Abstract
The architecture, engineering and construction (AEC) industry is currently encountering numerous challenges and actively pursuing industrial upgrades through digital transformation. Digital twin (DT) technology aligns well with the industry's evolving needs due to its capabilities in data integration, intelligent decision-making, and effective management of project progress, efficiency, and quality. This study conducts a comprehensive literature review to analyze the strengths, weaknesses, opportunities, and threats (SWOT) associated with DT applications in the AEC sector. Additionally, field visits and semi-structured interviews were conducted on a selected construction project where DT was implemented, allowing for an in-depth examination of both its significant benefits and potential limitations. To further evaluate and prioritize the identified SWOT factors, a SWOT-AHP analysis was performed. The results indicate that the AEC industry has a strong interest in DT's advantages, particularly its potential to enhance resource allocation and process efficiency. Furthermore, the analysis reveals that the strategic quadrilateral occupies the largest area in the fourth quadrant (0.8554), with the strategic center of gravity located at (0.2321, -0.04135), suggesting that Strengths-Threats (ST) strategies should be implemented to support the future development and adoption of DT. Based on this strategic framework, the study formulates actionable development strategies to facilitate the effective integration and widespread adoption of DT in the AEC industry.
Keywords
1. Introduction
The architecture, engineering, and construction (AEC) industry is undergoing significant transformation due to the rapid proliferation of smart technologies and digital tools that are reshaping construction practices[1]. However, despite these advancements, the AEC industry is still often perceived as a traditional, labor-intensive sector. This conventional nature is further exacerbated by challenges such as significant energy consumption and outdated management practices. As a result, the AEC industry faces both new opportunities and significant challenges in the wake of technological transformation. Notably, the AEC industry suffers from limited information flow, a low degree of intelligence, and a lack of production innovation, all of which hinder its progress[2]. Achieving sustainable development necessitates innovative technological solutions[3], making it imperative for the AEC industry to adopt new digital strategies to enable greener, more efficient, and safer practices[4]. While the industry is actively exploring new information technologies, tools such as Computer-Aided Design (CAD) and Building Information Modeling (BIM) still face limitations, including insufficient information integration and coordination challenges[5].
DT offers an imaginative solution to the challenges currently faced by the AEC industry. Originally introduced by NASA in the 1960s as part of the Apollo program[6]. DT was later adopted by the US Air Force Research Laboratory for military applications. It supports functionalities such as real-time remote monitoring, simulation, and predictive maintenance[7], enabling the U.S. Air Force address aircraft maintenance and life prediction issues in complex operational environments. With advancements in technology policies, DT was converted from military to civilian use. It facilitates product-specific digital energy measurement during both production and usage phases, providing feedback for sustainable product development and optimized monitoring[8]. At the Hannover Messe, Germany proposed the "Industry 4.0" concept, which incorporates DT and emphasizes its role in integrating information and physical systems to connect humans, machines, products, and other production resources[9]. At present, DT has emerged as the primary digital solution for intelligent manufacturing and product life cycle management[10].
In the AEC industry, despite numerous studies exploring various aspects of DT, both stakeholder needs and strategies for its effective adoption have received insufficient attention[11]. This gap limits a comprehensive understanding of DT's full potential and hinders its transition from theory to practice. The practical applications of DT remain an area of ongoing exploration, with current research primarily focusing on challenges related to digital integration and data fusion[1]. For instance, Alizadehsalehi and Yitmen[12] developed an automated construction progress monitoring system, DRX, by integrating DT. This system captures accurate, real-time data to enhance transparency and facilitate intelligent building operations. Additionally, Kor et al.[13] proposed a framework that combines deep learning with DT to enhance real-time decision-making during the planning and construction phases. While some studies have employed systematic methods to analyze the status of DT applications, they remain limited. For example, Altan and Isık[14] used AHP-BOCR analysis to assess the barriers and drivers of DT adoption in lean construction. Moreover, Zhang et al.[4] examined technical strategies for DT implementation in the operation and maintenance phase, whereas Nabizadeh Rafsanjani et al.[1] investigated the key advantages and applications of Virtual Design and Construction (VDC) and DT[1]. However, these studies do not comprehensively address both internal and external factors influencing DT adoption. Furthermore, Jiang et al.[15] analyzed DT development directions using the Systematic Literature Review (SLR) method, but their approach lacked a quantitative assessment of the complex internal and external environments.
To bridge this research gap, this study offers a novel perspective by exploring DT's future development from the standpoint of stakeholder needs while incorporating a quantitative analysis of both internal and external factors. This approach enhances the understanding of DT's capabilities and adoption dynamics—areas that remain insufficiently explored in existing literature—thereby contributing to its more effective and widespread implementation in the AEC industry.
Accordingly, this study aims to investigate the application of DT in construction projects, conduct a comprehensive analysis of the SWOT associated with DT adoption, and propose a strategic development framework for its implementation within the AEC industry. First, an extensive literature review was conducted to identify the application of DT in the AEC industry and to analyze its corresponding strengths, weaknesses, opportunities, and threats. Second, a case study on DT adoption in the AEC industry was examined through participatory observations, interviews, and document analysis. Surveys targeting project participants were then conducted to assess the significance of the identified SWOT factors and their interrelationships. Third, the SWOT-AHP methodology was employed to perform a detailed analysis and evaluation of the impacts of these SWOT factors. Finally, the analysis indicates that the strategic quadrilateral exhibits the largest area in the fourth quadrant, with a value of 0.8554. The coordinates of the strategic center of gravity are (0.2321, -0.04135). These findings suggest that Strengths-Threats (ST) strategies are essential for the future development and adoption of DT, providing a foundation for tailored recommendations to enhance its effective development and implementation in the AEC industry.
2. Literature Review
2.1 The comparison between DT and BIM
There is a strong correlation between DT and BIM[11], yet it is crucial to distinguish differentiate between them[16]. BIM is a digital methodology based on 3D modeling that integrates visualization, simulation, and system integration capabilities. It is primarily used to facilitate collaboration and information sharing throughout the design and construction phases[17]. However, BIM models has limitations in processing dynamic, real-time data from building systems, which can result in inefficient decision-making and suboptimal operational performance, ultimately increasing costs[16]. In contrast, DT has a broader scope of data utilization[16] and is regarded as an advancement of BIM[11]. It is widely employed in advanced applications such as energy management, facility operations, structural health monitoring, and predictive maintenance, contributing to overall project management[18]. DT collects real-time data through sensors and other devices, enabling real-time monitoring. Additionally, it can optimize the structure and layout of civil engineering projects while predicting and assessing their behavior and performance under various conditions[17]. Moreover, BIM offers limited interactivity, restricting real-time user participation and primarily serving as a tool for designers and engineers during the design and construction phases[19]. In contrast, DT enhances interactivity and user engagement, making it accessible to a wider range of stakeholders[19]. By addressing BIM's limitations, DT presents an opportunity for more advanced and efficient building system management.
2.2 The basic mechanism of DT
DT enables virtual simulation, real-time monitoring, and intelligent decision-making[20]. Typically, the implementation of DT requires a data connection between a physical entity and its corresponding virtual model. Modeling techniques are used to generate a virtual model that reflects the parameters of the physical environment such as geometry, function, state, location, process and performance[21]. For existing buildings, geometric information for digital twins is obtained through 3D scanning, oblique photogrammetry, and LiDAR scanning, while real-time data collection is facilitated by integrating drone footage, high-definition cameras, sensors, and IoT technologies[4,22]. Consequently, the data and status of physical entities are continuously updated in real time, allowing simulation outcomes from virtual entities to be applied to their physical counterparts. This process enables virtual-reality integration and closed-loop control. Figure 1 illustrates the realization of DT.
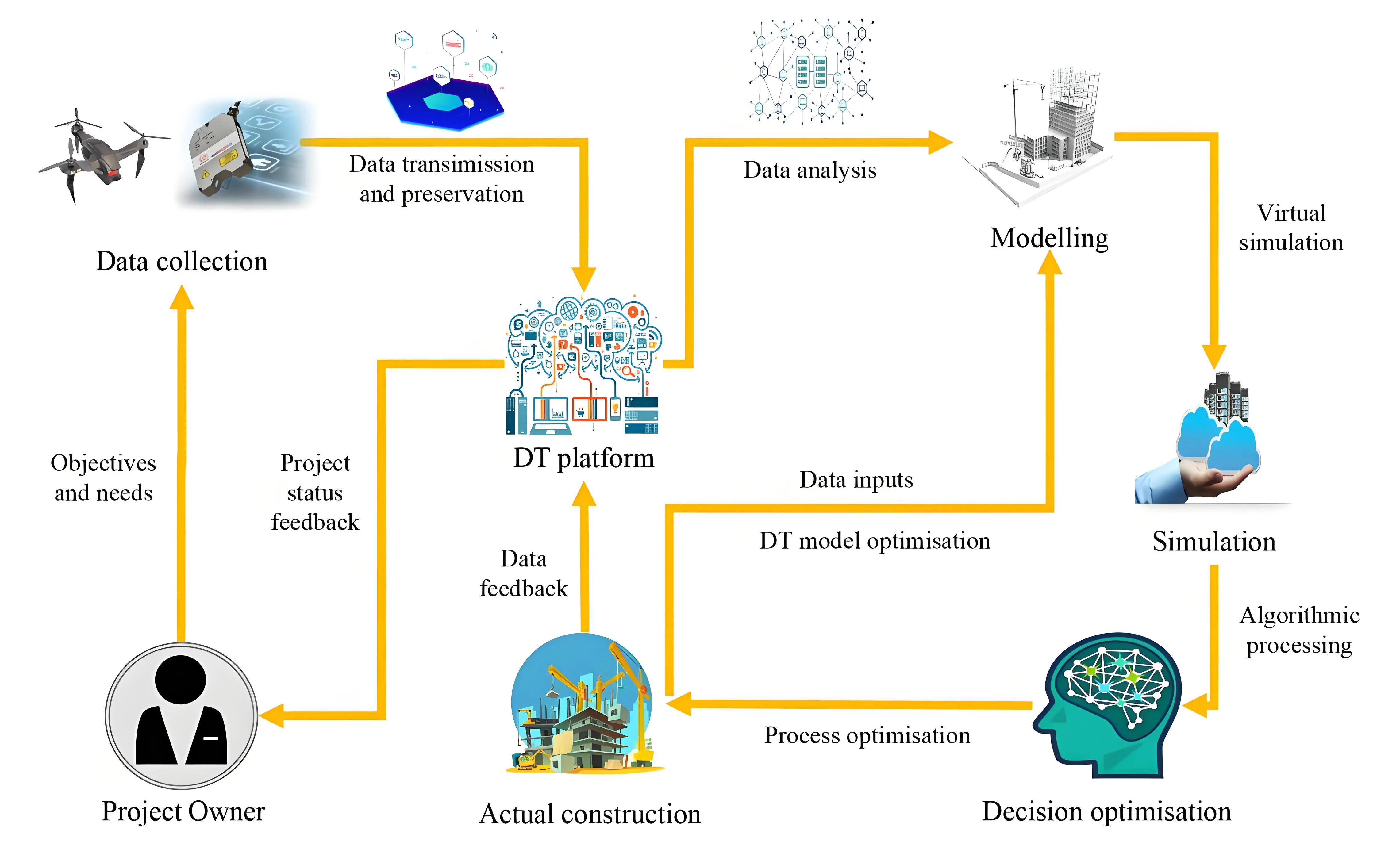
Figure 1. The basic mechanism of DT. The icons above were obtained from PNGKey . DT: digital twin.
2.3 The SWOT of the DT adoption in the AEC industry
2.3.1 Strength of the current state of DT use
The application of DT during the construction process can build a more efficient and scientific construction mode. For instance, Alsakka et al.[23] applied DT to production estimation, scheduling, and real-time monitoring in an off-site construction setting at a wall-framing workstation. Their study demonstrated that DT reduced deviations from the actual production schedule by 81% compared to the fixed-rate method commonly employed in traditional construction practice. In addition, Reuter et al.[24] developed an inspection planning method utilizing DT, which significantly minimizes manual inspection efforts for sill beam geometry measurements, reducing inspection time by up to 4.5 hours. The data-driven inspection approach enables precise evaluation of workpiece features, leading to a 75% reduction in overall inspection effort.
DT is also applicable in building structural health monitoring, enabling the detection and prevention of potential quality issues[25]. In Zheng et al.'s[26] research, five-dimensional DT models were developed to support various services, including quality monitoring and optimization, predictive maintenance, and the goal of zero defects. Additionally, a DT platform was developed for tunnel construction safety management, monitoring air quality, machine positioning, and worker comfort, with data displayed on a dashboard[27].
2.3.2 Weakness of the current state of DT use
Neither current hardware nor software can fully realize the vision of DT[28]. The implementation of DT depends on comprehensive physical data and the integration of various supporting technologies[29]. For instance, DT primarily incorporates advanced BIM-based technologies to optimize workflows, aiming for maximum efficiency in project designing and building projects[30]. In addition, DT requires engineers to possess both software development expertise and a deep understanding of the engineering disciplines being modeled[28]. However, the demand for such multidisciplinary talent remains a significant challenge.
Currently, DT standards are still in the development stage[31], and the data management capabilities of existing DT systems remain immature[32]. Additionally, the absence of a standardized data framework hinders data transmission and sharing due to data heterogeneity issues[33]. Data loss can also occur when storing information on cloud platforms, with losses of up to 2% when using the DT framework for data replication[34]. Moreover, the implementation of DT necessitates significant investment in IT infrastructure, such as setting up servers and communication links to enable two-way data flow between physical and virtual environments[18]. The associated costs are substantial, encompassing expenses for sensors, computing resources, metering equipment, and software licenses[35]. Implementation costs primarily consist of labor costs for installation, training costs, and encompassing operational expenses. Additionally, the cost of software licenses and future updates imposes a financial burden, potentially reducing the attractiveness of DT adoption[36]. Privacy concerns present another key challenge. Since DT involves the processing of physical objects and sensitive data, the high degree of interconnectivity between devices increases the risk of data breaches and information misuse[37]. Ensuring secure data handling and achieving regulatory compliance remains a significant hurdle[27]. Compliance, particularly regarding data protection and ownership, has emerged as a major barrier to DT implementation, as existing legal frameworks remain inadequate to address the complex data-related challenges within the DT context[38].
2.3.3 Opportunity of the current state of DT use
Governments in various countries are actively promoting the integration of information technologies within the AEC industry, implementing funding and research initiatives to enhance their nation's competitiveness in construction. In Spain, for instance, BIM adoption is accelerating and has even become mandatory for projects exceeding a certain scale. Further versions of BIM such as DT could also benefit from this favorable condition[27]. This offers both policy support and opportunities for the advancement of DT in the AEC industry. The transition to a more digitized development environment will also require the development of academia. Many colleges have made computer science programs a key part of their undergraduate engineering degrees[28].
A major challenge in DT implementation is the lack of real-time data transfer, which can be addressed by adopting appropriate communication protocols to facilitate seamless sensor-to-DT exchange[34]. Schroederet al.[30] proposed a model designed to enhance interoperability between various systems associated with DT, effectively managing and exchanging the complex information related to building components. Additionally, Ni et al.[39] incorporates the combination of IoT and AI into DT to develop digital models of historical buildings.
2.3.4 Threat of the current state of DT use
The AEC industry's understanding of DT remains superficial, with an unclear definition under Industry 4.0. This ambiguity is primarily driven by uncertainties surrounding emerging technologies and market demands, which impede the widespread adoption of DT[40]. Currently, the construction of sharing mechanisms and service systems related to DT is still incomplete, and there are still security risks and conflicts of interest in the sharing of data and models between different subjects, making it difficult to meet the requirements related to the development and sharing of data by DT[41].
DT is highly dependent on hardware systems, such as sensors. When integrated ultrasonic sensors exhibit high accuracy and precision, the monitoring capabilities of the DT system perform optimally[23]. However, using high-precision equipment on construction sites is challenging, as sensors and measurements are delicate and require careful handling[27]. Additionally, DT relies on the support of other technologies, such as VR and AR, which can provide various scenario prediction analyses for the projects[42]. In 2021, the global market for AR and VR technologies was valued at approximately $30 billion. With an average device cost of $40, the estimated global number of devices remains under 1 billion[1]. The number is insufficient for widespread DT applications. Table 1 presents a summary of the SWOT analysis for the use of DT derived from the literature.
Internal factors | Strengths | Weaknesses |
1. The capacity of analyzing large amounts of data. 2. The capacity of monitoring the status of a system in real time. 3. Accurate prediction and decision support. 4. Improve efficiency by optimizing resource allocation and processes. | 1. Complexity requirements and high costs for implementation. 2. High dependence on high quality and large scale data. 3. High demand for specialized knowledge and skills. 4. Lack of standardization and a risk that sensitive information may be compromised. | |
External factors | Opportunities | Threats |
1. Growing market demand for DT. 2. Support and promotion by government and industry policies. 3. Rapid technological advancement and its related fields. 4. Establishment of standards and regulations. | 1. Competitive technological pressures inside and outside the DT field. 2. Complex integration with other intelligent building management technologies. 3. Insufficient number of hardware devices for DT systems and potentially high maintenance costs. 4. Uncertainty in the use created by rapid changes in the market environment and customer needs. |
DT: digital twin; SWOT: strengths, weaknesses, opportunities, and threats.
3. Methods
A mixed-methods approach was employed in this study, combining both qualitative and quantitative methodologies. Qualitative methods were employed to identify the SWOT factors related to the application of DT in the AEC industry and to develop an understanding of how DT is implemented in real-world construction projects. To strengthen the validity of the findings, statistical analyses were conducted on the quantitative data to assess the significance of the identified SWOT factors. These insights subsequently informed the strategic direction for deployment of DT in the later stages of the research. Figure 2 presents the methodology flowchart utilized in this study.
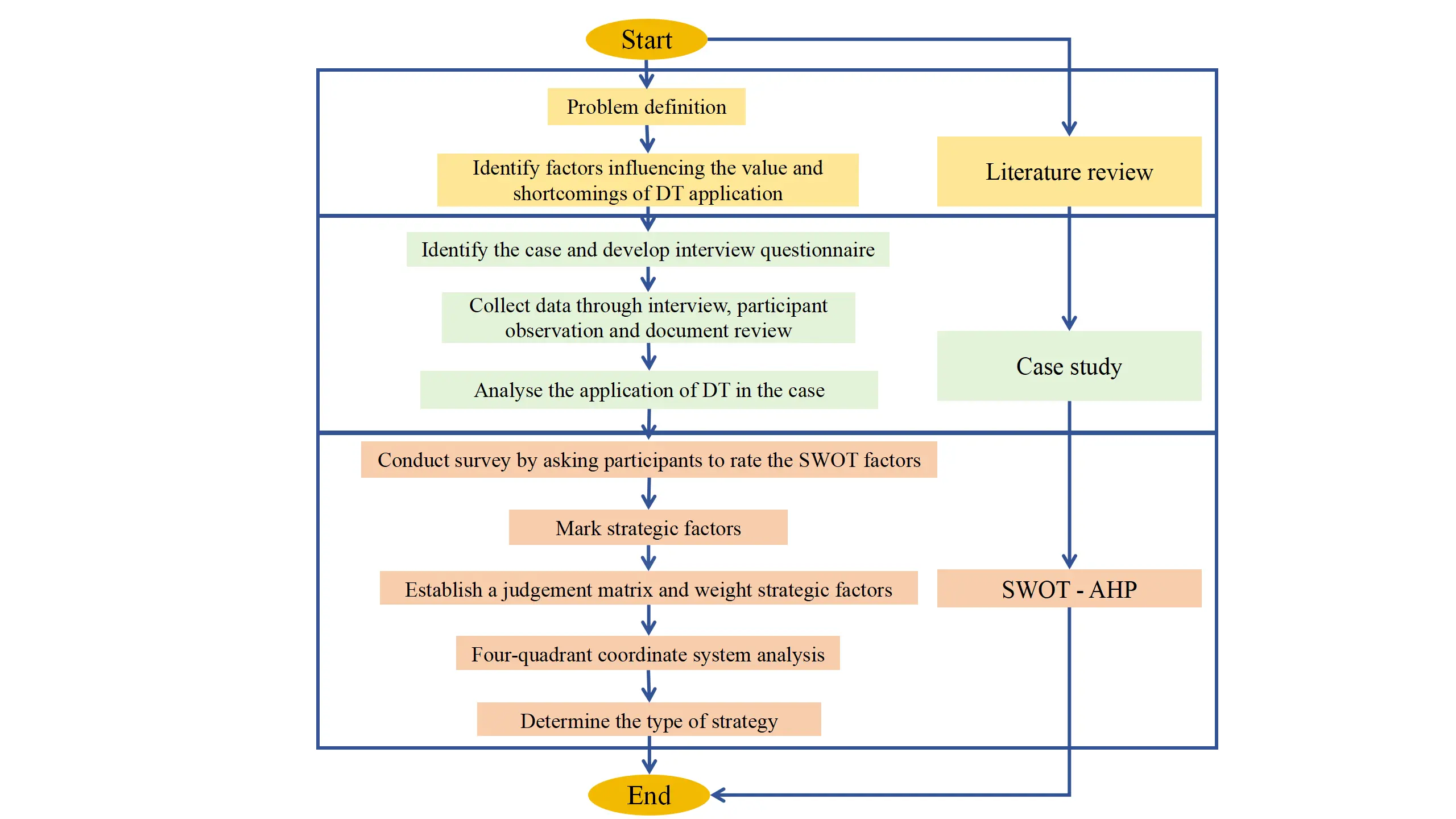
Figure 2. Research methodology flowchart. DT: digital twin; SWOT: strengths, weaknesses, opportunities, and threats; AHP: analytic hierarchy process.
3.1 Case study approach
The case study method employed not only to describe objective phenomena but also to explain, predict, and manage the development and transformation of these phenomena[43]. A construction project implementing DT was selected as case study for this research. The project's stakeholders include both private enterprises and government entities, providing a comprehensive perspective that captures the experiences and viewpoints of diverse stakeholders concerning regarding the application of DT.
The data collection tools used in the case study include participant observations, interviews, and document reviews. To gain a deeper understanding of the adoption of DT in the construction project, field visits were conducted, with the author spending two months working on the construction site. Semi-structured interviews were held with the on-site managerial staff, during which ten questions were posed to explore the use of DT in the project and to assess its strengths, weaknesses, opportunities, and threats. Additionally,, relevant documents were provided by the project staff as supplementary information.
The case was initially analyzed to investigate the implementation process of DT, and the results of the interviews were used to summarize the SWOT factors associated with DT adoption. Since the interview questions were consistent across participants, it was possible to compare and contrast the responses from different interviewees, thereby strengthening the conclusions.
3.2 SWOT-AHP approach
While SWOT analysis effectively identifies key factors in decision-making, it does not provide a quantitative measure of their relative importance, making it challenging to determine which factor has the greatest impact on a strategic decision. Empirically assessing causal relationships requires minimizing confounding factors that influence both causes and outcomes[44]. In the absence of such confounding influences, the impact of different factors can be analyzed quantitatively using the Analytic Hierarchy Process (AHP). The AHP is flexible and enables decision makers to assign relative importance to each factor through pairwise comparisons. When integrated with AHP, SWOT analysis provides a quantitative assessment of each factor's significance, enabling the estimation of their relative priorities within different categories. This combined approach is particularly useful for decision-making in contexts where influencing factors involve a degree of subjectivity[45], thereby enhancing the objectivity and comparability of SWOT analysis results.
Compared to other methods, such as Data Envelopment Analysis, which has lower discriminatory power[46], and qualitative analysis models, SWOT-AHP offers a more effective approach. Additionally, it is less complex and time-consuming than the ANP[47] and PROMETHEE[48] methods, making it a practical tool for decision-makers by improving data identification accuracy and strategy selection[49].
3.2.1 Data collection
A survey questionnaire was designed for project staff to evaluate the importance of the identified SWOT factors in DT adoption. A five-point rating scale was used, where 1 represents the lowest importance and 5 represents the highest. Additionally, respondents were asked to assess the relative importance of different factors based on the rating system outlined in Table 2. The questionnaires were distributed via the 'Questionnaire Star' application, and a total of twenty valid responses were collected for further analysis.
Score | Degree of importance |
1 | Equally important |
3 | Slightly important |
5 | Obviously important |
7 | Strongly important |
9 | The most important |
2, 4, 6, 8 | Middle of the road between the two above |
1/2.......1/9 | significance ratio |
3.2.2 Data analysis
A hierarchical analytical model was constructed for the identified SWOT factors of DT adoption. The hierarchical analysis steps are to decompose the complex multi-factor decision-making problem into a number of factors, classify and group these factors according to the attribute relationship, and transform them into a hierarchical structure[50]. In this model, strength and opportunity factors are assigned positive values, while weakness and threat factors are assigned negative values.
The scores provided by participants were averaged to construct a judgment matrix, from which the weights of each factor and the maximum eigenvalues of the four multi-order matrices were calculated. A judgment matrix quantifies the importance of factors through pairwise comparisons. The quantitative analysis involves a total of five matrices: A, S, W, O, and T. In this process, matrix A first performs pairwise comparisons of the four SWOT categories. Subsequently, matrices S, W, O, and T conduct pairwise comparisons of their respective impact indicators, ultimately leading to a comprehensive analysis.
Utilizing the hierarchical model, a judgment matrix was developed for sixteen internal and external environmental factors. The strategic strengths and weights of each factor were then calculated using Excel, while the maximum eigenvalue was determined using MATLAB.
The remaining calculation objectives and corresponding formulas are summarized in Table 3. For a 4 × 4 matrix, the Random Consistency Index (RI) is 0.90[51]. The Consistency Index (CI) and Consistency Ratio (CR) are then computed using formulas (3.1) and (3.2)[52]. A judgement matrix passes the consistency test if CR is less than 0.1; otherwise, if CR exceeds 0.1, the matrix fails the consistency test[52]. The strength and weight of strategic factors are determined using formula (3.3)[53], which then enables the calculation of the total strategic strength using formula (3.4). By applying SWOT-AHP analysis and determining SWOT coordinates through formula (3.5), (3.6) and (3.7)[53], the strategic types and directions for DT development can be determined.
Calculation objectives | Calculation formula | Formula number |
Consistency evaluation indicator | CI = (λmax - n)/(n - 1) | (3.1) |
Consistency ratio | CR = CI/RI | (3.2) |
Calculate strategic factor strength with strategic factor weights | Strategic factor strength= strategic factor score × strategic factor weights (Weights of groups × Factor weights) | (3.3) |
Total strategic strength | Total strategic strength= ∑ intensity of each strategic factor within the group | (3.4) |
Coordinates of the strategic centre of gravity | (3.5) | |
Coordinates of the strategic azimuthal angle | (3.6) | |
Strategic intensity factor P | (3.7) |
CI: consistency index; CR: consistency ratio; RI: random consistency index.
4. Case Study
4.1 Project description and main features
The case study focused on a newly developed residential project in eastern China, funded by government investment. The project's key stakeholders included the investor (government), the construction contractor, financial institutions, and material suppliers. This case study provided a specific scenario: the decoration phase of residential areas and specialized systems including municipal greening. The project involved the application of DT as a systematic quality construction of decorative facilities, which covered the whole process from the point of view of quality checking. The quality checks in this project encompassed various aspects, including the interior finishes of all residential units, external environments, and public infrastructure. The scope of inspection extended to substations, waste transfer stations, power systems, fire protection systems, fire monitoring rooms, and IT systems.
4.2 Application of DT in the project
The construction techniques used for the project's decorative finishes were diverse, with DT technology primarily employed for quality inspection, as illustrated in Figure 3. Outdoor data collection for residential facades and municipal landscapes primarily employed drone technology. Equipped with 3D laser scanning capabilities, the drones rapidly captured essential information, including spatial coordinates, reflectivity, and surface texture. The software enabled direct measurements of spatial dimensions, headroom, and flatness from the resulting point cloud model. Furthermore, the generated point cloud model was compared with the digital design model to detect geometric discrepancies. This process provided an accurate assessment of the overall quality of architectural decoration, facilitating enhanced quality control throughout the project.
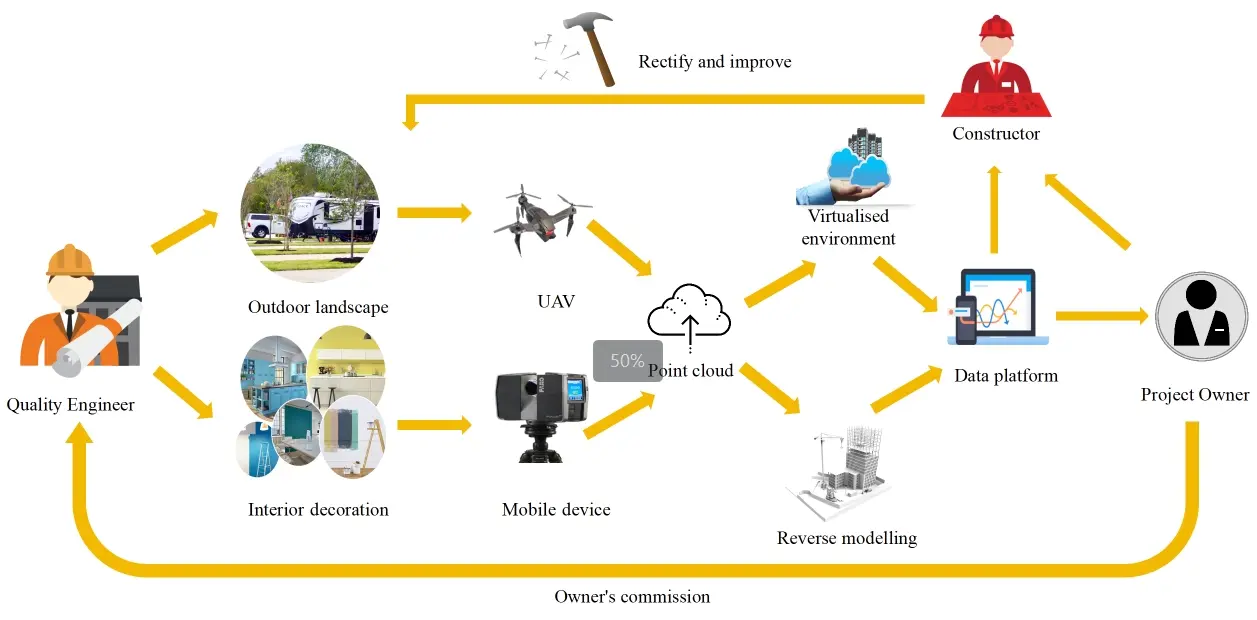
Figure 3. The DT implementation workflow. The icons above were obtained from PNGKey . DT: digital twin; UAV: unmanned aerial vehicle.
During the internal inspection, construction technicians monitored the installation status of each component using high-definition cameras. Upon completing each process, the on-site technician promptly recorded it in the real-time monitoring system. The high-resolution photos taken on-site were instantly transferred to the backend database. The inspection records included details such as the project undertaking unit, owner unit, transfer unit, and the specific inspection sub-items. Locations were documented with precision, down to the exact floor and corresponding house number. Inspection issues were categorized to facilitate organization, and to enhance accountability, each inspector was assigned a unique account number linked to their inspection records and upload times. Once problem images were uploaded to the database, builder could identify and locate defects through the photos, enabling timely rectification of decorative issues.
The inspection process encompassed approximately 1,000 households, covering a total construction area of around 100,000 square meters. As shown in Figure 4, the summarized information amounted to 9,900 entries. Issues identified in the decoration project were recorded in the project information platform, with accompanying photos providing visual details for better clarity. This made it easier for subcontractors to understand the situation. Compared to traditional quality management methods, this approach enabled real-time monitoring of the construction process, allowing for prompt feedback and corrective actions. Additionally, it efficiently managed large volumes of information, significantly improving overall work efficiency. However, due to the high volume of messages, the platform occasionally experiences crashes, which could hinder progress.
4.3 Challenges of using DT in the project
Data interaction and storage issues in this project hindered the effectiveness of DT, as real-time information exchange is crucial for its optimal performance. The data collected from sensors and video images in this project were independent, and their large quantities posed a challenge for the device to store and access the real-time data. The building site's environmental factors were complex, and despite the use of sensors to gather data, they did not provide an accurate picture of the spatial state of the building. This limitation required more efficient computer processing capabilities, which at that time could only be managed by human intervention. In addition, the current database storage capacity was severely limited, with the maximum number of files that could be exported at one time restricted to 200. This clearly could not meet the real need for nearly 10,000 pieces of information in this project.
5. Results & Discussion
5.1 Results of SWOT-AHP hierarchical analysis model
First, the AHP evaluation index was normalized by calculating the eigenvector λ. The maximum eigenvalue was then obtained using MATLAB, with the detailed calculation process provided in supplementary materials .
The maximum eigenvalues of the A, S, W, O, and T matrices are 4.1213, 4.2106, 4.1649, 4.0710, and 4.2353, respectively. Table 4 shows the consistency test results for each judgement matrix. As shown in the table, the CR values are below 0.1, indicating that there is good internal consistency among the influencing factors.
Judgement matrix | λmax | CI | RI | CR | Pass or fail the test |
A | 4.1213 | 0.0404 | 0.90 | 0.0449 | Pass |
S | 4.2106 | 0.0702 | 0.90 | 0.0780 | Pass |
W | 4.1649 | 0.0550 | 0.90 | 0.0611 | Pass |
O | 4.0710 | 0.0237 | 0.90 | 0.0263 | Pass |
T | 4.2353 | 0.0784 | 0.90 | 0.0871 | Pass |
CI: consistency index; CR: consistency ratio; RI: random consistency index. S: strengths; W: weaknesses; O: opportunities; T: threats; A: performs pairwise comparisons of the four SWOT categories.
Table 5 below presents the results of strategic factor weights and strategic strengths. Based on the hierarchical analysis weight calculation, the data shows that the strategic factor strength of "Strength" is 1.4907, which holds a clear advantage over the other strategic elements. In contrast, the strategic factor strength of "Weakness" is -0.5623, the lowest among the strategic factors. The results in Table 5 are further illustrated in a more intuitive manner through a bar graph, as shown in Figure 5.
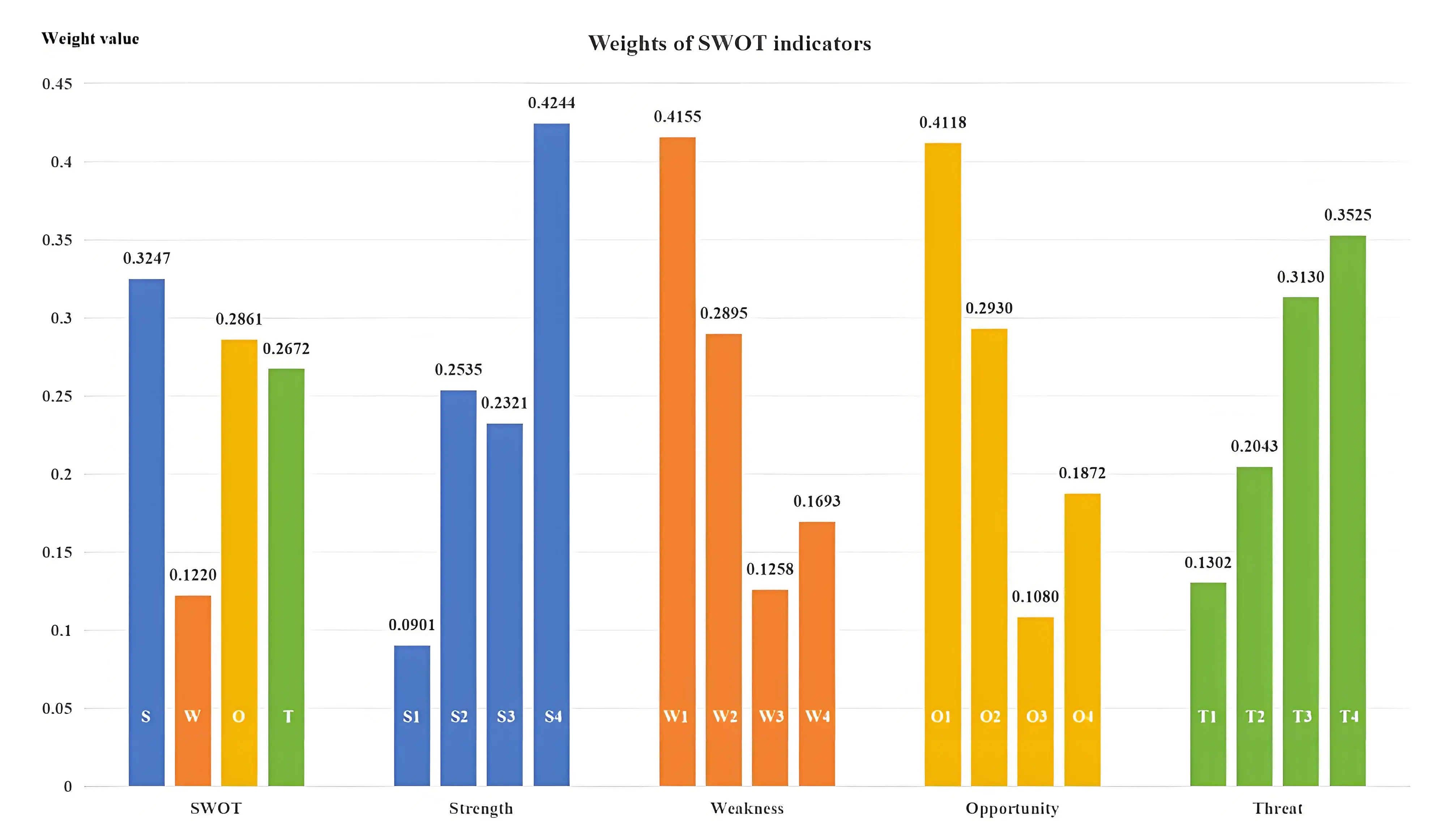
Figure 5. Weights of groups and SWOT indicators. SWOT: strengths, weaknesses, opportunities, and threats.
Dimension | Weights of groups | Elements of weights within groups | Factor weights | Strategic factor weights | Strategic factor score | Strategic factor strength | Total strategy strength |
S | 0.3247 | S1 | 0.0901 | 0.0292 | 4.35 | 0.1272 | 1.4907 |
S2 | 0.2535 | 0.0823 | 4.60 | 0.3787 | |||
S3 | 0.2321 | 0.0753 | 4.75 | 0.3579 | |||
S4 | 0.4244 | 0.1378 | 4.55 | 0.6269 | |||
W | 0.1220 | W1 | 0.4155 | 0.0507 | -4.80 | -0.2433 | -0.5623 |
W2 | 0.2895 | 0.0353 | -4.35 | -0.1536 | |||
W3 | 0.1258 | 0.0153 | -4.85 | -0.0744 | |||
W4 | 0.1693 | 0.0207 | -4.40 | -0.0909 | |||
O | 0.2861 | O1 | 0.4118 | 0.1178 | 3.20 | 0.3770 | 0.9823 |
O2 | 0.2930 | 0.0838 | 2.90 | 0.2431 | |||
O3 | 0.1080 | 0.0309 | 3.75 | 0.1159 | |||
O4 | 0.1872 | 0.0536 | 4.60 | 0.2464 | |||
T | 0.2672 | T1 | 0.1302 | 0.0348 | -4.90 | -0.1705 | -1.1477 |
T2 | 0.2043 | 0.0546 | -3.80 | -0.2074 | |||
T3 | 0.3130 | 0.0836 | -4.70 | -0.3931 | |||
T4 | 0.3525 | 0.0942 | -4.00 | -0.3767 |
S: strengths; W: weaknesses; O: opportunities; T: threats.
5.2 Discussion
As illustrated in Figure 5, strengths hold the highest weight, with a value of 0.3247. This indicates that the AEC industry places considerable emphasis on the strengths of DT, particularly its ability to optimize resource allocation and improve process efficiency. Interviews with project management staff revealed a consensus on the advantages of DT in improving construction efficiency, while they identified the complexity and extensive requirements for its implementation as significant weakness. For instance, project management personnel in the interview expressed concerns about the current immaturity of DT, noting that data modeling using 3D laser scanning needs further refinement to be effectively applied on-site. Additionally, they highlighted the challenges associated with data storage and the complexity of finding viable solutions.
In terms of opportunities, most participants believe that the growing market demand presents a favorable environment for the advancement of DT. Currently, BIM is the most widely adopted emerging technology in the industry. However, due to the vast amounts of data and information, BIM struggles to effectively integrate all collected parameter data. In contrast, DT offers several distinct advantages over BIM, including real-time capabilities and collaborative applications.
Regarding threats, the analysis shows that T4 has the highest weight, closely followed by T3. From the stakeholders' perspective, the needs of governmental units and private companies significantly influence the use of DT. For instance, the main reason for implementing DT in the case project was that the project owner, a governmental entity, favored the implementation of new technologies in construction. Without the requests and support from governmental entities, the project may have considered alternative technologies. Furthermore, the adoption of DT in the project placed substantial financial pressure on construction contractors, potentially reducing their motivation to adopt the technology.
Considering the synergy among factors, the weights of S4 and O1 are the largest, respectively. The advanced data analysis capabilities offered by DT are highly appealing to the construction industry, driving the development of standards and regulations governing their use, which in turn fosters their broader adoption across the sector.
Considering the conflicts among the factors, the weights of W1 and T4 represent the largest proportions. DT may hinder project realization due to its complexity and high cost. Additionally, the rapid evolution of the market environment and changing customer demands could create discrepancies between resource allocation, investments, and current requirements, further exacerbating uncertainty and potential risks. Therefore, DT should adopt more dynamic and flexible strategies to effectively respond to the changing external conditions.
5.2.1 Define DT development strategies
To better understand the influence of each SWOT indicator on the DT application process, a strategy quadrilateral has been established based on the weights of the SWOT indicators, as shown in Figure 6.
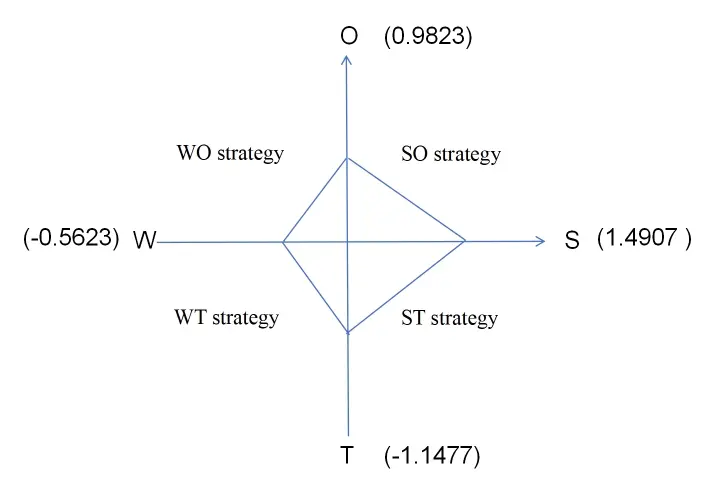
Figure 6. SWOT strategic quadrilateral. SWOT: strengths, weaknesses, opportunities, and threats.
The area of the four triangular regions formed by the strategic quadrilateral and the coordinate axes is calculated. S▲SO = 0.7322; S▲ST = 0.8554; S▲WO = 0.2762; S▲WT = 0.3227. The strategic quadrilateral occupies the largest area in the fourth quadrant. The strategy for the future development of DT should focus on leveraging its internal strengths while mitigating external threats.
To determine the specific strategy type, the centre of gravity coordinate of the strategy quadrilateral and the strategy azimuth are first calculated. The strategy type and orientation are then determined using the strategy type and strategy intensity spectrum in polar coordinates. According to formulas (3.5) and (3.6), the coordinate of the strategic centre of gravity is (0.2321, -0.04135) and the strategy azimuth Θ is -10.1°.
The strategic intensity factor (P) is calculated according to formula (3.7), resulting in a value of 0.6941. Since the strategic intensity factor P is greater than 0.5 and the centre of gravity coordinates line in the fourth quadrant, the ST strategy should be adopted for the future development of DT, as shown in Figure 6. Accordingly, the detailed strategies for different stakeholders are discussed below.
5.2.2 Specific measures of ST strategies
To promote the application of DT in the AEC industry, the following ST strategies are proposed based on the interview records and reflecting the perspectives of various stakeholders.
5.2.2.1 Create typical demonstration projects using DT (government and industry)
Currently, many professionals in the AEC industry lack sufficient knowledge about DT and its applications in their projects. Therefore, it is crucial to showcase successful case studies that demonstrate the effective use of DT in typical engineering projects. The implementation of DT demonstration projects can increase companies' familiarity with the technology, thereby enhancing their recognition of its value[11]. Once the benefits of DT are recognized and accepted within the industry, promoting its widespread adoption will become more feasible.
5.2.2.2 Establish application standards and specifications (government and industry)
With the continuous emergence of new technologies, materials, and processes, many traditional standards are inadequate for supporting the DT realization model. Standardization plays a key role in promoting the adoption of DT by ensuring system consistency and interoperability. It also enhances the construction industry's understanding of the technology's benefits, facilitating its application[54]. Additionally, standardization can also reduce implementation complexity and optimize cost-effectiveness[55].
5.2.2.3 Actively give policy and financial assistance (government and industry)
The AEC industry is currently undergoing a critical transition to the digital era. Immature or unclear policies can hinder the industry's overall progress. Therefore, it is essential for governments and policymakers to adopt a comprehensive approach and implement forward-thinking policies that foster the sector's development[56]. To promote the adoption of DT, the government could implement supportive policies, such as financial subsidies, to reduce implementation costs. Additionally, the government can also consider to give incentives that support vendors to further develop and improve the DT related systems. Research by Yang and Ng[11] highlights the effectiveness of government subsidies in promoting the adoption of DT, which can influence key stakeholders' decisions in construction projects.
5.2.2.4 Combine DT with other powerful information technology (technical service providers)
Technical service providers can greatly enhance their offerings by integrating DT with advanced information technologies such as artificial intelligence (AI), blockchain, and robotics technology. This integration improves data collection and analysis processes, enhancing data security through blockchain, which helps mitigate the risk of information leakage from databases[57]. DT can also adopt Privacy-Preserving Record Linkage in healthcare services, enabling data integration without compromising privacy, thus reducing the risk of privacy breaches[58]. AI can make a substantial impact by allowing engineers to input their ideas into DT models, optimizing strategies, and generating more effective solutions[1]. Furthermore, AI can update the DT by analyzing real-time data from sensors and other sources, thereby enhancing the decision-making capabilities of DT. Robotics also plays an increasingly important role in dynamically controlling DT construction systems[15]. Regarding data storage, the vast amounts of DT data can be stored in cloud systems, overcoming the limitations of traditional storage methods[59]. This integration will create a competitive advantage for the future application of DT.
5.2.2.5 Increase enterprise capital investment and talent training (construction contractors)
Contractors should adopt a proactive approach[56], increasing their financial investments in DT while simultaneously training their workforce to meet the growing demand for DT talent[55]. Additionally, contractors should continuously explore effective applications of DT, leveraging lessons learned to maximize the technology's potential and ensure its successful implementation.
5.3 Limitations and recommendations
Individuals who are heavily involved in the project and possess experience with DT are invited to participate in the study and share their insights based on their expertise. However, the sample size of participants for the interviews remains relatively limited, and the interviewees' views may be subjective and restricted in scope. Therefore, future studies could expand the sample size to gather a broader range of practitioners' perspectives on DT adoption.
Although the consistency check of the matrix shows that the CR value is less than 0.1, indicating that the matrix passed the consistency test, this does not completely eliminate the possibility of subjective biases in the participants' ratings, such as personal experience and preferences for digital technologies. Therefore, it is recommended to increase the number of participants in the survey and to conduct multiple rounds of feedback and discussion to further mitigate these biases, thereby enhancing the objectivity and consistency of the ratings.
In addition, the data is mainly collected from one construction project exploring the use of DT in China, so the results may need further validation in other contexts. Therefore, it is recommended that the use of DT in other countries can also be investigated to formulate customized strategies to better fit for the development and the implementation of DT in the local region.
From the perspective of DT application, the use of DT in this case study is limited to the decoration stage. In the future, DT can leverage advanced technologies such as AI and big data analytics to enable predictive maintenance and optimization of complex systems[60]. By extending across the entire project life cycle, DT can facilitate continuous improvement and optimization of engineering solutions[61].
6. Conclusions
The emergence of DT provides a new solution to address the existing problems in the AEC industry. This research, while reviewing the current use of DT in the sector, examined DT applications in a sample construction project through a combination of qualitative and quantitative methods. A case study approach was employed to identify both the advantages and challenges of implementing DT on-site. The degree of influence of each identified SWOT factor was further quantitatively analyzed using the AHP method. Finally, based on the strategic quadrangle, ST strategies were identified as the most suitable for future DT development, and specific strategies were proposed for different stakeholders to consider and implement.
The case study result provides practical insights into how DT can be implemented in construction projects, and the summarized DT implementation workflow can serve as guide for other practitioners to better utilize DT in their projects. The SWOT-AHP analysis reveals that the strengths of DT significantly favor its adoption. While DT offers a wealth of data that can enhance decision-making capabilities, its implementation requires substantial capital investment and specialized expertise. Moreover, the digitalization of the construction sector has not yet achieved full market maturity, suggesting that widespread adoption will take several years. ST strategies will be crucial in facilitating the future development of DT, and addressing current deficiencies will promote its broader adoption in the AEC industry.
Supplementary materials
The supplementary material for this article is available at: Supplementary materials .
Acknowledgment
The authors would like to sincerely convey our thanks for the support provided by the practitioners that participated in this project.
Authors contribution
Pan Z: Article conception and design, data analysis and interpretation.
Guo F: Article conception and design, substantive revision of the manuscript.
Conflicts of interest
Fangyu Guo received the financial support from Glodon company. Other authors have no conflicts of interest to declare.
Ethical approval
This research has obtained the approval from our university research ethics review panel with the document no. ER-LRR-0010000037820230915143020. Any opinions, findings, conclusions, or recommendations expressed in this paper are those of the authors and do not necessarily reflect the views of the various stakeholders.
Consent to participate
Obtained from all participants.
Consent for publication
Not applicable.
Availability of data and materials
Not applicable.
Funding
This work is supported by Glodon Company under the research project RDS10120220066.
Copyright
© The Author(s) 2025.
References
-
1. Rafsanjani HN, Nabizadeh AH. Towards digital architecture, engineering, and construction (AEC) industry through virtual design and construction (VDC) and digital twin. Energy Built Environ. 2023;4(2):169-178.
[DOI] -
2. Zeyu M, Gonzalez VA, Zou Y. Exploring a Digital Twin Framework for lean management of constraints in construction: a literature review. IOP Conf Ser: Earth Environ Sci. 2022;1101:082019.
[DOI] -
3. Christian F. Industry 4.0: The Digital German Ideology. tripleC. 2018;16(1):280-289.
[DOI] -
4. Zhang A, Yang J, Wang F. Application and enabling digital twin technologies in the operation and maintenance stage of the AEC industry: A literature review. J Build Eng. 2023;80:107859.
[DOI] -
5. Khudhair A, Li H, Ren G, Liu S. Towards Future BIM Technology Innovations: A Bibliometric Analysis of the Literature. Appl Sci. 2021;11(3):1232.
[DOI] -
6. Dihan MS, Akash AI, Tasneem Z, Das P, Das SK, Islam MR, et al. Digital twin: Data exploration, architecture, implementation and future. Heliyon. 2024;10(5):e26503.
[DOI] -
7. Durão LFCS, Zancul E, Schützer K. Digital Twin data architecture for Product-Service Systems. Procedia CIRP. 2024;121:79-84.
[DOI] -
8. Wehking S, Riedelsheimer T, Tanrikulu C, Lindow K. Developing Digital Twins for energy efficiency in the production phase of products. Procedia CIRP. 2024;122:933-938.
[DOI] -
9. Kampa A. Modeling and Simulation of a Digital Twin of a Production System for Industry 4.0 with Work-in-Process Synchronization. Appl Sci. 2023;13(22):12261.
[DOI] -
10. Zio E, Miqueles L. Digital twins in safety analysis, risk assessment and emergency management. Relia Eng Syst Saf. 2024;246:110040.
[DOI] -
11. Yang J, Ng ST. Prospects for digital twin technology in the building modular construction and operation phases: a game theory-based analysis. J Clean Prod. 2024;470(10):143344.
[DOI] -
12. Alizadehsalehi S, Yitmen I. Digital twin-based progress monitoring management model through reality capture to extended reality technologies (DRX). Smart Sustain Built Environ. 2023;12(1):200-236.
[DOI] -
13. Kor M, Yitmen I, Alizadehsalehi S. An investigation for integration of deep learning and digital twins towards Construction 4.0. Smart Sustain Built Environ. 2023;12(3):461-487.
[DOI] -
14. Altan E, Işık Z. Digital twins in lean construction: a neutrosophic AHP-BOCR analysis approach. Eng Constr Archit Manag. 2024;31(12):5029-5056.
[DOI] -
15. Jiang Y, Su S, Zhao S, Zhong RY, Qiu W, Skibniewski MJ, et al. Digital twin-enabled synchronized construction management: A roadmap from construction 4.0 towards future prospect. Dev Built Environ. 2024;19:100512.
[DOI] -
16. Tuhaise VV, Tah JHM, Abanda FH. Technologies for digital twin applications in construction. Autom Constr. 2023;152:104931.
[DOI] -
17. Huang J, Wu P, Li W, Zhang J, Xu Y. Exploring the Applications of Digital Twin Technology in Enhancing Sustainability in Civil Engineering: A Review. Struct Durab Health Monit. 2024;18(5):577-598.
[DOI] -
18. Hafeez MA, Procacci A, Coussement A, Parente A. Challenges and opportunities for the application of digital twins in hard-to-abate industries: a review. Resour Conserv Recycl. 2024;209:107796.
[DOI] -
19. Banfi F, Brumana R, Salvalai G, Previtali M. Digital Twin and Cloud BIM-XR Platform Development: From Scan-to-BIM-to-DT Process to a 4D Multi-User Live App to Improve Building Comfort, Efficiency and Costs. Energies. 2022;15(12):4497.
[DOI] -
20. Hoske MT. Digital twins advance digital transformation, control system integration. Control Engineering [Internet]. Cleveland (OH): WTWH Media LLC. Available from: https://www.controleng.com/digital-twins-advance-digital-transformation-control-system-integration/
-
21. Jones D, Snider C, Nassehi A, Yon J, Hicks B. Characterising the Digital Twin: A systematic literature review. CIRP J Manuf Sci Technol. 2020;29:36-52.
[DOI] -
22. Zhang J, Cheng JCP, Chen W, Chen K. Digital Twins for Construction Sites: Concepts, LoD Definition, and Applications. J Manag Eng. 2021;38(2):04021094.
[DOI] -
23. Alsakka F, Yu H, El-Chami I, Hamzeh F, Al-Hussein M. Digital twin for production estimation, scheduling and real-time monitoring in offsite construction. Comput Ind Eng. 2024;191:110173.
[DOI] -
24. Reuter L, Denkena B, Wichmann M. Adaptive inspection planning using a digital twin for quality assurance. Procedia CIRP. 2023;120:3-8.
[DOI] -
25. Bin H, Yu S, Yan W. Introduction to Special Issue Titled Digital Twin Driven Design and Manufacturing. J Comput Inf Sci Eng. 2021;21(3):030301.
[DOI] -
26. Zheng X, Psarommatis F, Petrali P, Turrin C, Lu J, Kiritsis D. A Quality-Oriented Digital Twin Modelling Method for Manufacturing Processes Based on A Multi-Agent Architecture. Procedia Manuf. 2020;51:309-315.
[DOI] -
27. Chacón R, Posada H, Ramonell C, Jungmann M, Hartmann T, Khan R, et al. Digital twinning of building construction processes. Case study: A reinforced concrete cast-in structure. J Manag Eng. 2024;84:108522.
[DOI] -
28. West TD, Blackburn M. Is Digital Thread/Digital Twin Affordable? A Systemic Assessment of the Cost of DoD's Latest Manhattan Project. Procedia Comput Sci. 2017;114:47-56.
[DOI] -
29. Sohyun P, Jisu H, Lim C, Chanwon J. Application of BIM Framework for Digital Twin Implementation. Soc Comput Des Eng. 2019;24(4):361-372.
[DOI] -
30. Schroeder GN, Steinmetz C, Pereira CE, Espindola DB. Digital Twin Data Modeling with AutomationML and a Communication Methodology for Data Exchange. IFAC-PapersOnLine. 2016;49(30):12-17.
[DOI] -
31. Angira S, Edward K, Jie Z, Alexandra B, Anisoara C. Digital Twins: State of the art theory and practice, challenges, and open research questions. J Ind Inf Integr. 2022;30:100383.
[DOI] -
32. Singh S, Shehab E, Higgins N, Fowler F, Reynolds D, Erkoyuncu JA, et al. Data management for developing digital twin ontology model. J Eng Manuf. 2021;235(14):2323-2337.
[DOI] -
33. El-Din MN, Pereira PF, Martins JP, Ramos NMM. Digital Twins for Construction Assets Using BIM Standard Specifications. Buildings. 2022;12(12):2155.
[DOI] -
34. Dellaly M, Skander-Mustapha S, Slama-Belkhodja I. A digital twin model-based approach to cost optimization of residential community microgrids. Global Energy Interconn. 2024;7(1):82-93.
[DOI] -
35. Mcclenaghan A, Gopsill J, Ballantyne R, Hicks B. Cost Benefit Analysis for Digital Twin Model Selection at the Time of Investment. Procedia CIRP. 2023;120:1197-1202.
[DOI] -
36. Oettl F, Eckart L, Schilp J. Cost estimation approach of a digital twin implementation in industry. Procedia CIRP. 2023;118:318-323.
[DOI] -
37. Kuštelega M, Mekovec R, Shareef A. Privacy and security challenges of the digital twin: systematic literature review. J Univ Comput Sci. 2024;30(13):1782-1806.
[DOI] -
38. Möhring M, Keller B, Radowski CF, Blessmann S, Breimhorst V, Müthing K. Empirical Insights into the Challenges of Implementing Digital Twins. In: Zimmermann A, Howlett RJ, Jain LC, editors. Human Centred Intelligent Systems. Singapore: Springer; 2022. p. 229-239.
[DOI] -
39. Ni Z, Eriksson P, Liu Y, Karlsson M, Gong S. Improving energy efficiency while preserving historic buildings with digital twins and artificial intelligence. IOP Conf Ser: Earth Environ Sci. 2021;863:012041.
[DOI] -
40. Pereira AC, Romero F. A review of the meanings and the implications of the Industry 4.0 concept. Procedia Manuf. 2017;13:1206-1214.
[DOI] -
41. Wang Y, Kang X, Chen Z. A survey of Digital Twin techniques in smart manufacturing and management of energy applications. Green Energy Intell Transp. 2022;1(2):100014.
[DOI] -
42. Aasish B, Harnish S, Kalyan P, Kapil CM. Application of augmented reality for remote collaborative work in architecture, engineering, and construction–a systematic review. Proc Hum Factors Ergon Soc Annu Meet. 2022;66(1):1829-1833.
[DOI] -
43. Wang Z, Wang H, Xu W, Zhu D, Xie WC. A new uncertainty analysis method based on geostatistical simulation and a case study. Case Stud Constr Mater. 2024;20:e02813.
[DOI] -
44. Yu D, Hwang C, Andersen OB, Chang ETY, Gaultier L. Gravity recovery from SWOT altimetry using geoid height and geoid gradient. Remote Sens Environ. 2011;265:112650.
[DOI] -
45. Etongo D, Bristol U, Epule TE, Bandara A, Sinon S. Expert elicitations of smallholder agroforestry practices in Seychelles: A SWOT-AHP analysis. Reg Sustain. 2023;4(3):282-295.
[DOI] -
46. Tavana M, Soltanifar M, Santos-Arteaga FJ, Sharafi H. Analytic hierarchy process and data envelopment analysis: A match made in heaven. Expert Syst Appl. 2023;223:119902.
[DOI] -
47. Sevkli M, Oztekin A, Uysal O, Torlak G, Turkyilmaz A, Delen D. Development of a fuzzy ANP based SWOT analysis for the airline industry in Turkey. Expert Syst Appl. 2012;39(1):14-24.
[DOI] -
48. Istiqomah DA, Windarni VA. Comparative analysis of the implementation of the AHP and AHP-PROMETHEE for the selection of training participants. In: 2019 4th International Conference on Information Technology, Information Systems and Electrical Engineering (ICITISEE); 2019 Nov 20-21; Yogyakarta, Indonesia. New York: IEEE; 2019. p. 67-72.
[DOI] -
49. Wu X, Tong Y, Zhou J. Strategy Selection of real estate by SWOT-AHP. J Phys: Conf Ser. 2019;1345:062006.
[DOI] -
50. Gu B, Zhai H, An Y, Khanh NQ, Ding Z. Low-carbon transition of Southeast Asian power systems—A SWOT analysis. Sustainable Energy Technol Assess. 2023;58:103361.
[DOI] -
51. Saaty TL. A scaling method for priorities in hierarchical structures. J Math Psychol. 1977;15(3):234-281.
[DOI] -
52. Vaidya OS, Kumar S. Analytic hierarchy process: An overview of applications. Eur J Oper Res. 2006;169(1):1-29.
[DOI] -
53. Kurttila M, Pesonen M, Kangas J, Kajanus M. Utilizing the analytic hierarchy process (AHP) in SWOT analysis—a hybrid method and its application to a forest-certification case. For Policy Econ. 2000;1(1):41-52.
[DOI] -
54. Saif W, Razavialavi S, Kassem M. Construction digital twin: a taxonomy and analysis of the application-technology-data triad. Autom Constr. 2024;167:105715.
[DOI] -
55. Weil C, Bibri SE, Longchamp R, Golay F, Alahi A. Urban Digital Twin Challenges: A Systematic Review and Perspectives for Sustainable Smart Cities. Sustain Cities Soc. 2023;99:104862.
[DOI] -
56. Su S, Zhong RY, Jiang Y, Song J, Fu Y, Cao H. Digital twin and its potential applications in construction industry: State-of-art review and a conceptual framework. Adv Eng Informatics. 2023;57:102030.
[DOI] -
57. Malaibari M, Siddiqui M, Xie S, Bahramimianrood B, Abdoli S, Djukic L. Digital Twin as A New Approach to Data Management: A Review. Procedia CIRP. 2024;128:375-380.
[DOI] -
58. Brown AP, Ferrante AM, Randall SM, Boyd JH, Semmens JB. Ensuring privacy when integrating patient-based datasets: New methods and developments in record linkage. Front Public Health. 2017;5:34.
[DOI] -
59. Jain U, Kumar S, Dubey S, Sharma O, Kumar Jain V. Virtualization-A New Dimension of Big Data. In: 2018 8th International Conference on Cloud Computing, Data Science & Engineering (Confluence); 2018 Jan 11-12; Noida, India. New York: IEEE; 2018. p. 803-808.
[DOI] -
60. Marino A, Pariso P, Picariello M. Digital Twin in SMEs: Implementing Advanced Digital Technologies for Engineering Advancements. Macromol Symp. 2024;413(3):2300176.
[DOI] -
61. Liu W, Tao F, Cheng J, Zhang L, Yi W. Digital twin satellite: concept, key technologies and applications. Comput Integr Manuf Syst. 2020;26(3):565-588.
[DOI]
Copyright
© The Author(s) 2025. This is an Open Access article licensed under a Creative Commons Attribution 4.0 International License (https://creativecommons.org/licenses/by/4.0/), which permits unrestricted use, sharing, adaptation, distribution and reproduction in any medium or format, for any purpose, even commercially, as long as you give appropriate credit to the original author(s) and the source, provide a link to the Creative Commons license, and indicate if changes were made.
Publisher's Note
Share And Cite