Abstract
Amid growing global attention to occupational health and safety, the construction industry faces critical challenges associated with engineered stone, which emits high concentrations of respirable crystalline silica during processing and has been linked to severe lung diseases. In response, Australia enacted a comprehensive nationwide ban on engineered stone in July 2024. Drawing on media framing theory, this study analyzes public discourse and sentiment surrounding the ban by examining 7,198 comments collected from Reddit and YouTube. Through Latent Dirichlet Allocation, three dominant themes emerged: health risks and safety concerns, economic impacts and industry transition, and regulatory implementation. Sentiment analysis revealed that 55.5% of the comments expressed negative sentiment, mainly centered on economic concerns, while 21.3% were positive, emphasizing health benefits, and 23.1% were neutral. Economic impact frames predominated among negative comments, whereas health risk frames were more common in positive ones. These findings suggest that future policy communications should more effectively integrate narratives around both health protection and economic transition. This study contributes to the methodological development of sentiment analysis and offers practical insights for policy formulation and implementation.
Keywords
1. Introduction
Engineered stone, also referred to as artificial stone or composite stone, has gained considerable popularity and widespread use in global architecture and interior design. Commercially available engineered stone products are typically composed of a mixture of quartz powder and resin, making them a preferred choice for both residential and commercial applications due to their aesthetic appeal, durability, and low production and maintenance costs[1]. However, despite its broad market acceptance, the processing and use of engineered stone have raised significant health concerns. Numerous studies have reported that engineered stone contains a high proportion of respirable crystalline silica (RCS), exposing workers to hazardous dust during cutting, grinding, and polishing processes. Such exposure has been linked to silicosis, a severe and irreversible occupational lung disease[2-4].
The global rise in silicosis cases has led to renewed scrutiny of the safety of engineered stone. Although many countries have adopted enhanced workplace safety measures, such as improved personal protective equipment and the implementation of wet cutting techniques, to reduce workers’ exposure to respirable crystalline silica[5-9], these interventions have not fully eliminated the health risks associated with occupational exposure[10-12]. In response to these concerns, Australia has taken unprecedented regulatory action. According to the final report released by Safe Work Australia in 2023, which reviewed extensive evidence on silica exposure during engineered stone processing, regulatory authorities concluded that a complete ban was the most effective wayto protect workers. Following this recommendation, a series of events unfolded[13]:
• February 2023: Work Health and Safety (WHS) ministers requested that Safe Work Australia develop a comprehensive plan to ban engineered stone.
• October 27, 2023: Safe Work Australia released its final report, strongly recommending a total ban on the use, supply, processing, and installation of engineered stone due to its high crystalline silica content.
• December 13, 2023: WHS ministers from the Commonwealth, states, and territories unanimously voted to implement the ban.
• July 1, 2024: Australia became the first country in the world to enforce a nationwide ban on engineered stone benchtops, panels, and slabs.
• September 1, 2024: Additional amendments took effect, introducing stricter regulation on all materials containing at least 1% crystalline silica.
• January 1, 2025: A ban on the importation of engineered stone products was enacted, further reinforcing the national regulatory framework.
In contrast, other countries, particularly those in Europe and North America, have primarily emphasized technological improvements and occupational health monitoring, rather than imposing a complete ban on engineered stone[14-16]. Australia’s decisive regulatory action has not only reignited global discussions on r the governance of engineered stone but has also prompted other nations to consider whether they should adopt similar measures[17].
In Australia, the use and processing of engineered stone have also drawn significant public attention in recent years. Surveys indicate that the engineered stone market is sizable, accounting for over 50% of countertop applications nationwide. However, as an increasing number of stone fabrication workers have been diagnosed with silicosis, both the public and policymakers have begun to question the safety of this material. Reports from Australian health authorities reveal that exposure to high concentrations of respirable crystalline silica during the processing and installation of engineered stone has led to a series of occupational health crises[18-20].
Existing research has predominantly focused on the physical characteristics of engineered stone and its associated occupational health risks. In particular, prior studies have established that respirable crystalline silica poses a significant threat to workers’ health. However, comparatively little attention has been paid to public perceptions of engineered stone, especially in the context of recent regulatory changes and proposed bans. Drawing on media framing theory[21,22], this study posits that the way health risks and regulatory measures are framed in public discourse plays a critical role in shaping policy acceptance and implementation[23]. Understanding public attitudes and perceptions through the lens of framing theory is essential for policy development, as these factors not only influence the level of public support but may also affect consumer behavior and the broader trajectory of the industry[24].
According to existing literature, sentiment analysis has been widely applied in studies exploring public attitudes toward health, policy, and environmental issues. Building upon this methodological foundation and guided by framing theory, the present study applies sentiment analysis and topic modeling techniques to systematically examine how different frames influence Australian public attitudes toward the engineered stone ban[25-27]. This research not only aims to address the existing gap in understanding public perceptions but also seeks to provide robust methodological and analytical support for policymakers in developing future regulatory measures. The specific objectives of the study are as follows:
• To examine the dominant sentiment trends and thematic variations in public discourse surrounding the engineered stone ban, thereby offering insights into prevailing public attitudes.
• To identify the primary factors driving public support for or opposition to the ban, uncovering key influences that shape public opinion.
2. Literature Review
2.1 Overview of engineered stone
2.1.1 Types of engineered stone
Engineered stone typically refers to composite materials that contain a significant proportion of mineral fillers, most commonly quartz, bonded by a resin or cement-based binder. However, the term is sometimes used more broadly in the literature to include polymer-rich composites that only partially resemble traditional stone in their properties. To clarify these variations, some studies distinguish between two main categories:
Resin-Dominated Composites (Polymer-Based)
These materials primarily use synthetic polymers, such as epoxy resin, polymethyl methacrylate, or polyester resin, as their principal binding agent[28-30]. While they incorporate mineral-based fillers, the polymer component is typically the dominant phase. These composites are known for their chemical plasticity and relatively low processing temperatures, although they exhibit limited heat resistance[31,32]. The polymerization reactions (e.g., epoxy curing) form a robust three-dimensional cross-linked network, providing good impact resistance and chemical corrosion resistance[33]. Due to their flexibility and overall durability, polymer-based composites are often deemed suitable for building applications[34,35].
Mineral-Dominated Composites (High-Silica or Cement-Based)
In contrast, many commercially available products labeled as “engineered stone” are predominantly composed of minerals. These composites often incorporate inorganic binders, such as cement or lime, combined with quartz sand or other silica-rich aggregates[36,37]. Typically processed using thermoset mechanisms, these materials are known for their high heat and fire resistance, as well as their favorable weather durability. However, less stringent production processes may involve low-cost waste fillers (e.g., stone slurry, limestone powder), which can lead to reduced mechanical strength and lower economic value. Furthermore, the production of cement and lime is associated with significant carbon dioxide emissions, raising environmental concerns[38].
In the context of the Australian ban and in most industrial usage, the term “engineered stone” typically refers to mineral-dominated composites, particularly quartz-based materials with a high silica content. The polymer-rich variants described above are less commonly implicated in the occupational health concerns targeted by this study, largely because the Australian legislation specifically addresses the risk of silicosis associated with materials containing substantial amounts of crystalline silica.
2.1.2 Extraction and environmental issues associated with engineered stone
The production of engineered stone involves the extraction of raw materials such as quartz and marble, processes that are often linked to environmental degradation. As documented in the historiography of stone extraction and reinforced by contemporary research, quarrying can have significant ecological impacts, including habitat disruption, high water consumption, and substantial energy use[39,40]. Additionally, the generation of dust during production poses risks not only to worker health but also to the surrounding environment. The incorporation of resin in engineered stone further raises concerns, particularly regarding chemical pollution stemming from the production and disposal of resin-based materials[41]. While dust-related hazards have been extensively studied in quarrying and mining contexts, there is a notable lack of comprehensive research assessing the environmental impacts of engineered stone across its entire lifecycle, from raw material extraction and manufacturing to usage and end-of-life disposal. This gap highlights the need for further investigation into the full environmental footprint of engineered stone, especially considering its distinctive composition and processing methods.
2.1.3 Australian engineered stone ban
Australia has been a major market for engineered stone, particularly in interior applications for residential and commercial construction. However, the growing use of this material has been accompanied by increasing occupational health risks, prompting widespread concern. In response, the Australian government introduced a series of regulations aimed at reducing workers’ exposure to RCS during the processing of high-silica engineered stone[16]. Effective from July 1, 2024, Australia implemented a nationwide ban on engineered stone to address the alarming rise in silicosis cases. This legislation imposes strict restrictions on the production, sale, and installation of engineered stone. In contrast to countries, in Europe and North America, which primarily rely on technological controls to manage dust exposure, Australia has taken a legislative approach by prohibiting the use of this high-risk material entirely. This ban has had significant implications for the construction and manufacturing sectors. As a result, many stakeholders are now actively exploring alternative materials that can provide comparable functional and aesthetic qualities to engineered stone, while being entirely free of hazardous crystalline silica[42].
2.1.4 Occupational health and safety and control measures in Australia
Due to the high concentration of RCS in the dust produced during the processing of engineered stone, Australia’s WHS framework has adopted a multi-tiered approach to risk mitigation . Each Australia state enforces its own specific prevention and control standards tailored to local conditions and industry practices. For example, regulations in Queensland mandate the use of wet cutting techniques and powered air-purifying respirators when operating machinery such as bridge saws, in order to minimize dust dispersion[43]. Additionally, employers are required to conduct regular health monitoring for workers to facilitate early detection of silica-related illnesses[44]. In New South Wales, further protective measures include the compulsory use of Australian-standard-compliant half-mask respirators, annual fit testing to ensure proper seal and functionality, and the deployment of vacuum dust extraction systems alongside wet processing methods[45]. Moreover, workplaces must routinely monitor airborne dust levels to ensure compliance with WHS air quality standards[46]. At the national level, SafeWork Australia, the primary agency responsible for occupational safety guidelines, has played a central role in shaping the regulatory framework that supports the engineered stone ban . Its final report, released in 2023, offers updated implementation guidance and outlines the anticipated outcomes of the adopted control measures[47].
2.2 Media framing theory in health policy research
2.2.1 Core concepts of framing theory
Media framing theory offers a conceptual foundation for understanding how public health policies are communicated and interpreted by the public. According to Entman, frames are interpretative structures that enable individuals “to locate, perceive, identify, and label” events or information[21]. With the context of public health policy, framing functions to emphasize particular elements of an issue while downplaying others, thereby shaping how the public perceives, evaluates, and responds to policy initiatives[22].
2.2.2 Framing in occupational health policy
In the discourse surrounding occupational health policy, several dominant frames frequently emerge. These key frames are summarized in Table 1.
Frame Type | Description | Key Elements | Reference |
Health risk frame | Emphasizes immediate and long-term health consequences of workplace exposures | Medical evidence, Personal narratives, Expert testimonies | [48] |
Economic frame | Focuses on financial implications of health policies | Implementation costs, Industry impacts, Employment effects | [49] |
Regulatory frame | Centers on government intervention and control measures | Enforcement mechanisms, Compliance requirements, Policy guidelines | [50] |
Social justice frame | Highlights worker rights and corporate responsibility | Worker protection, Industry accountability, Equity considerations | [51] |
2.2.3 Social media and frame amplification
Social media platforms have significantly reshaped the construction and dissemination of policy frames within public discourse. These digital environments function as interactive arenas where various stakeholders negotiate and contest policy interpretations. Features such as sharing, commenting, and algorithmic amplification facilitate the rapid spread of particular frames while simultaneously diminishing the visibility of competing narratives. This dynamic contributes to the phenomenon of “frame resonance”, where specific policy interpretations gain prominence through network sharing[52].
The interactive nature of social media platforms offers unique opportunities to examine frame effects in real time, enabling researchers to observe how various stakeholders engage with, respond to, and shape policy discourse. In the context of occupational health discussions, this often reveals intricate interactions among health, economic, and social justice frames. However, the echo chamber effect inherent to social media can reinforce particular interpretations within like-minded communities, potentially leading to heightened polarization in public perceptions of policy initiatives, such as Australia’s engineered stone ban.
2.3 Application of deep learning in sentiment analysis
Deep learning techniques have transformed the field of sentiment analysis, offering substantial improvements over traditional methods. Conventional approaches, such as lexicon-based models, often struggle with complex linguistic phenomena, including polysemy, sarcasm, and context-dependent meanings[53]. In contrast, deep learning architectures, such as Convolutional Neural Networks (CNNs), Recurrent Neural Networks (RNNs), and Long Short-Term Memorys (LSTMs) networks—excel at capturing intricate patterns in large-scale text data. These models automatically learn relevant features without the need for manual input, making them particularly well-suited for detecting subtle nuances in public sentiment. In this study, such methods have proven effective in extracting the sentiment dynamics essential to the experimental analysis.
3. Methodology
In recent years, social media has emerged as an increasingly influential platform for public discourse. Platforms such as Twitter (now X), Facebook, Reddit, and YouTube provide dynamic spaces where users engage with current affairs and express their opinions, thereby offering valuable reflections of public attitudes on issues such as health and the environment[25,54]. Numerous studies have demonstrated that natural language processing (NLP) techniques, such as sentiment analysis and topic modeling, are effective tools for identifying trends in public sentiment and uncovering key themes in online discussions. These methods offer critical insights into how the public perceives and responds to specific issues[55]. For example, NLP techniques have been applied to analyze sentiment toward flu vaccination, revealing that “distrust” and “criticism of government policies” were dominant themes[56]. Similarly, another study employed BERTopic to examine discourse surrounding the United Nations Decade of Healthy Ageing campaign, identifying 16 thematic clusters. The findings indicated that the public strongly associates healthy aging with proper diet and lifestyle choices[57]. Together, these studies highlight the significant potential of NLP methods in advancing research at the intersection of health communication and public policy.
For our study, we selected Reddit and YouTube as the primary data sources based on their unique user structures and interaction patterns, which align well with our research objectives. Reddit’s hierarchical organization into subreddits attracts a younger, tech-savvy, and highly engaged audience, fostering in-depth and topic-specific discussions that capture nuanced public sentiments and opinions[58,59]. YouTube, on the other hand, offers a diverse content ecosystem that includes news, expert commentary, and community reactions, providing a rich source of commentary data and capturing both linguistic and non-linguistic cues essential for sentiment analysis[60]. Although other platforms such as Twitter (now X) and Facebook are also important channels for public discourse, Twitter’s brevity and real-time focus may limit the depth of discussion compared to Reddit, while Facebook’s often closed-network structure can constrain the diversity of publicly accessible opinions. Therefore, our targeted approach, focusing on Reddit and YouTube, offers detailed and comprehensive insights. Nevertheless, we acknowledge that excluding other platforms constitutes a limitation that may affect the generalizability of our findings.
Future research should broaden its scope to include a wider range of platforms, such as Twitter and Facebook, and consider integrating survey data or alternative methodologies to capture a more balanced and representative cross-section of public attitudes. Figure 1 presents an overview of the data collection and analysis process employed in this study.
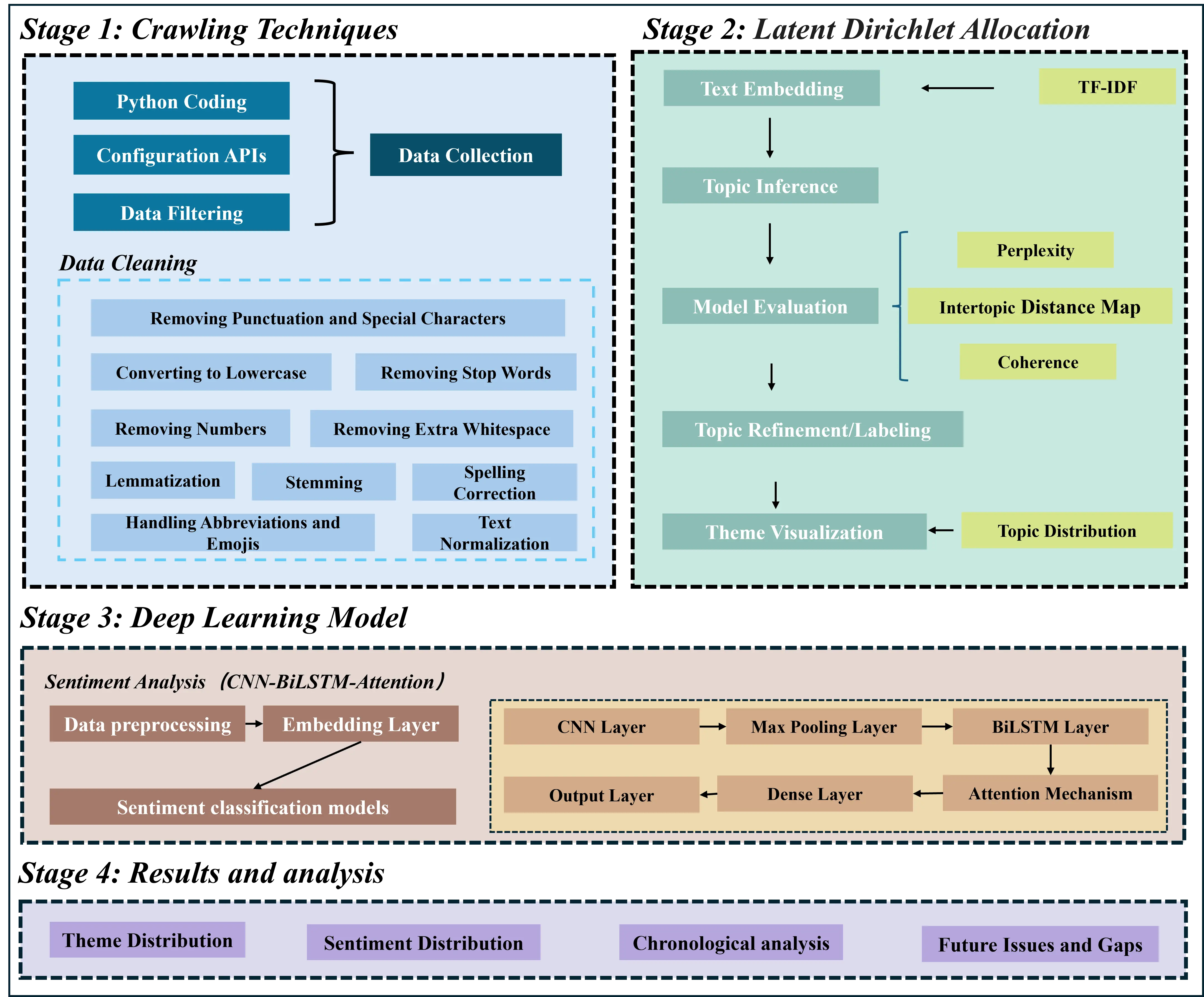
Figure 1. Overview of data processing and analytical methods. TF-IDF: Term Frequency–Inverse Document Frequency; CNN: Convolutional Neural Network; BiLSTM: Bidirectional Long Short-Term Memory.
3.1 Data extraction
Web crawling techniques enable the rapid extraction of large volumes of information from numerous web pages, significantly enhancing the efficiency and scope of data collection. Moreover, these techniques can be flexibly tailored to meet specific data acquisition requirements, offering highly customizable solutions for diverse research needs[61]. In this study, Python was employed as the primary programming language to implement the data extraction process, as detailed below.
1) Configuring APIs: Web crawler libraries such as prew (Python Reddit API Wrapper) were employed to extract data from Reddit, while youtube-dl was used to retrieve video and comment information from YouTube.
2) Data screening: This process involved a keyword-based search, informed by policy documents and framing theory literature, to ensure coverage of different frame types such as health risks, economic impacts, and regulations (Table 2). The search spanned the period from January 2022 to May 2024, a timeframe strategically selected to capture shifts in public attitudes related to the introduction and enforcement of the engineered stone ban. Keyword selection was based on a preliminary analysis of policy texts, media reports, and exploratory data collection, which identified commonly used terms and their variations in public discourse. The ‘most relevant’ sorting algorithm defined by each platform’s API was used to prioritize content closely matching the keywords. An automated filtering mechanism was also incorporated into the data collection pipeline to evaluate the relevance of the retrieved content.
Material Keywords | Policy Keywords | Example Combinations | Frame-specific Keywords |
Engineered stone | Ban | “Engineered stone ban” | Health (“risk”, “disease”) |
Artificial stone | Prohibition | “Artificial stone prohibition” | Economics (“cost”, “jobs”) |
Artificial slab | Forbidden | “Artificial slab restriction” | Safety (“protection”) |
Countertop | Restriction | “Quartz forbidden” | Rights (“worker rights”) |
Quartz | “Countertop ban” |
3) Data collection: The data collected included post titles, introductions, publishing dates, commenting times, and the number of likes on both posts and comments. Due to privacy protection regulations, geolocation information for posters and commenters was not available. During data collection, the “drop_duplicates” method was used to remove duplicate entries, errors = ‘coerce’ was applied to handle erroneous data, and applied the “fillna” function was used to address missing values. A total of 12,152 comments were collected, most of which were short text entries, typically fewer than 100 words.
4) Data preprocessing: Python was used to clean up the collected database, including converting all characters to lowercase, and removing duplicates, spaces, URLs, and special characters (e.g., %, $). The NLTK library was used to split text and remove stop words. Notably, the dataset included various instances of Internet slang and Spanish-language comments, which were manually filtered out to prevent noise and maintain the accuracy of subsequent analysis. After preprocessing, a total of 7,198 valid text entries were retained. A detailed dataset description is provided in Table 3.
Platform | Data Type | Number of Posts | Number of comments before data cleaning | Number of Comments | Time Range | Example |
Post Title | 1,527 | - | - | January 2022 - May 2024 | “Australia’s ban on engineered stone - what are your thoughts?” | |
Post Comment | - | 11,076 | 6,729 | January 2022 - May 2024 | “As someone who works with engineered stone, I’m worried about losing my job. The government should have provided more support for the industry during this transition.” | |
Comment Timestamp | - | - | - | January 2022 - May 2024 | “2023-03-15 09:42:36” | |
YouTube | Video Title | 108 | - | - | January 2022 - May 2024 | “The truth behind Australia’s engineered stone ban.” |
Video Comment | - | 1,076 | 469 | January 2022 - May 2024 | “I don’t understand why they’re banning engineered stone completely. Shouldn't they focus on improving safety measures in the workplace instead?” | |
Comment Timestamp | - | - | - | January 2022 - May 2024 | “2023-02-28 05:15:23” | |
Total | - | 1,635 | 12,152 | 7,198 | - | - |
3.2 Model synthesis
In this study, we utilize two analytical tools—Latent Dirichlet Allocation (LDA) and a Convolutional Neural Network - Bidirectional Long Short-Term Memory - Attention Model (CNN-BiLSTM-Attention model)—to extract and analyze key thematic and sentiment features from social media comments. The primary goal of using LDA is to efficiently identify the underlying topics present in the data, directly addressing our research question regarding public reactions to the engineered stone ban[62-64]. In parallel, the CNN-BiLSTM-Attention model is used exclusively for sentiment analysis, allowing us to classify public opinions as positive, negative, or neutral, thus linking the analytical results directly to the objectives of our study. While the technical specifications of these models are provided in the supplementary material, the main focus in the text is on their application: LDA organizes the data into coherent themes, and the CNN-BiLSTM-Attention model assesses sentiment trends within these themes[65].
3.3 Topic modelling
LDA is one of the most widely used and effective topic modeling algorithms. It is a generative statistical model designed to uncover latent topics within a collection of documents[66]. LDA operates on the assumption that each document is a mixture of multiple topics, and each topic is characterized by a specific distribution of words[67]. By analyzing word frequencies and co-occurrences patterns within the corpus, LDA identifies the underlying thematic structure of the documents. In this study, the Python programming language was selected for its versatility and ease of use, and the Gensim library was chosen for its effectively in handling large-scale text data. Specifically, the “gensim.models.ldamodel.LdaModel” package was employed to implement the LDA model.
3.4 Sentiment analysis
Sentiment analysis is a technique used to detect and classify emotional tendencies within textual content. It is commonly applied to identify opinions and emotional attitudes expressed in text. In recent years, sentiment analysis has become an essential tool for assessing public opinion on social media, particularly in relation to societal issues, policy debates, and environmental concerns, where public engagement has significantly increased[55,67,68]. Mainstream sentiment classification methods can be broadly categorized into binary, ternary, and more complex five-class or six-class systems. Among these, binary classification, distinguishing between positive and negative sentiments, is the simplest and most efficient, often used when rapid insight into user needs, attitudes, or satisfaction is required[69]. However, binary classification lacks the ability to capture more subtle emotional shifts and does not accommodate neutral perspective[70]. Ternary classification addresses this shortcoming by introducing a neutral category alongside positive and negative sentiments. It is particularly well-suited for contexts such as policy debates, social media commentary, and news analysis, where public opinion is frequently nuanced and may reflect uncertainty or a more objective and rational stance[71]. Therefore, ternary classification is considered effective in capturing these emotional distinctions.
For more complex tasks, five-class or six-class classification systems are often preferred. Five-class classification includes categories such as very positive, positive, neutral, negative, and very negative, making it suitable for fine-grained sentiment analysis in contexts such as film or product reviews[72]. This approach allows for a more nuanced distinction between strong dissatisfaction and strong enthusiasm, rather than relying solely on broad positive or negative categories. Six-class classification extends emotional dimensions further by incorporating specific emotional states such as anger, disgust, fear, joy, sadness, and surprise. This level of emotional monitoring is primarily used in fields such as crisis management, customer service, and psychological health assessment, where the detection of complex emotional expressions is critical[73,74]. However, these advanced classification systems are associated with increased model complexity and higher training costs.
In this study, sentiment classification was applied to user-generated discussions on YouTube and Reddit to explore public attitudes toward the engineered stone ban. Considering the research objectives and the nature of the data, ternary classification was deemed the most appropriate approach. This method enables the differentiation of public sentiment into three categories: positive, negative, and neutral. The analysis begins with preprocessing of the textual data, which includes noise reduction and the removal of irrelevant content. The cleaned data is then fed into the sentiment analysis model, where it undergoes encoding and decoding processes. The model subsequently extracts features and classifies the input texts according to the three sentiment categories mentioned above.
3.5 CNN-BiLSTM-attention model
In the field of sentiment analysis, a wide range of deep learning models have been developed, including standalone architectures such as CNN, RNN, and their variants like LSTM networks and Gated Recurrent Unit. However, these individual models often face inherent limitations in capturing complex semantic relationships and long-range contextual dependencies within textual data[75].As a result, an increasing number of studies are exploring hybrid architectures to enhance overall model performance and accuracy[76-79]. The complete architecture of the model is presented in Figure 2.
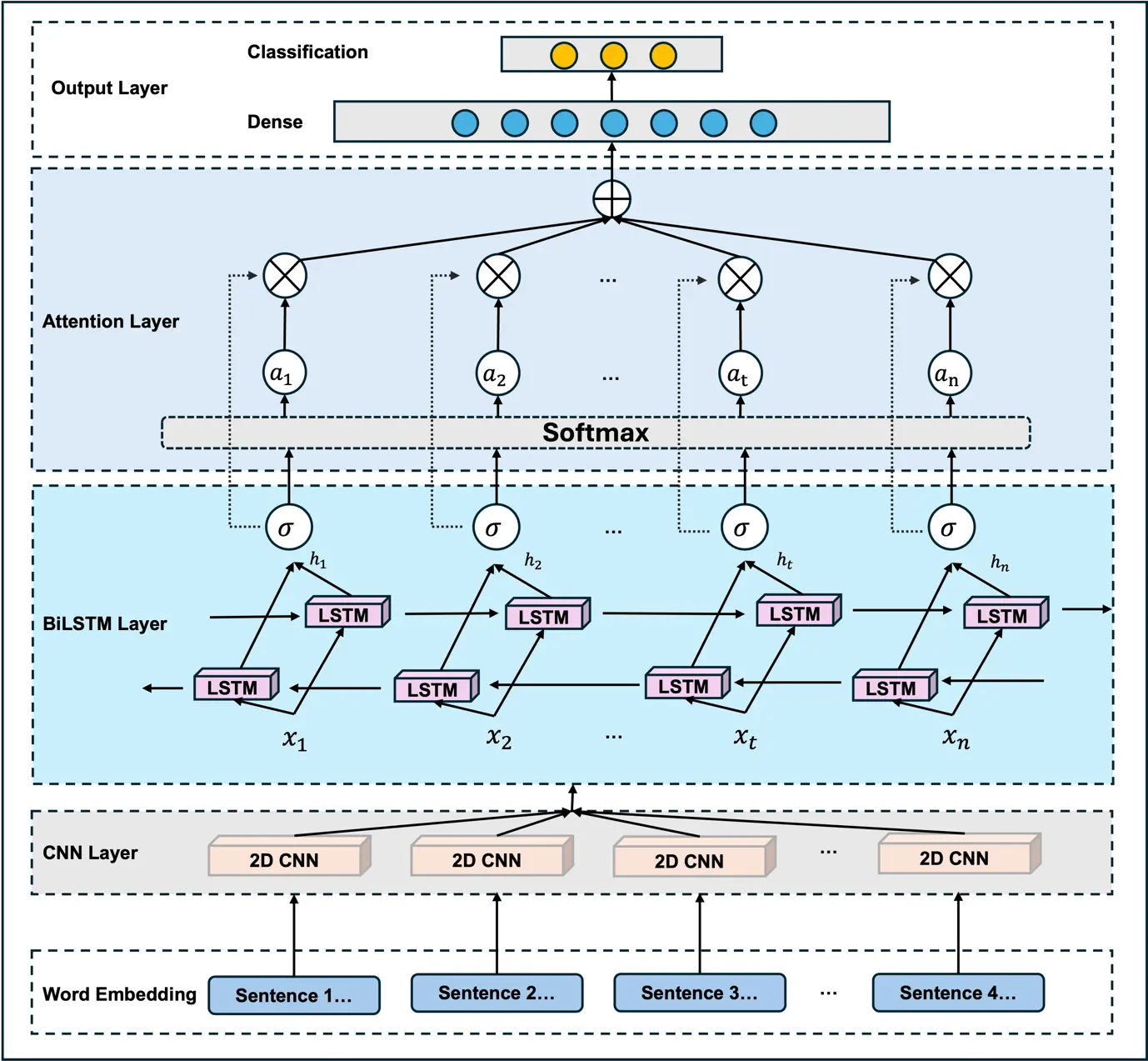
Figure 2. CNN-BiLSTM-attention model architecture. LSTM: Long Short-Term Memory; CNN: Convolutional Neural Network.
4. Results
4.1 Optimal topic number determination
To determine the optimal number of topics for the topic model, this study employed Multidimensional Scaling to evaluate the distribution characteristics among topics and assessed the model’s semantic differentiation capability based on keyword distributions. Figure 3 illustrates the inter-topic distance distribution when the number of topics is set to three and four, reflecting the semantic structural under different topic settings.
In the three-topic model, the topic distribution is relatively balanced, with minimal overlap between topics. This suggests that the model can identify well-separated and clearly clearly defined thematic structures. Moreover, the associated keyword distributions show strong semantic coherence, aligning closely with the core content of each topic, which enhances the interpretability of the model. By contrast, increasing the number of topics to four leads to a noticeable decline in semantic separation, particularly between Topic 1 and Topic 2, where substantial overlap is observed. Additionally, the keyword distributions become more scattered, weakening semantic differentiation and indicating a reduction in topic quality as the number of topics increases.
From the model’s performance, it is evident that while increasing the number of topics may capture more semantic nuances, an excessive number of topics can result inblurred semantic boundaries and even redundant content. The three-topic model strikes an effective balance between semantic coherence and topic distinctiveness, making it the most suitable choice for this study. This finding is consistent with the conclusion of Chen and Wei, who emphasized that selecting an appropriate number of topics helps to prevent overfitting and enhances the interpretability of the model[68].
4.2 Principal topics analysis
Based on the results of the LDA topic model, three principal themes were identified: “Protection and Utilization”, “Government Regulation and Industry Impact”, and “Worker and Population Well-being”. These themes were derived from the dominant keywords associated with each topic. As shown in Table 4, public discourse on social media is primarily focused on “Worker and Population Well-being” (0.487), “Protection and Utilization” (0.266), and “Government Regulation and Industry Impact” (0.246).
Theme | Weights | Keywords |
Protection and utilization | 0.266 | Dust, mask, wear, years, kitchen, bench, cutting, use, concrete, time, day, good, masks, work, wearing, back, PPE |
Government regulation and industry impact | 0.246 | People, good, government, OSHA, right, companies, employees, years, many, employers, someone, even, job |
Worker and population well-being | 0.487 | Stone, silica, economics, ban, cutting, safe, work, asbestos, workers, safety, alternative, product, use, natural, risk |
PPE: Personal Protective Equipment; OSHA: Occupational Safety and Health Administration.
In the “Protection and Utilization” theme, discussions primarily center on safety practices and the use of engineered stone, particularly in operations involving materials such as kitchen countertops and concrete. Social media users emphasize the necessity of wearing masks and personal protective equipment (PPE), safety precautions during cutting processes, and protective measures required for prolonged working hours. The “Government Regulation and Industry Impact” theme highlights the proactive role of government and regulatory bodies, such as the Occupational Safety and Health Administration (OSHA), in safeguarding worker health. Comments frequently reference regulatory policies, corporate accountability, and employee rights. Nevertheless, many users express concerns that the implementation of these regulations may pose challenges to industrial transformation and employment, potentially affecting the economic stability of both businesses and workers.
The most prominent theme, “Worker and Population Well-being”, captures widespread concerns regarding the health risks posed by stone materials, particularly engineered stone containing silica. Discussions focus on the adverse health effects of dust and hazardous substances generated during stone cutting, especially for workers. Users also express apprehensions about the potential bans on engineered stone and safety issues related to associated products. Additionally, many commenters highlight the economic consequences of such bans and explore the feasibility of adopting natural alternatives, reflecting broader concerns about the potential impact on industry and employment.
4.3 Trend of the topics over time
Figure 4 illustrates both the overall posting trends and the temporal evolution of the thematic categories identified through our topic modeling analysis. The visualization reveals distinct patterns, highlighting how different themes gained prominence in public discourse at various stages over time.
In early 2023, public discussions predominantly focused on health risks, with a notable peak occurring in February, during which health-related concerns constituted approximately 21.3% of the dialogue. This surge in attention coincided with the initial debates surrounding the proposed ban and growing awareness of silicosis cases among workers in the engineered stone industry.
The most significant surge in discussion volume occurred in December 2023, with economic impact emerging as the dominant theme, accounting for approximately 55.5% of posts. This dramatic increase corresponded with the government’s formal announcement of the ban, which triggered intense public debate regarding the costs of industry adaptation and concerns over job security. During this period, the total number of monthly posts exceeded 3,200, the highest recorded throughout the study period.
In early 2024, the discourse shifted toward regulatory compliance, accounting for approximately 23.1% of the discussions. This transition reflected businesses’ increasing focus on the practical challenges of implementation as they prepared for the July 2024 enforcement deadline. Although the overall discussion volume moderated after January 2024, attention remained centered on compliance issues and industry adaptation strategies.
The temporal analysis highlights how public discourse evolved in response to key policy developments, transitioning from initial health concerns to economic impacts and finally to implementation challenges. This progression illustrates the dynamic nature of public reactions to major policy shifts and underscores the critical role of timing in policy communication strategies. The findings also indicate that while economic concerns dominated the overall discourse, specific events and official announcements significantly shaped the relative salience of each theme over time.
4.4 Results from public sentiments
To evaluate public sentiment regarding the engineered stone ban, we analyzed 7,198 comments collected from Reddit and YouTube using the trained CNN-BiLSTM-Attention model. As presented in Table 5, the majority of comments (3,998, or 55.5%) conveyed negative sentiments, significantly exceeding those expressing positive (1,540, or 21.3%) or neutral (1,660, or 23.1%) views. This sentiment distribution indicates substantial public opposition and resistance to Australia’s implementation of the engineered stone ban.
Sentiment | Number of Comments | Percentage | Example |
Positive | 1,540 | 21.30% | “Banning engineered stone is definitely great news! Finally, there's some real focus on worker health. Working with harmful dust every day is literally risking your life.” |
Neutral | 1,660 | 23.10% | “Engineered stones are often up to 90% silica. It's the concentration that is the issue here. Most other commonly cut and ground silica-containing products are nowhere near this level.” |
Negative | 3,998 | 55.50% | “The real issue to be addressed is unsafe work practices. Every stone product contains silica, including cement, concrete, tiles, bricks, and even drywall. I have seen on many occasions people with no mask protection, covered in dust from cutting, grinding, and sanding concrete, tiles, and drywall, even when masks were provided by the company. State and federal workplace ministers had the opportunity to address these unsafe work practices around working with all these products that produce dangerous dust but chose not to. So, people will still be dying from dust-related lung diseases until they address the real issue, which is unsafe work practices.” |
Total | 7,198 | 100.00% |
The prevalent negative sentiment likely reflects widespread concerns about working conditions, safety standards, and dissatisfaction with both corporate and government regulations, particularly in relation to worker health and safety. The introduction of the ban appears to have intensified longstanding issues within the engineered stone industry, contributing to a surge in critical public sentiment. Although a segment of the public supports the ban, as evidenced by the proportion of positive comments, these voices remain in the minority. Meanwhile, the substantial share of neutral comments suggests that a notable portion of the public remains undecided or ambivalent about the ban’s overall impact.
However, a closer examination of the negative comments reveals that many are not purely expressions of opposition to the ban, but rather reflect calls for stricter enforcement and additional regulatory measures. For instance, some commenters acknowledged that while the ban represents a positive step forward, the current level of enforcement has a greater impact on the prospects of the industry and more appropriate measures are needed.
The identified themes encapsulate distinct yet interconnected dimensions of the engineered stone ban’s implications. The theme “Worker and Population Well-being” highlights the health risks associated with engineered stone, particularly exposure to respirable crystalline silica and its established link to occupational diseases such as silicosis. This directly corresponds to the health dimension of the study, reinforcing the rationale behind the ban as a measure to protect worker health and mitigate occupational hazards. The predominance of negative sentiment within this theme reflects public concern over systemic shortcomings in workplace safety and the perceived inadequacy of regulatory enforcement, as illustrated by comments such as “unsafe work practices remain the real issue”.
In contrast, the theme of “Government Regulation and Industry Impact” reflects public discourse surrounding the regulatory framework and economic consequences of the policy. While some comments acknowledge the necessity of the ban, many express dissatisfaction with its perceived lack of foresight and the financial burden it imposes on affected industries. Notably, a substantial portion of negative commentary highlights the financial strain on small businesses and criticizes the limited government support provided to assist with the transition. This theme is closely aligned with the policy dimension of the study, underscoring public concerns about the challenge of balancing regulatory effectiveness with economic stability.
Finally, the theme of “Protection and Utilization” encompasses discussions on sustainable practices, resource management, and the environmental consequences associated with the use and disposal of engineered stone. This theme aligns with the environmental dimension of the study, emphasizing the importance of developing practical solutions that mitigate ecological harm while promoting the safe and responsible utilization of materials.
5. Discussion
5.1 Methodological effectiveness and model performance
The CNN-BiLSTM-Attention model employed in this study demonstrated notable effectiveness in analyzing social media discourse surrounding the engineered stone ban. The experimental results revealed that the model achieved an accuracy of 0.83, outperforming standalone CNN (0.72) or BiLSTM (0.79) models.
5.2 Frame analysis and public response
Our findings illustrate how different media frames shaped the public discourse surrounding Australia’s engineered stone ban. The prevalence of negative sentiment (55.5%) was largely driven by frames emphasizing economic impacts, particularly concerns about industry costs and job losses. This aligns with framing theory, which suggests that economic consequence frames can have a strong influence on public reception of policy initiatives[80]. In contrast, positive sentiments (21.3%) were primarily linked to health risk frames, which highlighted concerns about worker safety and the long-term health benefits of the ban.
It is important to note that negative sentiments should not be broadly interpreted as opposition to the engineered stone ban. In certain instances, these negative reflect a call for stronger regulatory measures rather than disapproval of the ban itself. This nuanced perspective highlights the need to consider public opinion within a wider socio-political context. Indeed, criticism of the current policy implementation can be viewed as a demand for more support for the stakeholders affected by the ban.
The interaction between these competing frames offers valuable insights for policy communication. While health risk frames generated support for the ban, their effectiveness was often diminished when economic impact frames dominated the conversation. This indicates that the successful implementation of policy may require a more balanced integration of both health and economic considerations in public communication.
5.3 Broader implications and policy recommendations
The public’s concerns extend beyond the engineered stone ban, with many that it alone is insufficient to fully address the risks associated with silica dust exposure. Other commonly used construction materials, such as concrete and bricks, also contain high levels of silica[81,82]. While a blanket ban on all silica-containing materials is unrealistic due to their widespread use, ensuring worker safety will require more comprehensive regulatory measures. The government could enhance inspections, introduce real-time dust monitoring tools, promote worker health assessments, and utilize big data to identify high-risk occupations and regions.
The economic impact of the ban has been a central point of public discussion, especially within the construction industry. The transition to alternative materials, adjustments in production processes, and the need for equipment upgrades and employee retraining will increase operating costs and construction lead times. Potential material shortages or delayed deliveries may further hinder industry productivity and disrupt project schedules[83]. These economic pressures have contributed to the public’s negative perception of the ban. To mitigate these concerns, the government could provide financial support and subsidies to affected businesses, promote innovation, and facilitate the transition to safer alternative materials. Additionally, offering retraining programs for affected workers or helping them find employment in industries with technical overlaps could safeguard their job prospects.
6. Conclusions
Australia’s ban on engineered stone represents a globally pioneering policy initiative; however, no prior research has specifically examined public attitudes and the social impacts of this prohibition. This study addresses several critical research gaps by leveraging natural language processing techniques, namely, LDA for topic modeling and a CNN-BiLSTM-Attention model for sentiment analysis—to analyze the complex public sentiment surrounding Australia’s comprehensive ban on engineered stone, driven by health concerns related to high silica content.
Guided by framing theory, our analysis of 7,198 comments from Reddit and YouTube identified three principal themes: protection and utilization, government regulation and industry impact, and worker welfare. Sentiment analysis revealed a predominance of negative sentiment, comprising 55.5% of all comments, largely centered on economic concerns and dissatisfaction with regulatory implementation. Positive sentiment accounted for 21.3%, primarily highlighting the policy’s potential benefits for worker health and safety, while neutral sentiment made up 23.1%, reflecting ambivalence regarding on the ban’s broader implications. This distribution suggests that public discourse was predominantly framed around economic and regulatory lenses, with relatively less emphasis placed on the intended health benefits of the ban.
To improve public understanding and reduce resistance, this study recommends that future policies prioritize transparent communication about the health risks of silica exposure. Additionally, offering economic support to industries affected by such regulations may help address public concerns and facilitate smoother policy adoption. Overall, the findings underscores the importance of a comprehensive policy strategy that integrates health, economic, and social considerations to foster greater public support and compliance.
Acknowledgments
The authors acknowledge the institutional support provided by The University of Adelaide. During the preparation of this work, the authors used ChatGPT to improve the readability and language of the manuscript. After using this tool, the authors reviewed and edited the content as needed and took full responsibility for the content of the published article.
Authors contribution
Sheng Y: Conceptualization, methodology, data curation, formal analysis, writing-original draft.
Oteng D: Software, validation, investigation, writing-review & editing.
Rodrigo N: Data curation, resources, visualization.
Chang R: Methodology, formal analysis, writing-review & editing.
Han J: Software, validation, project administration.
Bi P: Supervision, writing-review & editing.
Zuo J: Conceptualization, supervision, project administration, writing-review & editing.
Conflicts of interest
The authors declare no conflicts of interest.
Ethical approval
Not applicable.
Consent to participate
Not applicable.
Consent for publication
Not applicable.
Availability of data and materials
Not applicable.
Funding
None.
Copyright
© The Author(s) 2025.
References
-
1. Alqadi SB, Alamleh D, Naser Eldin, Naser Eldin. A comparative life cycle energy and green house emissions of natural and artificial stone-manufacturing phase. Results Eng. 2023;18:101055.
[DOI] -
2. Frankel A, Blake L, Yates D. LATE-BREAKING ABSTRACT: Complicated silicosis in an Australian worker from cutting engineered stone countertops: An embarrassing first for Australia. Eur Respir J. 2015;46:PA1144.
[DOI] -
3. Hoy RF, Baird T, Hammerschlag G, Hart D, Johnson AR, King P, et al. Artificial stone-associated silicosis: a rapidly emerging occupational lung disease. Occup Environ Med. 2018;75(1):3-5.
[DOI] -
4. Ophir N, Shai AB, Alkalay Y, Israeli S, Korenstein R, Kramer MR, et al. Artificial stone dust-induced functional and inflammatory abnormalities in exposed workers monitored quantitatively by biometrics. ERJ Open Res. 2016;2(1):00086.
[DOI] -
5. Cooper JH, Johnson DL, Phillips ML. Respirable silica dust suppression during artificial stone countertop cutting. Ann Occup Hyg. 2015;59(1):122-126.
[DOI] -
6. Johnson DL, Phillips ML, Qi C, Van AT, Hawley DA. Experimental evaluation of respirable dust and crystalline silica controls during simulated performance of stone countertop fabrication tasks with powered hand tools. Ann Work Expo Health. 2017;61(6):711-723.
[DOI] -
7. Madl AK, Donovan EP, Gaffney SH, McKinley MA, Moody EC, Henshaw JL. State-of-the-science review of the occupational health hazards of crystalline silica in abrasive blasting operations and related requirements for respiratory protection. J Toxicol Environ Health B. 2008;11(7):548-608.
[DOI] -
8. Requena-Mullor M, Alarcón-Rodríguez R, Parrón-Carreño T, Martínez-López JJ, Lozano-Paniagua D, Hernández AF. Association between crystalline silica dust exposure and silicosis development in artificial stone workers. Int J Environ Res Public Health. 2021;18(11):5625.
[DOI] -
9. Weller M, Clemence D, Lau A, Rawlings M, Robertson A, Sankaran B. An assessment of worker exposure to respirable dust and crystalline silica in workshops fabricating engineered stone. Ann Work Expo Health. 2024;68(2):170-179.
[DOI] -
10. Carrieri M, Guzzardo C, Farcas D, Cena LG. Characterization of silica exposure during manufacturing of artificial stone countertops. Int J Environ Res Public Health. 2020;17(12):4489.
[DOI] -
11. Salamon F, Martinelli A, Vianello L, Bizzottoc R, Gottardo O, Guarnieri G, et al. Occupational exposure to crystalline silica in artificial stone processing. J Occup Environ Hyg. 2021;18(12):547-554.
[DOI] -
12. Seneviratne M, Shankar K, Cantrell P, Nand A. Respirable silica dust exposure of migrant workers informing regulatory intervention in engineered stone fabrication. Saf Health Work. 2024;15(1):96-101.
[DOI] -
13. Safe Work Australia. Decision Regulation Impact Statement: Prohibition on the use of engineered stone [Internet]. Canberra: Safe Work Australia [cited 2024 Jul 25]. Available from: https://www.safeworkaustralia.gov.au/doc/decision-regulation-impact-statement-prohibition-use-engineered-stone
-
14. European Union. Directive (EU) 2017/2398 of the European Parliament and of the Council of 12 December 2017 amending Directive 2004/37/EC on the protection of workers from the risks related to exposure to carcinogens or mutagens at work [Internet]. Brussels: Official Journal of the European Union; 2017 [cited 2024 Jul 25]. Available from: https://eur-lex.europa.eu/eli/dir/2017/2398/oj
-
15. Hua JH, Rose CS, Redlich CA. Engineered stone–associated silicosis—a lethal variant of an ancient disease. JAMA Intern Med. 2023;183(9):908-910.
[DOI] -
16. Ramkissoon C, Gaskin S, Song Y, Pisaniello D, Zosky GR. From engineered stone slab to silicosis: A synthesis of exposure science and medical evidence. Int J Environ Res Public Health. 2024;21(6):683.
[DOI] -
17. Cavalin C, Menéndez-Navarro A, León-Jiménez A, Lecureur V, Lescoat A. The ban on engineered stone in Australia: a milestone in the fight against emerging silica hazards. Eur Respir J. 2024;63(6):2400138.
[DOI] -
18. Perret JL, Miles S, Brims F, Newbigin K, Davidson M, Jersmann H, et al. Respiratory surveillance for coal mine dust and artificial stone exposed workers in Australia and New Zealand: a position statement from the Thoracic Society of Australia and New Zealand. Respirology. 2020;25(11):1193-1202.
[DOI] -
19. Turner MT, Samuel SR, Silverstone EJ, Yates DH. Silica exposure and connective tissue disease: an underrecognized association in three Australian artificial stone workers. Am J Respir Crit Care Med. 2020;201(3):378-380.
[DOI] -
20. Wise J. Doctors call for ban on cutting artificial stone after reporting first UK cases of silicosis. BMJ. 2024. 386:q1755;
[DOI] -
21. Entman RM.. J Commun 1993;
[DOI] -
22. Scheufele DA, Iyengar S. The state of framing research: A call for new directions. In: Kenski K, Jamieson KH, editors. The Oxford Handbook of Political Communication. United Kingdom: Oxford University Press; 2017. p. 619-632.
[DOI] -
23. Diepeveen S, Ling T, Suhrcke M, Roland M, Marteau TM. Public acceptability of government intervention to change health-related behaviours: a systematic review and narrative synthesis. BMC Public Health. 2013;13:756.
[DOI] -
24. Drews S, van den Bergh JCJM. What explains public support for climate policies? A review of empirical and experimental studies. Clim Policy. 2015;16(7):855-876.
[DOI] -
25. Golder S, Scantlebury A, Christmas H. Understanding public attitudes toward researchers using social media for detecting and monitoring adverse events data: multi methods study. J Med Internet Res. 2019;21(8):e7081.
[DOI] -
26. Li Y, Wang S, Tang JHCG, Peng Z, Zhuge C. Public attention and attitudes towards bike-sharing in China: A text mining approach. Transp Res Part D: Transp Environ. 2024;134:104348.
[DOI] -
27. Lian Y, Tang H, Xiang M, Dong X. Public attitudes and sentiments toward ChatGPT in China: A text mining analysis based on social media. Technol Soc. 2024;76:102442.
[DOI] -
28. Baramaleki S, Mahdavian L. Investigation of mechano-chemical properties of the effects of nanoparticles in artificial stone produced. Silicon. 2019;11:1071-1082.
[DOI] -
29. Barreto GNS, de C. Lírio JLD, Gomes MLPM, Carvalho EAS, Lopera HAC, Vieira CMF. Manufacturing epoxy and polyurethane-based artificial stones from waste glass of colorless beverage packaging. Mater Res. 2023;26:e20230002.
[DOI] -
30. Barreto GNS, Carvalho EAS, Souza VS, Gomes MLPM, de Azevedo, Monteiro SN, et al. Engineered stone produced with glass packaging waste, quartz powder, and epoxy resin. Sustainability. 2022;14(12):7227.
[DOI] -
31. Carvalho EAS, Marques VR, Rodrigues RJS, Ribeiro CEG, Monteiro SN, Vieira CMF. Development of epoxy matrix artificial stone incorporated with sintering residue from steelmaking industry. Mater Res. 2015;18:235-239.
[DOI] -
32. Duan D, Liao H, Song H, Cheng F. Hydration mechanism of solid waste-based artificial stone prepared by hot pressing. Constr Build Mater. 2022;357:129317.
[DOI] -
33. Ribeiro CEG, Rodriguez RJS, Vieira CMF. Chemical resistance of ornamental compound stone produced with marble waste and unsaturated polyester. In: Carpenter JS, Bai C, Escobedo JP, Hwang JH, Ikhmayies S, Li B, Li J, Monteiro SN, Peng Z, Zhang M, editors. Characterization of Minerals, Metals, and Materials 2015. Cham: Springer; 2015. p. 283-289.
[DOI] -
34. Cunha Demartini, Rodríguez RJS, Silva FS. Physical and mechanical evaluation of artificial marble produced with dolomitic marble residue processed by diamond-plated bladed gang-saws. J Mater Res Technol. 2018;7(3):308-313.
[DOI] -
35. El-Bashir SM, Binhussain MA, Al-Thumairi NA, AlZayed N. Preparation and characterization of PMMA/stone waste nanocomposites for marmoreal artificial stone industry. J Reinf Plast Compos. 2013;33(4):350-357.
[DOI] -
36. Huang Y, Ma L, Lai W, Mo Q, Zheng Y, Li Y, et al. Mechanical properties improving and microstructure characterization of inorganic artificial stone binder. Sci Eng Compos Mater. 2022;29(1):335-345.
[DOI] -
37. Khaidarov BB, Suvorov DS, Lysov DV, Luchnikova GG, Druzhinina ME, Kuznetsov DV, et al. Investigation of mineral hydraulic binders based on the slag-cement system obtained with the use of vortex electromagnetic homogenization. Refract Ind Ceram. 2021;62:103-107.
[DOI] -
38. Nidheesh PV, Kumar MS. An overview of environmental sustainability in cement and steel production. J Clean Prod. 2019;231:856-871.
[DOI] -
39. Seelen LMS, Teurlincx S, Bruinsma J, Huijsmans TMF, van Donk, Lürling M, et al. The value of novel ecosystems: Disclosing the ecological quality of quarry lakes. Sci Total Environ. 2021;769:144294.
[DOI] -
40. Vandana M, John SE, Maya K, Padmalal D. Environmental impact of quarrying of building stones and laterite blocks: a comparative study of two river basins in Southern Western Ghats, India. Environ Earth Sci. 2020;79:366.
[DOI] -
41. Mulligan S, Hatton PV, Martin N. Resin-based composite materials: elution and pollution. Br Dent J. 2022;232:644-652.
[DOI] -
42. Safe Work Australia [Internet]. Canberra: Safe Work Australia. Prohibition on the use of engineered stone; [about 2 screens]. Available from: https://consult.swa.gov.au/prohibition-on-the-use-of-engineered-stone
-
43. Queensland Government. Managing respirable crystalline silica dust exposure in the stone benchtop industry: Code of Practice 2019 [Internet]. Brisbane: Queensland Government; 2019. Available from: https://os-data-2.s3-ap-southeast-2.amazonaws.com/electrotraining-com-au/bundle37/managing-respirable-crystalline-silica-dust-exposure-in-the-stone-benchtop-industry-code-of-practice-2019.pdf
-
44. Safe Work Australia [Internet]. Canberra: Safe Work Australia. Crystalline silica and silicosis; [about 2 screens]. Available from: https://www.safeworkaustralia.gov.au/safety-topic/hazards/crystalline-silica-and-silicosis
-
45. Safe Work Australia. Code of practice – Managing the risks of respirable crystalline silica from engineered stone in the workplace [Internet]. Canberra: Safe Work Australia; 2022. Available from: http://safework.nsw.gov.au/__data/assets/pdf_file/0005/1042367/managing-the-risk-of-silica-from-engineered-stone-in-the-workplace-COP.pdf
-
46. Safe Work Australia. Health monitoring guidance – crystalline silica [Internet]. Canberra: Safe Work Australia. Available from: https://www.safeworkaustralia.gov.au/sites/default/files/2021-09/health-monitoring-guidance-crystalline-silica.pdf
-
47. Cavalin C, Menéndez-Navarro A, Lescoat A. The banning of engineered stone in Australia: An evidence-based and precautionary policy. Int J Soc Determ Health Health Serv. 2024;
[DOI] -
48. Temmann LJ, Wiedicke A, Schaller S, Scherr S, Reifegerste D. A systematic review of responsibility frames and their effects in the health context. J Health Commun. 2021;26(12):828-838.
[DOI] -
49. Rowbotham S, McKinnon M, Marks L, Hawe P. Research on media framing of public policies to prevent chronic disease: A narrative synthesis. Soc Sci Med. 2019;237:112428.
[DOI] -
50. Gunningham N. Environment law, regulation and governance: Shifting architectures. J Environ Law. 2009;21(2):179-212.
[DOI] -
51. Dutta-Bergman MJ. Theory and practice in health communication campaigns: A critical interrogation. Health Commun. 2005;18(2):103-122.
[DOI] -
52. Dodge J, Metze T. Approaches to policy framing: deepening a conversation across perspectives. Policy Sci. 2024;57:221-256.
[DOI] -
53. Benamara F, Taboada M, Mathieu Y. Evaluative language beyond bags of words: Linguistic insights and computational applications. Comput Linguist. 2017;43(1):201-264.
[DOI] -
54. Hiaeshutter-Rice D, Hawkins I. The language of extremism on social media: An examination of posts, comments, and themes on Reddit. Front Polit Sci. 2022;4:805008.
[DOI] -
55. Boon-Itt S, Skunkan Y. Public perception of the COVID-19 pandemic on Twitter: sentiment analysis and topic modeling study. JMIR Public Health Surveill. 2020;6(4):e21978.
[DOI] -
56. Ng QX, Lee DYX, Ng CX, Yau CE, Lim YL, Liew TM. Examining the negative sentiments related to influenza vaccination from 2017 to 2022: An unsupervised deep learning analysis of 261,613 Twitter posts. Vaccines. 2023;11(6):1018.
[DOI] -
57. Ng QX, Lee DYX, Yau CE, Lim YL, Liew TM. Public perception on ‘healthy ageing’ in the past decade: An unsupervised machine learning of 63,809 Twitter posts. Heliyon. 2023;9(2):e13118.
[DOI] -
58. Achuthan K, Khobragade S, Kowalski R. Public sentiment and engagement on cybersecurity: Insights from Reddit discussions. Comput Hum Behav Rep. 2025;17:100573.
[DOI] -
59. Ghosh I, Alfaro-Cortés E, Gámez M, García-Rubio N. Reflections of public perception of Russia-Ukraine conflict and Metaverse on the financial outlook of Metaverse coins: Fresh evidence from Reddit sentiment analysis. Int Rev Financ Anal. 2024;93:103215.
[DOI] -
60. Burgess J, Green J, Rebane G. Agency and controversy in the YouTube community. In: Friese H, Nolden M, Rebane G, Schreiter M, editors. Handbuch Soziale Praktiken und Digitale Alltagswelten. Wiesbaden: Springer; 2020. p. 105-116.
[DOI] -
61. Ferrara E, De Meo, Fiumara G, Baumgartner R. Web data extraction, applications and techniques: A survey. Knowl-Based Syst. 2014;70:301-323.
[DOI] -
62. Henderi H, Hayadi BH, Sofiana S, Padeli P, Setiyadi D. Unsupervised learning methods for topic extraction and modeling in large-scale text corpora using LSA and LDA. J Appl Data Sci. 2023;4(3):103-118.
[DOI] -
63. Wang B, Wang Z. Heterogeneity evaluation of China’s provincial energy technology based on large-scale technical text data mining. J Clean Prod. 2018;
[DOI] -
64. Zhu B, Zheng X, Liu H, Li J, Wang P. Analysis of spatiotemporal characteristics of big data on social media sentiment with COVID-19 epidemic topics. Chaos Solit Fractals. 2020;140:110123.
[DOI] -
65. Sun F, Chu N. Text sentiment analysis based on CNN-BiLSTM-attention model. In: 2020 International Conference on Robots & Intelligent System (ICRIS); 2020 Nov 7-8; Sanya, China. New York: IEEE; 2020. p. 749-752.
[DOI] -
66. Tetteh MO, Boateng EB, Darko A, Chan APC. What are the general public’s needs, concerns and views about energy efficiency retrofitting of existing building stock? A sentiment analysis of social media data. Energy Build. 2023;301:113721.
[DOI] -
67. Liu M, Luo X, Lu WZ. Public perceptions of environmental, social, and governance (ESG) based on social media data: Evidence from China. J Clean Prod. 2023;387:135840.
[DOI] -
68. Chen K, Wei G. Public sentiment analysis on urban regeneration: A massive data study based on sentiment knowledge enhanced pre-training and latent Dirichlet allocation. PLOS One. 2023;18(4):e0285175.
[DOI] -
69. Ahmeda RAED, Shehaba ME, Morsya S, Mekawiea N. Performance study of classification algorithms for consumer online shopping attitudes and behavior using data mining. In: 2015 Fifth International Conference on Communication Systems and Network Technologies; 2015 Apr 4-6; Gwalior, India. New York: IEEE; 2015. p. 1344-1349.
[DOI] -
70. Sharma NA, Ali ABMS, Kabir MA. A review of sentiment analysis: tasks, applications, and deep learning techniques. Int J Data Sci Anal. 2025;19:351-388.
[DOI] -
71. Chakraborty P, Sharma A. Public opinion analysis of the transportation policy using social media data: a case study on the Delhi odd-even policy. Transp Dev Econ. 2019;5:5.
[DOI] -
72. Peng J, Wu T, Zhang W, Cheng F, Tan S, Yi F, et al. A fine-grained modal label-based multi-stage network for multimodal sentiment analysis. Expert Syst Appl. 2023;221:119721.
[DOI] -
73. Balan O, Moise G, Petrescu L, Moldoveanu A, Leordeanu M, Moldoveanu F. Emotion classification based on biophysical signals and machine learning techniques. Symmetry. 2020;12(1):21.
[DOI] -
74. Wen W, Liu G, Cheng N, Wei J, Shangguan P, Huang W. Emotion recognition based on multi-variant correlation of physiological signals. IEEE Trans Affect Comput. 2014;5(2):126-140.
[DOI] -
75. Yadav A, Vishwakarma DK. Sentiment analysis using deep learning architectures: a review. Artif Intell Rev. 2020;53:4335-4385.
[DOI] -
76. Cai R, Qin B, Chen Y, Zhang L, Yang R, Chen S. Sentiment analysis about investors and consumers in energy market based on BERT-BiLSTM. IEEE Access. 2020;8:171408-171415.
[DOI] -
77. Ombabi AH, Ouarda W, Alimi AM. Deep learning CNN-LSTM framework for Arabic sentiment analysis using textual information shared in social networks. Soc Netw Anal Min. 2020;10:1-13.
[DOI] -
78. Tan KL, Lee CP, Anbananthen KSM, Lim KM. RoBERTa-LSTM: a hybrid model for sentiment analysis with transformer and recurrent neural network. IEEE Access. 2022;10:21517-21525.
[DOI] -
79. Wei J, Liao J, Yang Z, Wang S, Zhao Q. BiLSTM with multi-polarity orthogonal attention for implicit sentiment analysis. Neurocomputing. 2020;383:165-173.
[DOI] -
80. Menz G. Framing the matter differently: the political dynamics of European Union labour migration policymaking. Camb Rev Int Aff. 2015;28(4):554-570.
[DOI] -
81. Rey-Brandariz J, Martínez C, Candal-Pedreira C, Pérez-Ríos M, Varela-Lema L, Ruano-Ravina A. Occupational exposure to respirable crystalline silica and lung cancer: a systematic review of cut-off points. Environ Health. 2023;22:82.
[DOI] -
82. Xiao P, Li Y. Dose response assessment of silica exposure and poisoning of construction workers. Environ Pollut Bioavailab. 2023;35:2190489.
[DOI] -
83. Katsaliaki K, Galetsi P, Kumar S. Supply chain disruptions and resilience: a major review and future research agenda. Ann Oper Res. 2022;319:965-1002.
[DOI]
Copyright
© The Author(s) 2025. This is an Open Access article licensed under a Creative Commons Attribution 4.0 International License (https://creativecommons.org/licenses/by/4.0/), which permits unrestricted use, sharing, adaptation, distribution and reproduction in any medium or format, for any purpose, even commercially, as long as you give appropriate credit to the original author(s) and the source, provide a link to the Creative Commons license, and indicate if changes were made.
Publisher’s Note
Share And Cite