commun. 2023;682(2):1-20.
[DOI] -
108. Jha BK, Sherpa ML, Imran M, Mohammed Y, Jha LA, Paudel KR, et al. Progress in Understanding Metabolic Syndrome and Knowledge of Its Complex Pathophysiology. Diabetology. 2023;4(2):134-159.
[DOI] -
109. Euliano EM, Sklavounos AA, Wheeler AR, McHugh KJ. Translating diagnostics and drug delivery technologies to low-resource settings. Sci Transl Med. 2022;14(666):eabm1732.
[DOI] -
110. Lustick DR, Zaman MH. Biomedical engineering education and practice challenges and opportunities in improving health in developing countries. In: Proceedings of the 2011 Atlanta Conference on Science and Innovation Policy; 2011 Sep 15-17; Atlanta, USA. New York: IEEE; 2011. p. 15-17.
[DOI] -
111. Varnosfaderani SM, Forouzanfar M. The Role of AI in Hospitals and Clinics: Transforming Healthcare in the 21st Century. Bioengineering. 2024;11(4):337.
[DOI]
Copyright
© The Author(s) 2023. This is an Open Access article licensed under a Creative Commons Attribution 4.0 International License (https://creativecommons.org/licenses/by/4.0/), which permits unrestricted use, sharing, adaptation, distribution and reproduction in any medium or format, for any purpose, even commercially, as long as you give appropriate credit to the original author(s) and the source, provide a link to the Creative Commons license, and indicate if changes were made.
Publisher’s Note
Share And Cite