Abstract
Digital twin (DT) technology is revolutionizing the architecture, engineering, construction, and operation (AECO) industry, driven by the advancements of Construction 4.0 (C4.0). Despite this, adopting DT in the AECO sector remains limited due to various barriers. This study, driven by four research questions (RQ), aims to advance DT adoption in the AECO industry under C4.0 using an integrated approach. The degrees of influence and importance ranking of barriers are assessed through the decision-making trial and evaluation laboratory (DEMATEL). The hierarchy and causal relationships among barriers are revealed through interpretative structural modeling (ISM). Then, barriers are divided into four clusters employing the cross-impact matrix multiplication applied to classification (MICMAC) method. The paper reveals that "lack of government financial and policy support" is the most critical barrier, "lack of trust and long-term perspective in DT" is the most significant direct-influence barrier, and "immature 3D engine technology" is the most fundamental barrier. By exploring interrelationships and prioritizing barriers, the study provides insights to enhance adopting DT in the AECO industry in the context of C4.0.
Keywords
1. Introduction
The progression of Construction 4.0 (C4.0) has given rise to a proliferation of digital technologies, among which digital twin (DT) stands out as the most cutting-edge advancements. C4.0 refers to the integration of advanced technologies such as automation and data analytics into construction processes to improve efficiency and sustainability[1], and DT is a key component of C4.0, enabling real-time virtual representations of physical assets. This paper analyzes the barriers to DT adoption within the context of C4.0, which is well-established and widely adopted, aiming to provide a comprehensive understanding of the current challenges in the DT adoption process. C4.0 can enhance cooperation among stakeholders by facilitating digital platforms that integrate the virtual versions of physical entities[2]. DT denotes a digital replica of systems, real entities, or procedures, utilizing immediate data from sensors or Internet of Things (IoT) devices to track and measure the immediate condition of tangible assets[3,4].
DT necessitates proficiency in diverse areas, including building information modeling (BIM), software engineering, and data science[5]. The DT paradigm comprises physical and digital entities and an accurate digital replica, connecting through a data connection[6]. DT can transfer the information of physical assets into data used for developing virtual models[7]. Integrating DT into C4.0 revolutionizes the interaction between tangible and digital environments, enabling instantaneous tracking and regulation of physical systems via virtual representations.
DT has been applied over diverse industries, encompassing manufacturing, aerospace, production, and operations management. In many developing countries, the architecture, engineering, construction, and operation (AECO) sector is less digitized compared with other sectors[8-10]. However, as an industry highly reliant on dynamic information, the AECO sector requires data to be accurate, accessible, and understandable for improved energy and carbon control[11,12], lifecycle monitoring of buildings[13-15], and waste management[16]. Due to the rising demand for DT and its inherent digitalization impact on the AECO sector, DT presents substantial prospects.
Although DT can be utilized throughout a building's entire lifecycle, many of its applications remain superficial and theoretical. To better promote the practical application of DT in the AECO sector, it is essential to reveal and analyze DT barriers. The paper's novelty is providing a thorough analysis of barriers to DT adoption in the AECO industry, utilizing an integrated approach of decision-making trial and evaluation laboratory (DEMATEL), interpretative structural modeling (ISM), and cross-impact matrix multiplication applied to classification (MICMAC). The paper adopts the DEMATEL method to quantify the barriers and their interrelationships across three dimensions, employs the ISM approach to reveal hierarchical relationships and correlation paths, and utilizes MICMAC analysis to divide the barriers into four clusters according to the varying degrees of driving and dependency power. The study's findings offer critical insights and actionable recommendations, pioneering a framework to overcome industry-specific challenges and facilitate DT adoption. The research questions (RQ) listed below directed this study:
RQ1: What are the barriers to adopting DT within the AECO industry?
RQ2: Which barriers are the most important and should be prioritized to solve?
RQ3: How do the barriers interact and influence each other?
RQ4: How to address the barriers to promoting DT in the context of C4.0?
2. Literature Review
2.1 Applications of DT in the era of C4.0
The promotion of Industry 4.0 has significantly increased the popularity and utilization of DT in the AECO industry. C4.0 requires the construction industry to evolve towards digitalization and automation in production processes[17]. As the AECO industry increasingly prioritizes efficiency and competitiveness, the utilization of DT has become more prevalent[18], with its application being extensively studied over construction projects' life cycle.
DT has the capability to identify potential issues, optimize designs, simulate construction processes, and monitor building performance throughout their lifecycle[3]. Additionally, DT can evaluate various construction methods and forecast their impact on structural performance, energy usage, and sustainability[19]. Employing DT on construction sites can enhance workers' productivity, safety, and health levels, while optimizing efficiency through the monitoring and diagnosis of asset conditions[20].
Existing research has also stressed the importance of adopting DT in the AECO sector. Boje et al.[21] emphasized the importance of an intelligent DT with comprehensive, scalable semantics and highlighted the need to understand the physical world to achieve smart and efficient construction practices. Pan and Zhang[22] integrated data mining, IoT, and BIM to model worker collaboration and working processes, subsequently optimizing schedules via adjusting task assignments and staffing based on evolving site conditions. Wang et al.[23] generated a DT system through virtual reality facilitating collaborative human-robot construction work, integrating visualization, task planning, execution, and communication, validated through a drywall installation case study. DT technology in the AECO sector demonstrates significant potential by enhancing design, construction processes, and maintenance through advanced data integration, predictive modeling, and real-time monitoring.
2.2 Relevant research in analyzing barriers to DT adoption
There is a growing requirement to examine barriers to adopting DT to overcome the complexities of integrating this transformative technology into various industries. Mohandes[20] analyzed the importance of barriers impeding DT implementation using the methodologies of Ginni's coefficient of mean difference, mean ratings, and ranking analysis. Saporiti et al.[24] utilized a Delphi method involving experts from both academia and industry to map the key challenges and propose countermeasures for implementing DT in manufacturing. Weil et al.[25] carried out a literature review to determine and categorize key challenges in implementing DT, highlighting issues such as interoperability, data quality, and governance. Deepu and Ravi[26] utilized a Grey-based DEMATEL to model key elements for adopting DT and physical internet, finding top management commitment and comprehensive planning as the factors with the highest impactability.
While there is a pressing demand for analyzing barriers to DT applications, there is limited analysis of such barriers within the AECO industry. Moreover, the methodologies adopted in previous studies are not comprehensive, often concentrating on singular aspects such as the significance of barriers. The lack of integrated causal relationships and hierarchical structures hinders a clear and systematic understanding of interdependencies among barriers in complex systems. Therefore, this paper aims to advance adopting DT in the AECO industry by analyzing the interrelationship, importance, and influential ability of barriers.
3. Methodology
To achieve the proposed research aim, an integrated approach including DEMATEL, ISM, and MICMAC methods is adopted. Firstly, a systematic review was conducted to identify DT barriers, followed by expert interviews to validate these barriers. After that, data is collected by distributing questionnaires to experts in the relevant field. The DT adopted in this paper refers to the digital twin technology implemented under the framework of C4.0, encompassing both mechanical and management applications in new and existing buildings, including residential and infrastructure projects. The nine survey respondents all have a background in DT implementation within the AECO industry and possess extensive knowledge of DT. The experts' information is shown in Table S1. The data is subsequently utilized for barrier analysis through the DEMATEL, ISM, and MICMAC analysis. The research workflow is demonstrated in Figure 1.
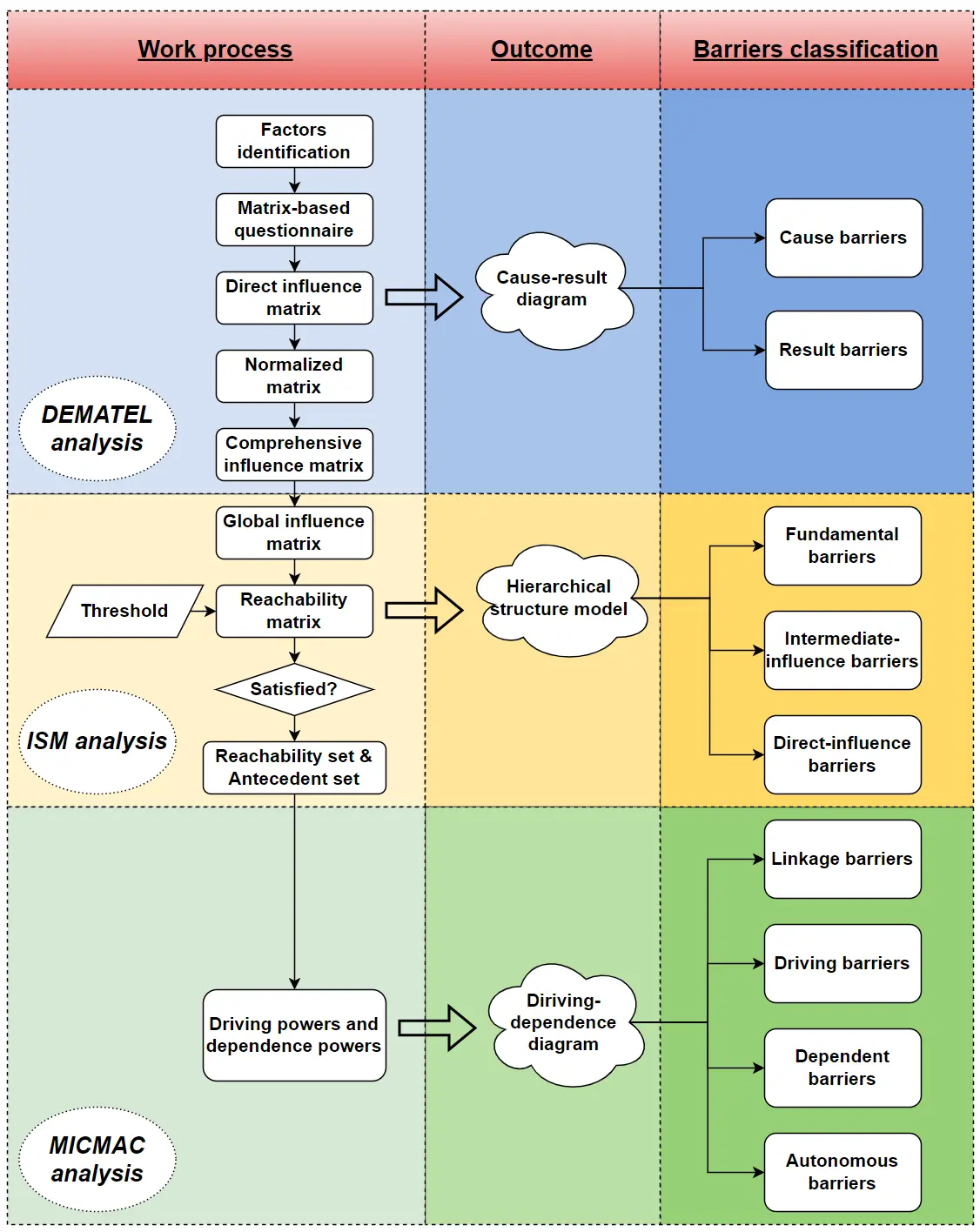
Figure 1. Framework of the research process. DEMATEL: decision-making trial and evaluation laboratory; ISM: interpretative structural modeling; MICMAC: cross-impact matrix multiplication applied to classification.
The overall context of the paper is C4.0, which aims to transform traditional construction processes through digitalization and automation. Some well-known technologies adopted in the AECO industry are part of C4.0, such as DT, big data, the Internet of Things, and remote sensing[27]. Among these, DT attracts the most attention as it encompasses a wide range of other digital technologies. During the semi-structured interviews and surveys, the experts and respondents were informed about the full context of C4.0. Both the discussion and the Likert-scale survey reflected the overall background of C4.0.
3.1 Barriers identification
The technology-organization-environment framework is adopted in this paper for barrier identification from three perspectives: technology, organization, and environment. This framework is suitable for analyzing barriers that impact adopting emerging technologies[28,29]. After identifying barriers through a systematic literature review, expert interviews were conducted to validate and provide feedback on the identified barriers[30]. A key characteristic of AECO projects is their custom, non-standardized nature. In contrast to manufacturing sectors like aviation and automotive, which benefit from mass production and standardization, AECO projects are typically tailored to specific needs, with each project having its own unique set of requirements. Consequently, technologies like DT, which depend on standardized data and processes, encounter considerable challenges in the AECO industry[31]. The slower adoption of DT in AECO, compared to manufacturing sectors, is largely due to the industry's inherent complexity, the project-specific nature of construction, and the absence of standardized, repeatable processes that are common in other industries. The barriers were subsequently revised based on the experts' comments. All the barriers identified and analyzed are summarized in Table 1.
Category | Number | Barrier | References |
Environment | X1 | Lack of industrial guidelines and data standards | [19,32-41] |
X2 | Lack of government financial and policy support | [35,36,42-45] | |
X3 | Lack of experts in the DT field | [4,19,35,36,38,41,43,45-47] | |
X4 | Unclear understanding and requirements of DT | [34,39,48] | |
Organization | X5 | Lack of organizational strategies and leadership on DT | [19,32,35,36,41,45,49,50] |
X6 | Lack of trust and long-term perspective in DT | [4,19,34,36,37,41,43,49] | |
X7 | High investment with an uncertain rate of return | [4,19,33,35,37,41-43,47,50-52] | |
X8 | Slow culture change (Existing processes and institutions do not apply to DT) | [19,35,36,39,41,42,45,47,49,50] | |
Technology | X9 | High requirements on data itself and data management | [4,19,32-35,37-43,45,47,49,51,53-58] |
X10 | Immature application scenarios of DT | Experts input | |
X11 | Immature 3D engine technology | Experts input; [59,60] | |
X12 | Mismatching of IoT devices and DT | Experts input; [61] | |
X13 | High accuracy and validation requirement of DT models | [38,40,56] | |
X14 | Hard to realize real-time and bidirectional communication between virtual and physical objects | [4,38,56,61] | |
X15 | Difficulty in compatibility and interoperability of different technologies and models | [4,19,37,38,43,47,51,56] | |
X16 | Insufficient adoption of (information technology) IT infrastructure | [34,41,43,45-47,50] | |
X17 | Difficult to choose a proper platform, and software and hardware | [4,38,49] |
DT: digital twin; AECO: architecture, engineering, construction, and operation.
3.2 DEMATEL-ISM-MICMAC approach
This paper innovatively adopts the integrated DEMATEL-ISM-MICMAC approach for DT barrier analysis. DEMATEL is a useful matrix-based mathematical method for determining how various barriers in a complicated system are interconnected[57]. ISM serves as a simulation and classification tool by converting usable data, detailing individual variables, delineating inter-variable relationships, and elucidating the rationale behind each connection[62,63]. The MICMAC approach divides barriers into four categories following the driving and dependence power[64], allowing for a deeper insight into the system[65]. In analyzing DT barriers, DEMATEL identifies and quantifies relationships, ISM structures and layers them, and MICMAC classifies them into different clusters. The integrated DEMATEL-ISM-MICMAC approach is a progressive process that deepens analysis step by step, aiding in systematically understanding and solving complex problems. Together, this integration provides a holistic understanding of dynamic interactions and structural relationships, making it effective for addressing the multifaceted challenges of adopting DT in the AECO industry.
DEMATEL, ISM, and MICMAC methods have demonstrated their effectiveness as powerful tools for capturing intricate relationships within a system. However, when utilized individually, each method emphasizes specific aspects and difficult to offer a comprehensive analysis of the influencing barriers within a complex system. The integrated approach overcomes the limitations of each method and enhances objectivity, comprehensiveness, and consistency in analyzing the influence and degree of interrelation among barriers[66]. Compared with other traditional approaches that focus on ranking or decision-making, such as the analytical hierarchy process and the technique for order preference by similarity to ideal solution, this integrated approach provides a more comprehensive analysis by exploring causal relationships, structural hierarchies, and dependency-priority mapping. Given the complementary and synergistic function of the DEMATEL, ISM, and MICMAC methods, this paper adopts the integrated approach for barrier analysis.
3.3 Data collection
After the identification of barriers, industry experts who have the relevant DT experience were invited to validate and score each barrier's degree of influence on other barriers in a 17×17 matrix-style questionnaire. The experts were requested to assess the degree of interaction between each of the two barriers through a four-level Likert scale ranging from 0 to 3. A score of "0" indicates the two barriers have no direct relation, "1" denotes a weak relation, "2" represents a general relation, and "3" signifies a strong relation. Following the collection of data, section 3.4 provides detailed data analysis PROCESS.
3.4 Data analysis techniques
In total, there are 12 steps for the DEMATEL-ISM-MICMAC analysis. Table 2 presents the specific calculation steps along with corresponding equations. Further elaboration on the methods and detailed explanations for each step of model establishment are provided in the subsequent paragraphs. The results for each intermediate step are shown in Appendix Table S2,S3,S4,S5,S6.
Steps | Eq. | No. |
Calculate the direct-influence matrix X (Table S2) | (1) | |
Calculate the normalized matrix N (Table S3) | (2) | |
Calculate the comprehensive influence matrix T (Table S4) | | (3) |
Calculate influence degree, influenced degree, centrality, and causality | (4) | |
(5) | ||
(6) | ||
(7) | ||
Calculate the global influence matrix H (Table S5) | (8) | |
Calculate the reachability matrix R (Table S6) | (9) | |
The intersection of the reachability set and antecedent set | (10) | |
MICMAC analysis | (11) | |
(12) |
MICMAC: cross-impact matrix multiplication applied to classification.
The value of Xij in matrix X is settled by calculating the collected average scores and then adjusted using Eq. (1). The normalized matrix N is determined via normalizing matrix X, as Eq. (2) shows. Matrix N equals matrix X divided by the maximum row sum of matrix X. Matrix T is calculated utilizing Eq. (3), where "I" represents the unit matrix. Matrix T can show both the direct and indirect relationship, whereas matrix X exclusively reflects the direct impact degree among barriers[67,68].
The influence degree, influenced degree, centrality, and causality are determined through matrix T. The influence degree of barrier i is represented by ei, which equals the sum of row i, as Eq. (4) shows. The influenced degree of barrier i is expressed by fi, the calculation of which is shown in Eq. (5). The influenced degree represents to what extent other barriers can impact the barrier. The centrality of barrier i is represented by ci, which is calculated by adding the value of influence and influenced degree, as Eq. (6) shows. As shown in Eq. (7), the value of the influenced degree is subtracted from the influence degree to determine the causality of barrier i, represented by ri. If the value of ri exceeds 0, the barrier acts as the cause barrier. Conversely, if the value of ri is below 0, the barrier serves as the result barrier. Matrix T takes the influence of other barriers into consideration instead of the barrier itself. Therefore, the global influence matrix H should be calculated based on the unit matrix and matrix T, as Eq. (8) shows.
The reachability matrix R can be converted from matrix H based on a threshold, λ, using Eq. (9). When the influence degree of a given barrier surpasses the predefined threshold, it signifies that the barrier possesses the capacity to directly influence other barriers. Conversely, if the influence degree remains below the threshold, the barrier is unable to exert a direct impact on other barriers. The threshold in the study is 0.15, which is determined by test results, actual simulations, and consulting experts[66,69-71]. The reachability set, denoted as R(Si), encompasses the barrier itself and those barriers influenced by it. The antecedent set, labeled as A(Si), includes the barrier itself and other barriers that can have an imapct on it. If the intersection of these two sets matches R(Si), as illustrated in Eq. (10), the barrier common to the intersection is classified within this level and eliminated from R(Si) when establishing the subsequent level[72]. The final step is the MICMAC analysis. By aggregating the count of rows within matrix R, the driving power of barriers can be determined, as depicted in Eq. (11). Similarly, the dependency power is calculated by summing the count of columns within matrix R, as shown in Eq. (12).
4. Results
4.1 Results of DEMATEL analysis
Table 3 demonstrates the influence degree, influenced degree, centrality, and causality of the 17 barriers. The causality and centrality can be determined by aggregating or subtracting the influenced and influence degrees. The ranking of centrality represents the importance of barriers from high to low.
Barrier | Influence degree | Influenced degree | Centrality | Causality | Ranking by centrality |
X1 | 3.158 | 3.228 | 6.385 | -0.070 | 3 |
X2 | 3.280 | 3.340 | 6.620 | -0.060 | 1 |
X3 | 3.189 | 2.999 | 6.187 | 0.190 | 4 |
X4 | 3.721 | 2.861 | 6.581 | 0.860 | 2 |
X5 | 2.267 | 3.466 | 5.733 | -1.200 | 7 |
X6 | 1.564 | 3.594 | 5.157 | -2.030 | 13 |
X7 | 2.075 | 3.472 | 5.547 | -1.397 | 9 |
X8 | 1.743 | 2.929 | 4.672 | -1.185 | 17 |
X9 | 2.313 | 2.489 | 4.801 | -0.176 | 16 |
X10 | 3.232 | 2.608 | 5.840 | 0.625 | 5 |
X11 | 3.339 | 1.712 | 5.050 | 1.627 | 14 |
X12 | 2.990 | 2.265 | 5.255 | 0.725 | 11 |
X13 | 2.510 | 2.679 | 5.189 | -0.169 | 12 |
X14 | 3.247 | 2.387 | 5.633 | 0.860 | 8 |
X15 | 3.157 | 2.658 | 5.815 | 0.499 | 6 |
X16 | 3.042 | 2.444 | 5.486 | 0.598 | 10 |
X17 | 2.610 | 2.305 | 4.915 | 0.304 | 15 |
The overall impact that a barrier can exert on other barriers is called its influence degree. According to Table 3, the top five high-influence barriers are X2, X4, X10, X11, and X14, all of which are categorized as environmental or technological barriers. Among these barriers, X4 is expected to have the most significant influence on other barriers, with a degree of influence measuring 3.721. This is because unclear understanding and undefined requirements for DT serve as a foundational issue that cascades into other barriers. For instance, if the requirements for DT are not well defined, it becomes challenging to develop appropriate application scenarios or advance key technologies such as 3D engine technology and real-time communication systems. The lack of clear government support and policies also exacerbates these challenges, as it hinders the creation of the necessary infrastructure and regulations to address the other technological barriers. Therefore, X4 is particularly influential, as resolving its uncertainties can positively impact the development of these interconnected barriers.
The combined influencing ability of other barriers is referred to as the influenced degree of this barrier. X1, X2, X5, X6, and X7 are the barriers particularly susceptible to being influenced by other barriers, all falling within the realm of environmental or organizational barriers. X6 is the barrier most prone to being influenced by other barriers, with a degree of influence measured at 3.594. These five barriers are particularly susceptible to being influenced by other barriers because they fall within the environmental or organizational realms, which are interconnected with external factors such as technological advancements, policy changes, and shifts in stakeholder perspectives. These barriers are influenced by changes in the development of DT technologies, regulatory frameworks, and industry trends. For example, as DT technologies become more mature and gain wider acceptance, barriers like the lack of industrial guidelines or government support can be mitigated. Similarly, improvements in technological capabilities or external policies can lead to more effective organizational strategies and build trust in DT, as stakeholders see tangible results. Among them, X6 is the most prone to influence, as trust in DT and long-term perspectives are directly shaped by the success of DT adoption and external support, making this barrier especially sensitive to changes in other areas.
Centrality represents the overall importance of a factor within the system, calculated as the sum of its "influence degree" and "influenced degree." A higher centrality value indicates that the factor is highly interactive, acting as both an influencer and a receiver of influence, making it critical in the network. Causality, on the other hand, is the difference between the "influence degree" and "influenced degree" and highlights the directional role of a factor. Positive causality values indicate that the factor primarily acts as a cause, exerting influence on others, while negative values show that it functions more as an effect, being influenced by others. These indicators provide insights into the dynamics of the system, helping to identify key drivers and outcomes. Among the 17 barriers, X3, X4, X10, X11, X12, X14, X15, X16, and X17 are cause barriers, as their causality values exceed zero. The rest of the barriers are result barriers as their causality values are below zero. Figure 2 illustrates the cause-effect diagram, with causality values represented on the vertical axis and centrality values on the horizontal axis. X11 has the highest causality, indicating the most robust influencing ability among all the barriers. X6 has the lowest causality, making it the most easily affected barrier. Barriers with higher centrality values hold greater significance within the system. X2 is the most crucial barrier hindering DT adoption, with a centrality of 6.620. The top four most important barriers all belong to environmental barriers, namely X1, X2, X3, and X4, all belong to environmental barriers.
4.2 Results of ISM analysis
The reachable and antecedent sets (Table S7) are determined through the reachability matrix R. Subsequently, a hierarchical structure model is developed to illustrate the interconnections and hierarchy among the barriers, as depicted in Figure 3. The 17 barriers are classified into three categories: direct-influence, fundamental, and intermediate-influence barriers. Barriers in Levels 1 and 2 are the direct-influence barriers. Barriers in Levels 3 and 4 are the intermediate-influence barriers. Barriers in Levels 5 and 6 are the fundamental barriers.
The direct-influence barriers can directly influence adopting DT in the AECO sector. X1, X2, X3, X5, X6, X7, and X8 represent the direct-influence barriers, all categorized under either environmental or organizational barriers. X6 has the potential to directly influence DT adoption as it occupies the highest level in the hierarchy structure. The hierarchy model shows that X7 can directly influence X6, validating experts' opinions that the rate of return of DT projects are the primary problem. Uncertain rate of returns may lead to insufficient client's trust in DT.
The fundamental barriers, located in Levels 5 and 6, can significantly influence the barriers at other levels. X11, X12, and X14 are the fundamental barriers, all belonging to technological barriers. In the hierarchy model, X11 is the fundamental barrier located in the lowest level, indicating that it is the root barrier and has the strongest influence ability on adopting DT among all 17 barriers. The intermediate-influence barriers serve as the linkage between the direct-influence and fundamental barriers. The direct-influence barriers can be impacted by the fundamental barriers via the intermediate-influence barriers located in the middle level, Levels 3 and 4. The intermediate-influence barriers include X4, X9, X10, X13, X15, X16, and X17.
4.3 Results of MICMAC analysis
The DEMATEL analysis quantifies the causal relationships and the significance of barriers. ISM reveals the hierarchical relationships and interaction patterns of the barriers within the system. Building on the DEMATEL and ISM analysis, MICMAC classifies barriers into four categories via different driving and dependence degrees: driving, autonomous, linkage, and dependent clusters, allowing for systematic identification and understanding of the influence and dependency of each barrier within the system. MICMAC analysis can identify key barriers, assess their interdependencies, and prioritize strategic actions, facilitating more informed decision-making processes. Figure 4 reveals the four distinct clusters identified through MICMAC analysis.
The barriers located in the autonomous cluster are considered to be excluded from the system. In this research, no barriers belong to the autonomous cluster, which means all barriers are interconnected and influence the system. X5, X6, X7, X8, X9, and X13, are the dependent barriers located in the dependent cluster, which are easily impacted by other barriers. The driving barriers include X11, X12, and X14, all of which are technological barriers. The driving barriers, characterized by low dependency and high driving power, are crucial as they significantly influence other variables and are essential for barrier control. The barriers in the driving cluster have a better-influencing ability on other barriers. In terms of the linkage barriers, any slight modification to the linkage barriers can influence other barriers in the system. The study identified linkage barriers as X1, X2, X3, X4, X10, X15, X16, and X17.
5. Discussion
This research promotes DT adoption in the AECO industry by analyzing barriers using the DEMATEL-ISM-MICMAC approach, offering practical recommendations to improve adoption rates. The primary findings of the paper include identifying and prioritizing DT barriers, revealing their interrelationships, and providing recommendations for their resolution or mitigation. The study shows that "X2 - lack of government financial and policy support", "X4 - unclear understanding and requirement of DT", and "X1 - lack of industrial guidelines and data standards" are the three most critical barriers impeding DT adoption, all belong to the organizational level. All three barriers are categorized into the linkage cluster as determined by the MICMAC analysis. These three barriers and the corresponding mitigation strategies are analyzed below.
Barrier "X2 - Lack of government financial and policy support". Prioritizing the improvement of funding and policy support is important due to the highest centrality of this barrier. The government's policy and economic backing can promote companies to invest more in DT projects with fewer concerns. This finding corresponds with several previous studies. Hazarika and Zhang[73] stated that policies can have a notable impact on adopting new technologies. Arowoiya et al.[74] concluded that subsidies are necessary for promoting DT for the AECO companies. To tackle this issue, proactive steps could be adopted involving advocating for financial incentives like tax breaks and subsidies, alongside fostering partnerships between the government and industry to develop supportive policies. Additionally, raising awareness about DT's potential economic benefits can help secure stronger government backing and encourage increased investment in DT technology.
Barrier "X4 - Unclear understanding and requirement of DT". Owners, contractors, and designers may lack a clear perception of the advantages and objectives of DT, resulting in unclear requirements and understanding of its implementation. Some people confuse the concept of DT with BIM[75,76]. BIM is a virtual replica of an asset, while DT is more than just a digital portrayal of the physical systems since it can indicate the current status of physical assets in real-time[77]. To overcome this barrier, it is essential to provide stakeholders with training on DT. Efforts should emphasize how DT offers real-time insights into asset performance, contrasting with BIM's static representations.
Barrier "X1 - Lack of industrial guidelines and data standards". The format of DT data can be diverse, and a standard approach throughout the life cycle of DT projects is necessary[34,40]. As industry standards continue to improve, an increasing number of DT projects will also achieve success. Therefore, it is necessary to develop and enhance industry guidelines and data standards specifically tailored to DT projects in the AECO industry, thereby fostering consistency and success across diverse projects.
The study findings also identified fundamental barriers and direct-influence barriers, while revealing the hierarchical structure among them. The most fundamental barriers are "X11 - immature 3D engine technology", "X12 - mismatching of IoT devices and DT", and "X14 - hard to realize real-time and bidirectional communication between virtual and physical objects", all belonging to the technological level. All three fundamental barriers are categorized into the driving factors cluster based on the MICMAC analysis. In addition, the direct-influence barrier that can impact DT adoption is "X6 - Lack of trust and long-term perspective in DT", belonging to the dependent cluster. Solving this issue can directly improve the acceptance rate of DT in the AECO sector. These four barriers and their corresponding mitigation strategies are discussed below.
Barrier "X11 - Immature 3D engine technology" is located at the lowest level of the hierarchy model, which means that immature 3D engine technology is the most fundamental barrier and needs to be solved first. A 3D engine is utilized to create the visual component of DT, facilitating the development of user-friendly interfaces. Simulation is the most intrinsic driving force of developing DT in the AECO industry[78,79], but 3D engine technology is still a critical enabler, providing the necessary visual representation and data integration that enhances the effectiveness of simulations. Although DT does not entirely rely on 3D engine technology and several other technologies can be adopted by DT[80], the immaturity of 3D engine technology still appears to be a significant barrier hindering the development of DT. VanDerHorn and Mahadevan[59] concluded that 3D engine technology-driven visualization can enhance DT implementation by providing decision-makers with clear data insights. According to the experts, the expense and technical requirements for 3D engine technology are high. Besides, each 3D engine platform has different strengths and weaknesses, and it is difficult to have one platform to integrate all the advantages. There are some methods to improve the 3D engine technology, such as adopting more powerful processors and graphics processing units to improve the interaction effects. Collaboration between industry stakeholders and technology developers can help identify and address technical challenges while working toward standardization to mitigate compatibility issues. Additionally, investing in training programs about utilizing existing 3D engine platforms can optimize their effectiveness in creating user-friendly interfaces for DT.
Barrier "X12 - Mismatching of IoT devices and DT". The use of DT relies on the integration of several technologies[81], including IoT for real-time data collection. The underdevelopment of IoT devices leads to the low latency of digital objectives responding to physical assets[82]. This validates the relationship between "X12 - mismatching of IoT devices and DT" and "X14 - hard to realize real-time and bidirectional communication between virtual and physical objects". This finding corresponds with Lawal and Rafsanjani[83] statement that sometimes DT tools may not be compatible with sensors or IoT devices. Integrating C4.0 technologies together is crucial for maximizing the efficiency of DT utilization[84]. To overcome the obstacle, prioritizing the development and integration of IoT technologies is essential. This enables real-time data collection and bidirectional communication among digital and physical assets, promoting compatibility and minimizing latency issues.
Barrier "X14 - Hard to realize real-time and bidirectional communication between virtual and physical objects". According to the interviewed experts, DT collects physical data and displays models on virtual platforms. Automated analysis of DT models facilitates real-time alerts and control over physical assets, including equipment switching and fire protection measures. This aligns with the research conducted by Segovia and Garcia-Alfaro[56], which highlighted the unidirectional connection between DT and physical assets. They emphasized the importance for bidirectional data to control physical assets based on digital data. There are many aspects that need to be improved to promote real-time and bidirectional communication, such as the high precision of DT model, real-time data acquisition, and decision-making algorithms. To address this issue, implementing edge computing can enhance processing efficiency and enable faster decision-making. Robust cybersecurity measures will protect the integrity and security of bidirectional data exchange, ensuring reliable control over physical assets based on virtual data.
Barrier "X6 - Lack of trust and long-term perspective in DT" can hinder the adoption of DT technology, as stakeholders may doubt its reliability and its potential to provide a sufficient rate of return attributed to insufficient successful case studies and the technology's nascent stage in the AECO industry. To sole this problem, it is necessary to showcase successful pilot projects, provide transparent performance data, and emphasize long-term benefits to build trust and demonstrate the technology's viability. To ensure data reliability and trust in DT-based smart systems, organizations must meet client requirements, integrate secure architectures, and continually improve, with smart contract-based systems enhancing data security, privacy, and trust[85]. In addition, the government should provide incentives or subsidies to companies that adopt DT. This suggestion aligns with addressing barrier "X2 - Lack of government financial and policy support", highlighting the mutual influence of these two barriers.
In general, this study provides a systematic framework to understand the barriers to the adoption of DT technology in the AECO industry within the context of C4.0. By integrating the DEMATEL, ISM, and MICMAC methods, the research uncovers the hierarchical relationships and causal connections among various barriers, quantifying their influence and significance. This paper provides a comprehensive analysis of the interrelationships between barriers, identifying the most critical, direct-influence, and fundamental obstacles to DT adoption. This research fills gaps in existing literature, advancing the development of DT adoption theories and providing valuable academic support for the digital transformation of the AECO industry under C4.0.
The research findings also provide managerial recommendations for AECO practitioners aiming to promote the adoption of DT. Resolving the lack of government policy and financial support should be prioritized. In addition, addressing issues such as unclear understanding and requirements of DT, and the absence of industrial guidelines and data standards are crucial. Prioritizing improvements in funding, policy incentives, tax reduction, education related to DT, and establishing industry standards are recommended for overcoming these barriers. At the technological level, resolving challenges related to immature 3D engine technology, mismatching of IoT devices and DT, and achieving real-time bidirectional communication between virtual and physical objects is imperative. Adopting more powerful processors and graphics processing units to improve the interaction effects, accelerating IoT device development, and improving the precision of DT models, real-time data acquisition, and decision-making algorithms will help to overcome these barriers.
6. Conclusions
This paper thoroughly analyzes the barriers impeding the integration of DT in the AECO sector under the context of C4.0 using the combined methods of DEMATEL, ISM, and MICMAC. The study identifies that "lack of government financial and policy support" is the most critical barrier to implementing DT in the AECO industry, due to insufficient incentives for high-cost, uncertain-return investments. Additionally, "lack of trust and long-term perspective in DT" is the most significant direct-influence barrier, driven by concerns over reliability, security, and alignment with long-term goals. Furthermore, the research highlights that the most fundamental barrier requiring immediate attention is the immaturity of 3D engine technology, which serves as a critical enabler for DT implementation. By addressing these barriers, the study provides actionable insights to prioritize efforts for advancing DT adoption in the AECO industry. Meanwhile, the paper uncovers the hierarchical relationships and interconnectedness among the identified barriers to DT adoption through a comprehensive hierarchy model. This model not only clarifies the influence pathways between barriers but also provides a basis for prioritizing solutions. By leveraging the insights and recommendations provided, industry practitioners can develop further strategies to enhance the integration of DT under C4.0 within the AECO industry.
However, there are certain limitations of the research. The investigation, conducted in China with experts from Chinese construction companies, may lead to results applicable only to the Chinese context. Given that the evolution of DT in China's AECO sector is in its initial phase and there is a shortage of experts, future research should explore different countries to provide a broader perspective. In addition, the dynamic nature and fast development speed of DT technology means that identified barriers may change over time. To address this, continuous monitoring and updating of barriers alongside periodic follow-up studies are essential. Future studies could develop a flexible framework for barrier analysis to ensure the research remains reflective of the evolving technological landscape. Additionally, in future research, the exploration of maturity evaluation systems for DT technology, particularly in the AECO sector, can be expanded to help identify and overcome implementation barriers. Additionally, bibliometric analysis tools such as VOSviewer, CiteSpace, and Gephi can be utilized to objectively analyze the academic dynamics of DT, providing deeper insights into citation relationships, collaboration networks, and keyword co-occurrence. The integration of these methods will enhance the understanding of DT technology development and offer data-driven support for future studies.
Supplementary materials
The supplementary material for this article is available at: Supplementary materials.
Authors contribution
Zhao W: Article conception and design, data acquisition, data analysis and interpretation.
Wang K, Guo F: Data acquisition, data analysis and interpretation.
Zhang Q, Qi J: Data acquisition.
Hao J: Data analysis and interpretation.
Conflicts of interest
Fangyu Guo received financial support from the Glodon company. Other authors declared that there are no conflicts of interest.
Ethical approval
This research has obtained the approval from our university research ethics review panel with the document No. ER-DES-0010000037820230510161636.
Consent to participate
Informed consent statement was provided at the beginning of the questionnaire.
Consent for publication
Not applicable.
Availability of data and materials
Not applicable.
Funding
This work was supported by the Glodon Company under Grant RDS10120220066.
Copyright
© The Author(s) 2025.
References
-
1. Statsenko L, Samaraweera A, Bakhshi J, Chileshe N. Construction 4.0 technologies and applications: a systematic literature review of trends and potential areas for development. Constr Innov. 2023;23(5):961-993.
[DOI] -
2. Siriwardhana S, Moehler R. Mastering the skills of Construction 4.0: a review of the literature using science mapping. Smart Sustain Built Environ. 2023;13(4):989-1014.
[DOI] -
3. Kineber AF, Singh AK, Fazeli A, Mohandes SR, Cheung C, Arashpour M, et al. Modelling the relationship between digital twins implementation barriers and sustainability pillars: Insights from building and construction sector. Sustain Cities Soc. 2023;99:104930.
[DOI] -
4. Perno M, Hvam L, Haug A. Implementation of digital twins in the process industry: A systematic literature review of enablers and barriers. Comput Ind. 2022;134:103558.
[DOI] -
5. Delgado JMD, Oyedele L. Digital Twins for the built environment: learning from conceptual and process models in manufacturing. Adv Eng Inform. 2021;49:101332.
[DOI] -
6. Ozturk GB. Digital twin research in the AECO-FM industry. J Build Eng. 2021;40:102730.
[DOI] -
7. Chen Z, Lu J, Wang X, Pedrycz W. Identifying digital transformation barriers in small and medium-sized construction enterprises: a multi-criteria perspective. J Knowl Econ. 2024;15:15959-15995.
[DOI] -
8. Greif T, Stein N, Flath CM. Peeking into the void: digital twins for construction site logistics. Comput Ind. 2020;121:103264.
[DOI] -
9. Osorio-Gómez CC, Herrera RF, Prieto-Osorio JM, Pellicer E. Conceptual model for implementation of digital transformation and organizational structure in the construction sector. Ain Shams Eng J. 2024;15(7):102749.
[DOI] -
10. Hao JL, Zhao W, Gong G, Ma W, Li L, Zhang Y. Catalyzing sustainability through prefabrication: Integrating BIM-LCA for assessing embodied carbon in timber formwork waste. Sustain Chem Pharm. 2024;41:101698.
[DOI] -
11. Labaran YH, MathurVS , Muhammad SU, Musa AA. Carbon footprint management: a review of construction industry. Clean Eng Technol. 2022;9:100531.
[DOI] -
12. Boje C, Menacho ÁJH, Marvuglia A, Benetto E, Kubicki S, Schaubroeck T, et al. A framework using BIM and digital twins in facilitating LCSA for buildings. J Build Eng. 2023;76:107232.
[DOI] -
13. Marsh E, Allen S, Hattam L. Tackling uncertainty in life cycle assessments for the built environment: a review. Build Environ. 2023;231:109941.
[DOI] -
14. Song P, Wu L, Zhao W, Ma W, Hao J. Life cycle sustainability assessment: an index system for building energy retrofit projects. Buildings. 2024;14(9):2817.
[DOI] -
15. Zhao W, Hao JL, Gong G, Ma W, Zuo J, Di Sarno. Decarbonizing prefabricated building waste: Scenario simulation of policies in China. J Clean Prod. 2024;458:142529.
[DOI] -
16. Craveiro F, Duarte JP, Bartolo H, Bartolo PJ. Additive manufacturing as an enabling technology for digital construction: A perspective on Construction 4.0. Autom Constr. 2019;103:251-267.
[DOI] -
17. Moshood TD, Rotimi JO, Shahzad W, Bamgbade J. Infrastructure digital twin technology: a new paradigm for future construction industry. Technol Soc. 2024;77:102519.
[DOI] -
18. Bajpai A, Misra SC. Barriers to implementing digitalization in the Indian construction industry. Int J Qual Reliab Manag. 2022;39(10):2438-2464.
[DOI] -
19. Mohandes SR, Singh AK, Fazeli A, Banihashemi S, Arashpour M, Cheung C, et al. Determining the stationary digital twins implementation barriers for sustainable construction projects. Smart Sustain Built Environ. 2024.
[DOI] -
20. Boje C, Guerriero A, Kubicki S, Rezgui Y. Towards a semantic Construction Digital Twin: Directions for future research. Autom Constr. 2020;114:103179.
[DOI] -
21. Pan Y, Zhang L. A BIM-data mining integrated digital twin framework for advanced project management. Autom Constr. 2021;124:103564.
[DOI] -
22. Wang X, Liang CJ, Menassa CC, Kamat VR. Interactive and Immersive Process-Level Digital Twin for Collaborative Human-Robot Construction Work. J Comput Civil Eng. 2021;35(6):04021023.
[DOI] -
23. Saporiti N, Cannas VG, Pozzi R, Rossi T. Challenges and countermeasures for digital twin implementation in manufacturing plants: a Delphi study. Int J Prod Econ. 2023;261:108888.
[DOI] -
24. Weil C, Bibri SE, Longchamp R, Golay F, Alahi A. Urban Digital Twin Challenges: A Systematic Review and Perspectives for Sustainable Smart Cities. Sustain Cities Soc. 2023;99:104862.
[DOI] -
25. Deepu T, Ravi V. Exploring critical success factors influencing adoption of digital twin and physical internet in electronics industry using grey-DEMATEL approach. Digit Bus. 2021;1:100009.
[DOI] -
26. Zhao W, Hao JL, Gong G, Fischer T, Liu Y. Applying digital technologies in construction waste management for facilitating sustainability. J Environ Manag. 2025;373:123560.
[DOI] -
27. Mohtaramzadeh M, Ramayah T, Jun-hwa C. B2B E-Commerce Adoption in Iranian Manufacturing Companies: Analyzing the Moderating Role of Organizational Culture. Int J Hum-Comput Interact. 2017;34:621-639.
[DOI] -
28. Chen ZS, Wang Y, Xu YQ, Zhu Z, Chen YH, Skibniewski MJ. Towards social-welfare and confidence optimizing approach to examining barriers for digital transformation in SMCEs. Inf Sci. 2024;663:120312.
[DOI] -
29. Ma W, Liu T, Hao JL, Wu W, Gu X. Towards a circular economy for construction and demolition waste management in China: critical success factors. Sustain Chem Pharm. 2023;35:101226.
[DOI] -
30. Omrany H, Al-obaidi KM, Husain A, Ghaffarianhoseini A. Digital twins in the construction industry: a comprehensive review of current implementations, enabling technologies, and future directions. Sustainability. 2023;15:10908.
[DOI] -
31. Coupry C, Noblecourt S, Richard P, Baudry D, Bigaud D. BIM-Based Digital Twin and XR Devices to Improve Maintenance Procedures in Smart Buildings: A Literature Review. Appl Sci. 2021;11(15):6810.
[DOI] -
32. Feng H, Chen Q, de Soto BG. Application of digital twin technologies in construction: an overview of opportunities and challenges. In: 38th International Symposium on Automation and Robotics in Construction; 2021; Nov 2-4; Dubai, UAE. Reston: American Society of Civil Engineers; 2021. p. 979-986.
[DOI] -
33. Fuller A, Fan Z, Day C, Barlow C. Digital Twin: Enabling Technologies, Challenges and Open Research. IEEE Access. 2020;8:108952-108971.
[DOI] -
34. Gadekar R, Sarkar B, Gadekar A. Model development for assessing inhibitors impacting Industry 4.0 implementation in Indian manufacturing industries: an integrated ISM-Fuzzy MICMAC approach. Int J Syst Assur Eng Manag. 2022;15:646-671.
[DOI] -
35. Kutnjak A. Covid-19 Accelerates Digital Transformation in Industries: Challenges, Issues, Barriers and Problems in Transformation. IEEE Access. 2021;9:79373-79388.
[DOI] -
36. Olanipekun AO, Sutrisna M. Facilitating Digital Transformation in Construction-A Systematic Review of the Current State of the Art. Front Built Environ. 2021;7:660758.
[DOI] -
37. Rasheed A, San O, Kvamsdal T. Digital Twin: Values, Challenges and Enablers From a Modeling Perspective. IEEE Access. 2020;8:21980-22012.
[DOI] -
38. Shahzad M, Shafiq MT, Douglas D, Kassem M. Digital Twins in Built Environments: An Investigation of the Characteristics, Applications, and Challenges. Buildings. 2022;12(2):120.
[DOI] -
39. Wanasinghe TR, Wroblewski L, Petersen BK, Gosine RG, James LA, De Silva, et al. Digital Twin for the Oil and Gas Industry: Overview, Research Trends, Opportunities, and Challenges. IEEE Access. 2020;8:104175-104197.
[DOI] -
40. Wang K, Guo F, Zhang C, Schaefer D. From Industry 4.0 to Construction 4.0: barriers to the digital transformation of engineering and construction sectors. Eng Constr Archit Manag. 2022;31(1):136-158.
[DOI] -
41. Alaloul WS, Liew MS, Zawawi NAWA, Kennedy IB. Industrial Revolution 4.0 in the construction industry: Challenges and opportunities for stakeholders. Ain Shams Eng J. 2020;11(1):225-230.
[DOI] -
42. Kumar S, Suhaib M, Asjad M. Narrowing the barriers to Industry 4.0 practices through PCA-Fuzzy AHP-K means. J Adv Manag Res. 2020;18(2):200-226.
[DOI] -
43. Ozkan-ozen YD, Kazancoglu Y, Mangla SK. Synchronized barriers for circular supply chains in industry 3.5/industry 4.0 transition for sustainable resource management. Resour Conserv Recycl. 2020;161:104986.
[DOI] -
44. Raj A, Dwivedi G, Sharma A, de Sousa, Rajak S. Barriers to the adoption of industry 4.0 technologies in the manufacturing sector: An inter-country comparative perspective. Int J Prod Econ. 2020;224:107546.
[DOI] -
45. Karadayi-usta S. An Interpretive Structural Analysis for Industry 4.0 Adoption Challenges. IEEE Trans Eng Manag. 2020;67(3):973-978.
[DOI] -
46. Tripathi S, Gupta M. Impact of barriers on industry 4.0 transformation dimensions. In: International Conference on Precision, Meso, Micro and Nano Engineering (COPEN 2019); 2019 Dec 12-14; Indore, India. Berlin: Springer; 2019. Available from: https://www.researchgate.net/profile/Shubham-Tripathi-9/publication.pdf
-
47. Tuhaise VV, Tah JHM, Abanda FH. Technologies for digital twin applications in construction. Autom Constr. 2023;152:104931.
[DOI] -
48. Bosch-sijtsema P, Claeson-jonsson C, Johansson M, Roupe M. The hype factor of digital technologies in AEC. Constr Innov. 2021;21(4):899-916.
[DOI] -
49. Moktadir MA, Ali SM, Kusi-sarpong S, Shaikh MAA. Assessing challenges for implementing Industry 4.0: Implications for process safety and environmental protection. Process Saf Environ Prot. 2018;117:730-741.
[DOI] -
50. Sharma A, Kosasih E, Zhang J, Brintrup A, Calinescu A. Digital Twins: State of the art theory and practice, challenges, and open research questions. J Ind Inf Integr. 2022;30:100383.
[DOI] -
51. Hassan AM, Negash YT, Hanum F. An assessment of barriers to digital transformation in circular Construction: An application of stakeholder theory. Ain Shams Eng J. 2024;15(7):102787.
[DOI] -
52. Alshammari K, Beach T, Rezgui Y. Industry Engagement for Identification of Cybersecurity Needs Practices for Digital Twins. In: 2021 IEEE International Conference on Engineering, Technology and Innovation (ICE/ITMC); 2021 Jun 21-23. Cardiff, United Kingdom. New York: IEEE; 2021. p. 1-7.
[DOI] -
53. Autiosalo J, Ala-laurinaho R, Mattila J, Valtonen M, Peltoranta V, Tammi K. Towards Integrated Digital Twins for Industrial Products: Case Study on an Overhead Crane. Appl Sci. 2021;11(2):683.
[DOI] -
54. Karaarslan E, Babiker M. Digital Twin Security Threats and Countermeasures: An Introduction. In: 2021 International Conference on Information Security and Cryptology (ISCTURKEY); 2021 Dec 2-3; Ankara, Turkey. New York: IEEE; 2021. p. 7-11.
[DOI] -
55. Segovia M, Garcia-alfaro J. Design, modeling and implementation of digital twins. Sensors. 2022;22(14):5396.
[DOI] -
56. Si SL, You XY, Liu HC, Zhang P. DEMATEL Technique: A Systematic Review of the State-of-the-Art Literature on Methodologies and Applications. Math Probl Eng. 2018;2018:1-33.
[DOI] -
57. Turner CJ, Oyekan J, Stergioulas L, Griffin D. Utilizing Industry 4.0 on the Construction Site: Challenges and Opportunities. IEEE Trans Ind Informat. 2021;17(2):746-756.
[DOI] -
58. Vanderhorn E, Mahadevan S. Digital Twin: Generalization, characterization and implementation. Decis Support Syst. 2021;145:113524.
[DOI] -
59. Wang Y, Wang X, Liu A, Zhang J, Zhang J. Ontology of 3D virtual modeling in digital twin: a review, analysis and thinking. J Intell Manuf. 2023;36:95-145.
[DOI] -
60. Rafsanjani HN, Nabizadeh AH. Towards digital architecture, engineering, and construction (AEC) industry through virtual design and construction (VDC) and digital twin. Energy Built Environ. 2023;4(2):169-178.
[DOI] -
61. Artpairin A, Pinmanee S. Critical success factors in the management of petrochemical construction projects for contractors and subcontractors during the COVID-19 pandemic. Int J Constr Manag. 2022;23(11):1956-1968.
[DOI] -
62. Yuan H, Du W, Zuo J, Ma X. Paving a traceable green pathway towards sustainable construction: a fuzzy ISM-DEMATEL analysis of blockchain technology adoption barriers in construction waste management. Ain Shams Eng J. 2024;15(4):102627.
[DOI] -
63. Ebrahimi M, Daneshvar A, Valmohammadi C. Using a comprehensive DEMATEL-ISM-MICMAC and importance–performance analysis to study sustainable service quality features. J Econ Adm Sci. 2024.
[DOI] -
64. Wu P, Chen Q, Chen Y, Chen S, Zou J. ISM-MICMAC based safety risk sources analysis and control measures for underground engineering of urban rail transit projects. J Eng Res. 2023;11(3):40-50.
[DOI] -
65. Liang Y, Wang H, Zhao X. Analysis of factors affecting economic operation of electric vehicle charging station based on DEMATEL-ISM. Comput Ind Eng. 2022;163:107818.
[DOI] -
66. Huo T, Cong X, Cheng C, Cai W, Zuo J. What is the driving mechanism for the carbon emissions in the building sector? An integrated DEMATEL-ISM model. Energy. 2023;274:127399.
[DOI] -
67. Wang L, Cao Q, Zhou L. Research on the influencing factors in coal mine production safety based on the combination of DEMATEL and ISM. Saf Sci. 2018;103:51-61.
[DOI] -
68. Gong C, Xu H, Xiong F, Zuo J, Dong N. Factors impacting BIM application in prefabricated buildings in China with DEMATEL-ISM. Constr Innov. 2023;23(1):19-37.
[DOI] -
69. Kamble SS, Gunasekaran A, Sharma R. Analysis of the driving and dependence power of barriers to adopt industry 4.0 in Indian manufacturing industry. Comput Ind. 2018;101:107-119.
[DOI] -
70. Trivedi A, Jakhar SK, Sinha D. Analyzing barriers to inland waterways as a sustainable transportation mode in India: A dematel-ISM based approach. J Clean Prod. 2021;295:126301.
[DOI] -
71. Li D, Li X, Feng H, Wang Y, Fan S. ISM-based relationship among critical factors that affect the choice of prefabricated concrete buildings in China. Int J Constr Manag. 2019;22(6):977-992.
[DOI] -
72. Hazarika N, Zhang X. Factors that drive and sustain eco-innovation in the construction industry: The case of Hong Kong. J Clean Prod. 2019;238:117816.
[DOI] -
73. Arowoiya VA, Oke AE, Ojo LD, Adelusi AO. Driving Factors for the Adoption of Digital Twin Technology Implementation for Construction Project Performance in Nigeria. J Constr Eng Manag. 2024;150(1):05023014.
[DOI] -
74. Khajavi SH, Motlagh NH, Jaribion A, Werner LC, Holmstrom J. Digital Twin: Vision, Benefits, Boundaries, and Creation for Buildings. IEEE Access. 2019;7:147406-147419.
[DOI] -
75. Opoku DGJ, Perera S, Osei-kyei R, Rashidi M, Bamdad K, Famakinwa T. Barriers to the Adoption of Digital Twin in the Construction Industry: A Literature Review. Informatics. 2023;10(1):14.
[DOI] -
76. Broo DG, Schooling J. Digital twins in infrastructure: definitions, current practices, challenges and strategies. Int J Constr Manag. 2021;23:1254-1263.
[DOI] -
77. Torzoni M, Tezzele M, Mariani S, Manzoni A, Willcox KE. A digital twin framework for civil engineering structures. Comput Methods Appl Mech Eng. 2024;418:116584.
[DOI] -
78. Yang Y, Li M, Yu C, Zhong RY. Digital twin-enabled visibility and traceability for building materials in on-site fit-out construction. Autom Constr. 2024;166:105640.
[DOI] -
79. Opoku DGJ, Perera S, Osei-kyei R, Rashidi M. Digital twin application in the construction industry: A literature review. J Build Eng. 2021;40:102726.
[DOI] -
80. Javaid M, Haleem A, Suman R. Digital Twin applications toward Industry 4.0: A Review. Cogn Robot. 2023;3:71-92.
[DOI] -
81. Liu M, Fang S, Dong H, Xu C. Review of digital twin about concepts, technologies, and industrial applications. J Manuf Syst. 2021;58:346-361.
[DOI] -
82. Lawal K, Rafsanjani HN. Trends, benefits, risks, and challenges of IoT implementation in residential and commercial buildings. Energy Built Environ. 2022;3:251-266.
[DOI] -
83. Wang K, Guo F, Zhang C, Hao J, Wang Z. Unlocking determinants of smart construction: an integrated model of UTAUT2, TTF, and perceived risk for IoT acceptance in AEC industry. Eng Constr Archit Manag. 2024.
[DOI] -
84. Sasikumar A, Vairavasundaram S, Kotecha K, Indragandhi V, Ravi L, Selvachandran G, et al. Blockchain-based trust mechanism for digital twin empowered Industrial Internet of Things. Future Gener Comput Syst. 2023;141:16-27.
[DOI]
Copyright
© The Author(s) 2025. This is an Open Access article licensed under a Creative Commons Attribution 4.0 International License (https://creativecommons.org/licenses/by/4.0/), which permits unrestricted use, sharing, adaptation, distribution and reproduction in any medium or format, for any purpose, even commercially, as long as you give appropriate credit to the original author(s) and the source, provide a link to the Creative Commons license, and indicate if changes were made.
Share And Cite