Abstract
Aims: This study aims to enhance the design of augmented reality (AR) technologies for remote collaboration by examining the complex relationships among individual factors (user characteristics), psychological and physiological states during AR-mediated remote collaboration, and outcomes within an Input-Mediator-Output (IMO) model. The goal is to evaluate how individual characteristics influence psychological and physiological experiences, as well as task performance, in AR-mediated collaboration.
Methods: We hypothesize and evaluate an IMO model and use correlation analyses to examine the relationships among person-related input variables (e.g., predispositions, traits, attitudes, states, and contextual factors), psychological and physiological emergent states, and performance-related output variables.
Results: Our results demonstrate that individual characteristics significantly influence subjective experiences, physiological responses, and task performance, emphasizing the critical role of individual differences, alongside task- and technology-related factors, in shaping collaboration experiences and performance. These findings highlight the importance of considering individual characteristics in the design of AR tools to optimize user well-being and performance outcomes.
Conclusion: Our study provides a foundational framework for understanding the interplay between individuals, tasks, and technology, underscoring the need for AR tools that align with user characteristics. It also lays the groundwork for future IMO research in AR-mediated remote collaboration, contributing to the development of more effective and health-promoting AR technologies.
Keywords
1. Introduction
Augmented reality (AR) is gaining increasing interest as a tool for supporting remote collaboration in both academia[1,2] and industry, with companies like Airbus and Henkel using AR for expert guidance in product assembly and line management[3-5]. While prior studies have extensively examined how technological and task-related characteristics shape user experience and performance, the role of user-related factors remains underexplored. However, individual differences—such as prior experience[6], cognitive predispositions[7], and physiological responses[8]—can significantly influence users' perceptions and performance. To develop AR solutions that are not only functional but also user-centric, it is crucial to systematically account for these factors.
This shift in focus is essential for advancing AR research beyond its current emphasis on technological feasibility and early-stage implementation. Marques et al.[2] position research on AR-mediated remote collaboration within the replication and empiricism phases of the BRETAM model[9], which describes the evolution of new information technologies through six phases: breakthrough, replication, empiricism, theory, automation, and maturity. This classification suggests that AR research has largely focused on demonstrating feasibility and refining implementation but has yet to transition toward theoretical development. Building on this perspective, we argue that expanding the research focus to incorporate individual differences as a core factor in AR effectiveness and user well-being represents a necessary step in this evolution.
Thus far, studies on perception and cognition in the field of AR-mediated remote collaboration have primarily considered person-related variables, such as mental load or presence, as outcome variables directly influenced by technological design features, including display configuration, communication channels, and media richness[10]. Moreover, due to the developmental nascent stage of AR-mediated remote collaboration, most studies regard performance outcomes as a product of technology and task, often neglecting person-related variables that might influence performance independently of these elements[11].
We argue that, in addition to task- and technology-based factors, emergent states (e.g., mental load, presence) and performance are also shaped by individual baseline predispositions. For instance, a person's susceptibility to mental load may vary based on age[12] and experience[6], just as individual differences are known to impact (job) performance[13]. By considering these person-related variables, AR systems can be designed not only to optimize task performance but also to support the well-being and engagement of all users, regardless of their individual characteristics.
Consequently, we propose that human-centered design should prioritize user requirements, by integrating person-related factors as independent variables that influence psychological well-being, physiological well-being, and performance in AR-mediated remote collaboration. While previous research has highlighted the role of individual characteristics in digital interactions[14-16], these studies have predominantly focused on conventional screen-based environments, overlooking the unique demands of AR-mediated collaboration. Addressing these factors is essential for developing AR solutions that promote user well-being, ensuring that user needs and predispositions serve as the foundation for design principles and development guidelines.
Moreover, implementing technology in organizations is challenging, as one-size-fits-all approaches often fail to align with individual needs, preferences, and demands[17]. Consequently, understanding the influence of individual characteristics that can be encouraged, promoted, or trained through human resource interventions or offerings is essential for enhancing psychological and physiological well-being, as well as technology-related performance. These person-related variables likely interact with both the task and technology. These linkages may manifest through interactions, such as mediation or moderation effects, leading to path analyses or structural equation models that elucidate Input-Mediator-Output (IMO) chains in AR-mediated remote collaboration.
This study contributes to understanding the complex interplay between person-related variables (as both inputs and mediators) and the outcomes of AR-mediated remote collaboration. To achieve this, we propose an IMO model grounded in current literature and test it using data from N = 220 participants in an exploratory manner. The data is derived from an empirical evaluation study of the Ambient Awareness Tool—an AR-based tool for supporting temporal coordination in remote collaboration teams[18].
Our objective is to illustrate the relationships between person-related variables and collaboration outcomes within the IMO framework. By examining these relationships, we offer valuable insights that can inform the design and implementation of AR technologies tailored to individual needs and preferences. Furthermore, our findings contribute to identifying strategies for implementing health-promoting AR systems for remote collaboration.
The following sections explore the key variables involved by describing their complex interplay and nuanced relationships shaping users' interactions with AR technologies and outcomes in the context of an IMO model.
2. Placing the Human in the Center: An IMO Model for AR-Mediated Remote Collaboration
IMO-models are recognized theoretical frameworks that help map, explore, and explain team- and task-work related outcomes, such as team effectiveness[19] or team adaptation[20]. Thereby, inputs (e.g., individual- and team-related variables) are mediated by emergent states (e.g., cohesion) and processes (e.g., coordination), leading to outputs (e.g., performance)[21]. IMO-models were initially developed for studying face-to-face teams[22]. With the rise of spatially dispersed teams in the organizational contexts, IMO-models have been widely applied to investigate virtual teams[23-25]. However, to our knowledge, AR-mediated remote collaboration teams have not yet been considered in the context of IMO research.
To establish a foundation for future IMO research in AR-mediated remote collaboration, this paper focuses on defining relationships between inputs, mediators, and outputs at the individual level. We consider individual predispositions (e.g., age), traits and attitudes (e.g., retentivity), states (e.g., self-efficacy), and contextual factors (team partner familiarity) as inputs; task- and technology-mediated emergent states (e.g., spatial presence) as mediators; and performance outcomes as outputs. We assume that these emergent states can be differentiated into psychological and physiological states, with physiological states influenced by both individual-related inputs and psychological emergent states. Figure 1 shows the hypothesized IMO-model for AR-mediated remote collaboration.
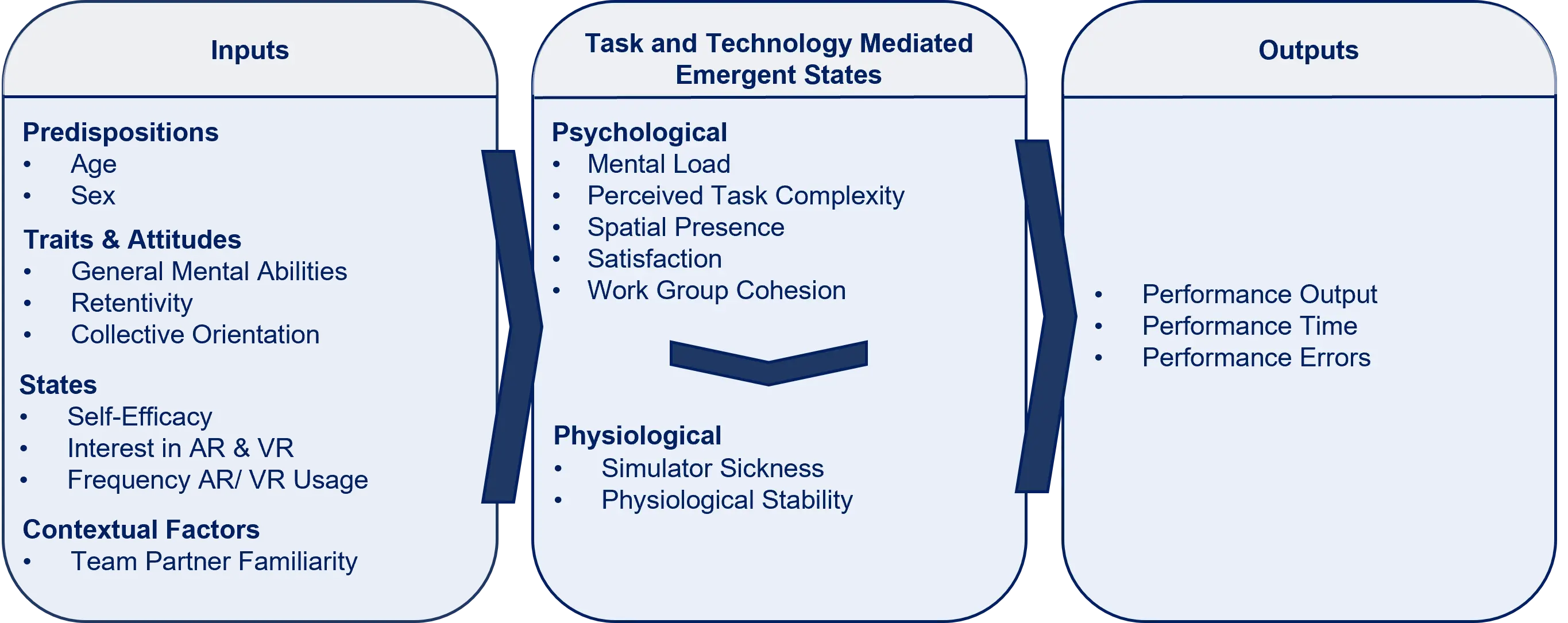
Figure 1. Hypothesized Input-Mediator-Output model for AR-mediated remote collaboration. AR: augmented reality; VR: virtual reality.
Since teams are complex systems, fundamental research should begin with simplified models to discern basic relationships and establish a foundational understanding before addressing more intricate scenarios. This study adopts a simplified approach, concentrating solely on the direct effects between inputs, emergent states, and outputs, omitting considerations of mediations. By narrowing the scope, we aim to lay the groundwork for understanding the mechanisms underlying AR-mediated remote collaboration.
The inputs, emergent states and outcomes, and their hypothesized relationships in the IMO-model are explained below.
2.1 Inputs
Inputs, defined as "antecedent factors"[19], are assumed to influence individual and team processes as well as emergent states. This paper focuses on the individual level, including individual predispositions, traits and attitudes, states, and contextual factors that are assumed to impact AR-mediated collaboration. Traits are stable individual characteristics[26] that are difficult to change, while states are "variable or fluctuating"[26] and more easily changed or influenced.
2.1.1 Predispositions
Age is a key predisposition in technology-mediated collaboration as it can significantly impact the perception of technology-mediated emergent psychological states, e.g. mental load or perceived task complexity[12], as well as physiological states, such as simulator sickness[27,28]. Furthermore, factors such as digital nativity and technological literacy in younger cohorts may affect their propensity for technology adoption and adaptation to AR systems. Additionally, age-related sensory system degeneration and cognitive decline[12] can influence physiological and psychological emergent states. For instance, impaired vision might lead to a decrease in perceived spatial presence, while diminished cognitive abilities might lead to higher mental load and perceived task complexity.
Sex can also significantly impact technology-mediated psychological and physiological emergent states. While research on AR-related sickness remains limited, findings from the field of virtual reality (VR) and motion sickness indicate, that women are more susceptible to VR- and motion-induced sickness[28,29], presumably due to physiological disparities. Sexual dimorphism, such as variations in pupillary distance, stature, and musculature can affect ergonomic dynamics and comfort levels associated with VR head-mounted displays[30]. Given the similarities in immersive technology experiences, these findings may offer insights into potential sex-related effects in AR use. Additionally, sex differences in cognitive abilities, like visuo-spatial abilities[31], may influence perceptions of AR tool supportiveness, thereby impacting psychological and physiological emergent states.
2.1.2 Traits and attitudes
General mental abilities are crucial for task and overall performance, especially in the workplace[32-35]. Similarly, good retentivity enhances performance, particularly in complex tasks, by facilitating performance time, error rates, and thus overall performance output[36]. Research on how these factors influence task- and technology-mediated emergent states, such as spatial presence or mental load is limited. However, it is reasonable to assume that general mental abilities and retentivity affect mental load and perceived task complexity due to their link to cognitive resources. Moreover, spatial presence, being a subjective psychological phenomenon, is likely influenced by or dependent on individual characteristics[7], with research suggesting general mental abilities and retentivity play pivotal roles in this context[7].
Collective orientation defined "as the propensity to work in a collective manner in team settings"[37], significantly influences team performance[38,39]. It is also linked to psychological emergent states like cohesion[40]. Collective orientation can be divided into two factors: dominance and affiliation[38]. Accordingly, collective orientation is strongly expressed when individuals exhibit a high need for belonging and a low tendency to dominate simultaneously[37].
2.1.3 States
Self-efficacy reflects an individual's confidence in achieving desired outcomes[41] and is closely tied to performance as well as physiological and psychological emergent states[42-44]. Higher self-efficacy in mastering AR-mediated team task may mitigate physiological discomfort, such as simulator sickness[45].
Moreover, prior training, experience, and domain-specific interest influence psychological emergent states such as spatial presence[7,46] and mental load in AR use[6]. While empirical research on adaptation effects in AR is still developing, findings from VR studies suggest that repeated exposure over hours or days may reduce simulator sickness[47]. Given the potential parallels in user adaptation across immersive technologies, similar effects might also be relevant for AR environments. Thus, interest in AR and VR, as well as frequency of use , may positively influence physiological and psychological emergent states. These variables were included to capture individual differences in general immersive technology experience, which could provide important insights into how prior exposure to immersive environments influences emergent states in AR specifically.
2.1.4 Contextual factors
Team partner familiarity is often considered in studies on AR-mediated remote collaboration[2], but it is typically used to describe the sample rather than to investigate its effects on psychological or physiological emergent states or performance. However, research in the field of virtual teams emphasizes that trust, facilitated by team partner familiarity, is one "key variable […] crucial for all aspects of collaboration"[48] and particularly linked to team performance[49]. Studies on video games also demonstrated that team partner familiarity affects factors like spatial presence[50], underscoring its importance as input in the proposed IMO-model for AR-mediated remote collaboration.
2.2 Task- and technology-mediated emergent states
Task- and technology-mediated emergent states encompass both psychological states (e.g., mental load) and physiological states (e.g., simulator sickness) that are influenced not only by the task and the technology but also by person-related inputs (e.g., gender or age). This study exclusively focuses on the influence of person-related variables on these states, while acknowledging the impact of task and technology design without examining it scientifically.
Psychological and physiological emergent states are experiences and perceptions whose relationship to other variables usually exhibit reciprocal causation, as they are both influenced by and influence other variables. For instance, job performance is significantly influenced by experience, and experience in turn is significantly affected by job performance[51].
2.2.1 Psychological emergent states
Mental load refers to the cognitive resources engaged by the task- and teamwork, with higher mental load linked to increased error rates and decreased performance[52], and negative effects on physiological and psychological well-being[53].
Perceived task complexity, subjectively gauging task difficulty, influences task performance[54], experience and perception (e.g., stress response[55] or mental load[56]), as well as physiological well-being (e.g., as a consequence of task complexity-induced stress).
Presence describes the "sense of being there", mirrored by interacting and responding accordingly in and with a virtual environment[57] or a simulation environment[58]. In simulation contexts, presence can be distinct in spatial presence and satisfaction. Spatial presence expresses how much the users feel as if being "there" within the simulated environment, whereas satisfaction indicates the degree of contentment working in a simulation. Results in relation to the effects of presence on performance are mixed and debated[59,60], yet several empirical studies showed a positive influence of perceived presence on performance[61]. Moreover, research indicates, that presence is related to physiological and psychological well-being[62,63].
Work group cohesion refers to the individual's belief "that the members of his or her work group are attracted to each other, willing to work together, and committed to the completion of the tasks and goals of the work group"[64]. It's a key determinant of team effectiveness, as evidenced by the well-documented link between work group cohesiveness and various performance outcomes[65].
2.2.2 Physiological emergent states
A major challenge in Virtual and Augmented Reality applications is technology-induced sickness. Factors influencing simulator sickness in VR include technology-related issues (e.g., latency, tracking accuracy, flicker[66,67]), experience and perception-related factors (e.g., perception of presence[68]), and person-related factors (e.g., sex[69], age[70]).
While VR content is more likely to cause motion-related sickness than AR, there is limited and mixed research on AR-related sickness[71]. Some studies reported negligible symptoms[11,72], while others found significant AR-induced sickness[73,74].
Simulator research suggests a strong link between sickness and performance[8]. To explore this relationship in AR-mediated remote collaboration, we included simulator sickness and perceived physiological stability after AR use in our hypothesized IMO-model.
2.3 Outputs
Since most AR-based remote collaboration applications are aiming at enhancing spatially dispersed task- and teamwork, task performance is one key performance indicator measured in the field of research[10]. Most studies focus on how technology-related design and implementation impact performance, including overall outcomes, accuracy, or task execution times[10]. Although many studies have considered the effects of psychological states such as mental load or presence on performance, there is still no comprehensive understanding of how physiological and psychological emergent states influence performance in AR-mediated remote collaboration.
To investigate potential relationships, we included performance output, performance time, and performance errors (as an indicator for performance accuracy) as output variables in our proposed IMO-model. The variables are detailed in Table S1.
3. Materials and Methods
3.1 Sample description
The data for this paper originate from an empirical study involving N = 264 participants, evaluating an AR-based interface for temporal coordination support in remote teams (Ambient Awareness Tool[18]). The study included n = 110 remote working dyadic teams and n = 22 side-by-side working dyadic teams (serving as a control group). Since this paper examines relationships between inputs, emergent states, and outputs relevant to AR-mediated remote collaboration, data from the side-by-side teams were excluded from the analyses. Consequently, the analyses presented below are based on a sample size of N = 220 participants. The study was conducted between October 2020 and January 2022 in the work unit's lab facilities. Of the participants, 96.36% were students from a German university. 68.18% identified as female (2 participants identified as non-binary). The average age was 23.98 years (SD = 3.71, range: 19;44), and 29.09% knew their team partner beforehand. While 46.82% had used a VR HMD before, only 6.82% had prior AR HMD experience. The study was approved by the local ethics committee (no 440) and adhered to the 'Deutsche Forschungsgemeinschaft' guidelines[75]. All participants provided written informed consent and met prerequisites, including being of legal age, having normal or corrected-to-normal vision, and no prior experience with the experimental use case (see below). Participants were compensated with either €40 or course credit ("Versuchspersonen-Stunden") for their participation.
3.2 Use case and study design
For this study, we employed WaTrSim (wastewater treatment plant simulation), a process control simulation of a wastewater treatment facility[76-80]. WaTrSim models a wastewater treatment plant that pursues the goal of treating wastewater produced in industrial cleaning processes. In the process, mixtures of approximately 60% water and 40% solvent are separated. To initiate this process, participants followed a Standard Operating Procedure (SOP) comprising 13 sequential steps in an act-wait-act format to optimize purified water output. These steps include adjusting valves, heater, and tank settings according to predefined process constraints and could be carried out digitally via tablet.
To examine remote collaboration, participants assigned to separate rooms, each equipped with two on-wall projected WaTrSim task displays: one for the individual task and one for the team task (Figure 2). As participants could only view one screen at a time, they had to physically turn and move their gaze to the opposite wall to check the status of the technical process. Consequently, participants could not directly perceive task state information from both screens at the same time, requiring ambient awareness of the screen that could not be monitored directly.
3.2.1 Task execution and monitoring
Participants in dyadic teams had to start-up WaTrSim on their own (individual task) and simultaneously in collaboration with their team partner (team task). While the individual task required the execution of all 13 steps of the SOP individually, in the team task, each member had to perform a predetermined subset of the 13 steps in alternating turns. Further, participants had to press a "monitoring" button, every 50 seconds (in both individual and team task) as a secondary task.
3.2.2 The ambient awareness tool
To support remote collaboration, we implemented an AR-based Ambient Awareness Tool for Microsoft HoloLens 1[81,82]. The tool displayed process state information from the unobserved task, allowing participants to anticipate upcoming SOP steps.
The interface presented three AR-based symbols, each representing the next required SOP step (Figure 3). The topmost symbol indicated the next immediate task and flashed when actionable. Once a team member completed a task, the other team member was notified via the flashing top symbol, signaling the transition of responsibility. The tool was positioned at the left periphery of the WaTrSim simulation surface to ensure minimal distraction while maintaining continuous access to critical process information.
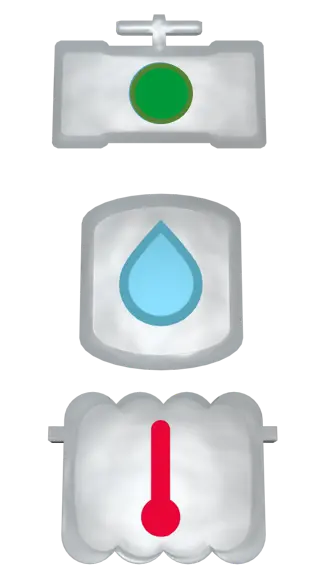
Figure 3. Ambient Awareness Tool depicting the next three steps of the task the user is currently not working on. From top to button: valve, tank, heater.
The design of the Ambient Awareness Tool was iteratively refined and validated through a usability study[82] to ensure intuitive interpretation and effective remote collaboration support.
3.2.3 Experimental procedure
Each experimental session (including questionnaires, training, and main experiment, cf, Figure 4) lasted approximately four hours, with participants completing six operational trials in WaTrSim. Each trial had a maximum duration of 240 seconds. The first two trials served as training and were excluded from the analysis.
3.3 Measurement of inputs, psychological and physiological emergent states, and outputs
Table S1 describes the measurement of the variables based on their time of assessment (1 to 4, cf. Figure 2). Questionnaire data were collected online via Qualtrics. As the study was conducted at a German university, all questionnaires were administered in German. Example items have been translated into English.
Performance data were retrieved from log files produced by the WaTrSim application. These log files captured key user interactions, including timestamps of actions, task execution times, and errors, providing an objective measure of task performance, capturing adherence to the SOP and efficiency.
Each team completed six trials, with the first two considered training trials and excluded from the analysis. In each trial, participants performed both an individual task (starting up the simulation independently) and a team task (starting up the simulation collaboratively), resulting in three log files per trial: one for each participant and one for the team. To analyze the data, a mean value was calculated for each participant's individual task performance based on their performance across the four trials. A separate mean value for the team task was calculated, applicable to both team members. An overall mean value for each participant was then determined by averaging the individual task mean with the shared team task mean. This approach enabled the combination of both individual and collaborative performance in the analysis.
Performance output (measured in liters of purified water) was derived from the log files, which recorded each user action along with the corresponding production output at that specific timepoint. For analysis, the final production output was extracted at the time of the last recorded action within the simulation. On average, participants produced M = 328.40 liters (SD = 100.87, range = 1.92; 557.03), with higher values indicating better performance.
Performance time was determined based on recorded simulation steps in the log files, with each simulation step corresponding to one second. The total number of simulation steps at the time of the last recorded action was used to calculate task completion time. On average, participants required M = 155.58 seconds to start up WaTrSim (SD = 24.79, range = 103.88; 229.25), with lower values indicating faster task execution.
Performance errors were identified based on the actions recorded in the log files. Errors were defined as adjustment errors (incorrect value settings) or procedure errors (adjustments made to the wrong plant part). An Excel query was used to systematically detect deviations from the SOP in each log file. The total error count per participant was calculated without differentiating between error types. On average, participants made M = 0.5 errors (SD = 0.72, range = 0; 6), with higher values indicating lower performance accuracy.
Mental load was assessed using a secondary task paradigm, where participants pressed a "monitoring" button every 50 seconds. According to the secondary task paradigm, the frequency of secondary task execution (button presses) is inversely related to mental load, with more frequent button presses suggesting lower mental load. Device communication was facilitated via an Apache web server, which tracked the timestamps of each participant's button press. We used these timestamps to extract the number of secondary task executions throughout the experiment. On average, participants pressed the monitoring button M = 1.54 times (SD = 1.06, range = 0; 4).
3.4 Data analysis
All analyses were conducted using R (version 4.1.2, Bird Hippie)[83] and Rstudio(version 2023.6.0.421)[84]. To investigate relationships between inputs, emergent states, and outputs, correlation analyses were performed and tested for significance. Different types of correlation were applied based on the scale level of the variables, as outlined in Table 1.
Scale Level Variable 1 | Scale Level Variable 2 | Correlation Type |
Metric | Metric | Spearman's Rho |
Metric | Ordinal | Spearman's Rho |
Metric | Dichotomous | Point-biserial correlation |
Dichotomous | Dichotomous | Point-tetrachoric correlation |
Dichotomous | Ordinal | Biserial rang correlation |
The correlation analyses for metric-metric variable pairs were performed with Spearman's Rho since, in each case, at least one of the variables was not normally distributed.
Outliers, whether formally, visually, or statistically identified, were retained in the sample, as they are considered inherent in the sample and essential for the generalizability of results to real-world practice. This decision aligns with the objective of maintaining ecological validity and acknowledges the potential variability inherent in real-world scenarios.
4. Results
The complete correlation matrix can be found in the supplementary material (Table S2), along with a table of corresponding correlation types for each analysis (Table S3). Figure 5 shows a model with all significant correlations between inputs, emergent states, and outputs. Figure 6 displays a model with significant direct correlations between inputs and outputs.
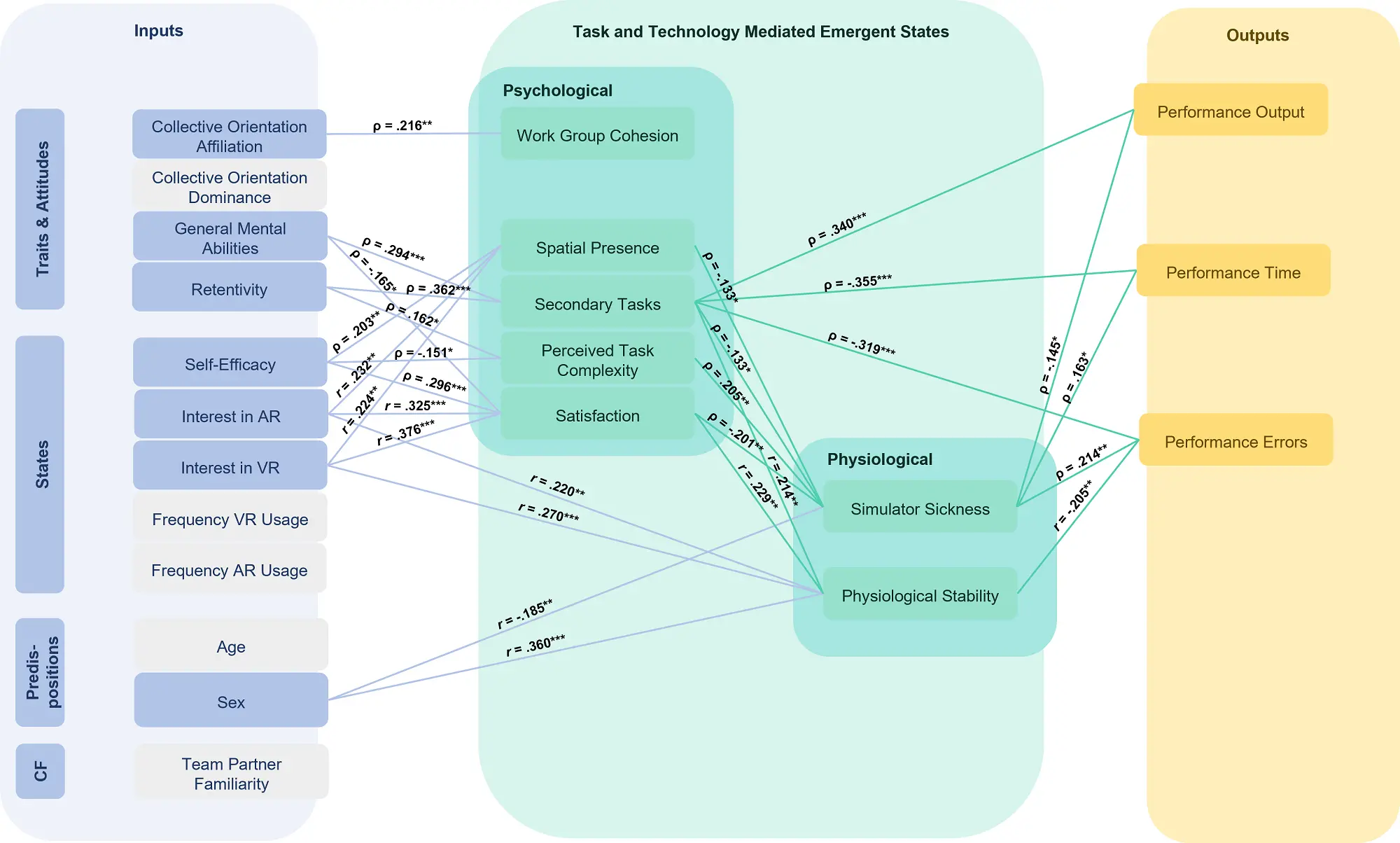
Figure 5. Significant correlations between inputs, emergent states, and outputs of the hypothesized IMO-model for AR-mediated remote collaboration, presented as ρ or r depending on the correlation analysis performed (Table 1). Secondary tasks were used to operationalize mental load (more secondary tasks = less mental load). The α -level was set at .05. p < .05*, p < .01**, p < .001*** Greyed-out input-variables were hypothesized to influence emergent states but yielded no statistically significant correlations in the analyses. CF: contextual factors. AR: augmented reality; VR: virtual reality.
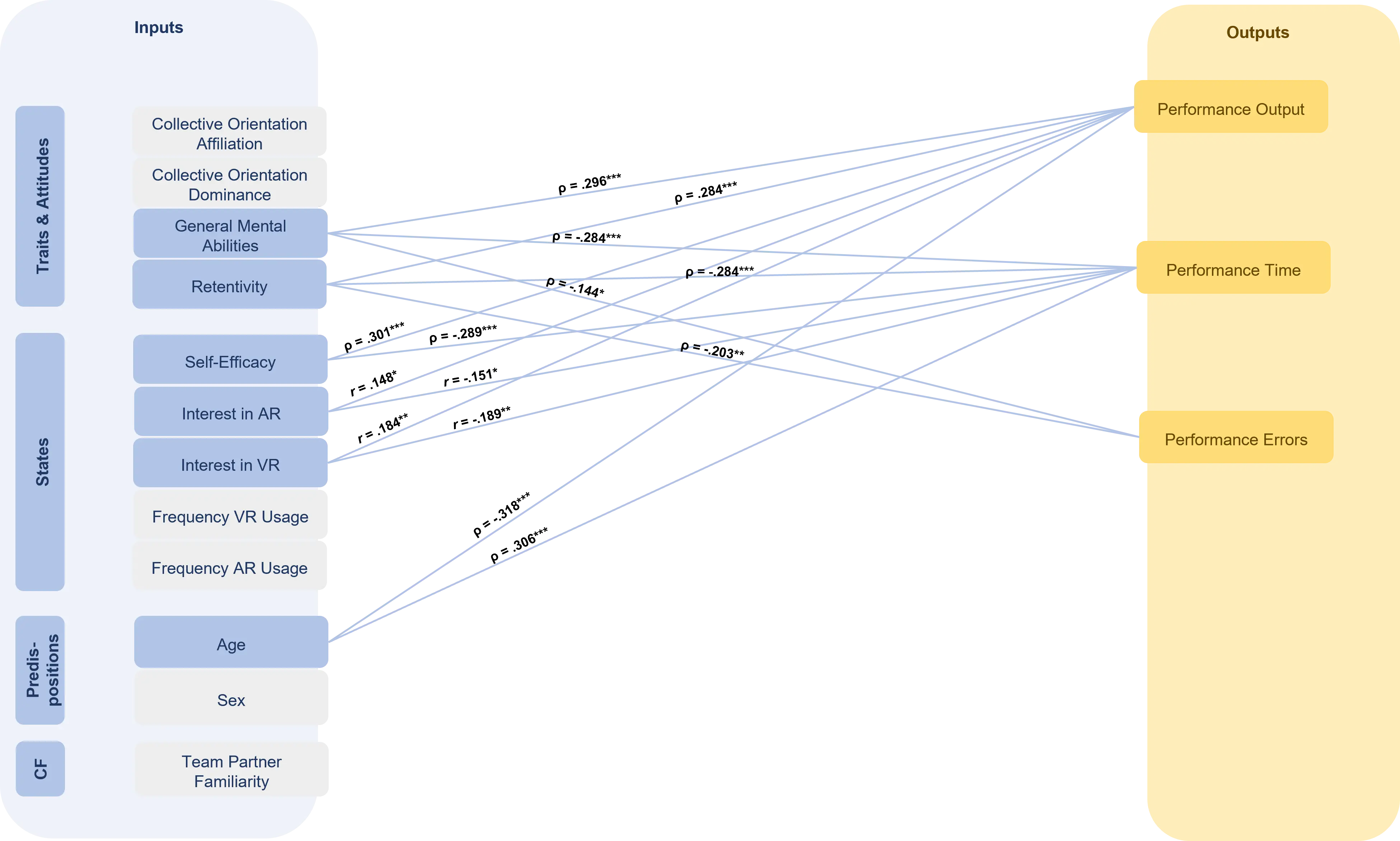
Figure 6. Significant correlations between inputs and outputs of the hypothesized IMO-model for AR-mediated remote collaboration, presented as ρ or r depending on the correlation analysis performed (cf. Table 1). Secondary tasks were used to operationalize mental load (more secondary tasks = less mental load). The α-level was set at .05. p < .05*, p < .01**, p < .001***. Greyed-out input-variables showed no significant direct correlations with the output-variables. CF: contextual factors; AR: augmented reality; VR: virtual reality.
Numerous significant correlations were found among the input, emergent state, and output variables, in addition to relationships between those variables. As these relationships were not part of the hypothesized IMO model, and a detailed analysis would exceed this article's scope, the results are provided in the supplementary material (Table S4,5,6). The following sections report significant correlation analyses results between:
• 4.1) inputs and emergent states,
• 4.2) psychological and physiological emergent states,
• 4.3) emergent states and outputs,
• 4.4) inputs and outputs.
4.1 Correlations between inputs and emergent states
Table 2 presents the results of the correlation analyses between inputs and emergent states.
Task and Technology Mediated Emergent States | |||||||||
Psychological | Psychological | ||||||||
Secondary Tasks | Perceived Task Complexity | Spatial Presence | Satisfaction | Work Group Cohesion | Simulator Sickness | Physiological Stability | |||
Inputs | Predis-positions | Age | ρ = -.013 | ρ = -.009 | ρ = -.106 | ρ = -.109, | ρ = .070 | ρ = -.035 | r = .035 |
Sex | r = .016 | r = -.111 | r = .028, | r = .080 | r = .067 | r = -.185** | r = .360*** | ||
Traits & Attitudes | General Mental Abilities | ρ = .294*** | ρ = .121 | ρ = .113 | ρ = -.165* | ρ = .101 | ρ = .016 | r = -.022 | |
Retentivity | ρ = .362*** | ρ = .162* | ρ = -.022 | ρ = -.060 | ρ = -.038 | ρ = .020 | r =-.004 | ||
Collective Orientation Affiliation | ρ = .047 | ρ = -.070 | ρ = .012 | ρ = .120 | ρ = .216** | ρ = -.106 | r = .080 | ||
Collective Orientation Dominance | ρ = .009 | ρ = -.001 | ρ = -.009 | ρ = -.107 | ρ = -.019 | ρ = -.052 | r = .042 | ||
States | Post-Training Self-Efficacy | ρ = .045 | ρ = -.151* | ρ = .203** | ρ = .296*** | ρ = -.074 | ρ = -.072 | r = -.081 | |
Interest AR | r = -.008 | r = .023 | r = .232** | r = .325*** | r = - .017 | r = -.068 | r = .220** | ||
Interest VR | r = -.033 | r = .036 | r = .224** | r = .376*** | r = -.055 | r = -.075 | r = .270*** | ||
Frequency AR Use | ρ = -.032 | ρ = .083 | ρ = -.035 | ρ = .007 | ρ = -.079 | ρ = -.114 | ρ = .089 | ||
Frequency VR Use | ρ = .012 | ρ = -.006 | ρ = -.006 | ρ = .009 | ρ = .001 | ρ = -.084 | ρ =.128 | ||
CF | Team Partner Familiarity | r = -.028 | r = .040 | r = .046 | r = .110 | r = .104 | r = .025 | r = -.024 |
The α-level was set at .05; *p < .05, **p < .01, ***p < .001. Male sex was coded as 1, female sex was coded as 0, so positive correlations refer to relationships with males, and negative correlations to relationships with females. Secondary tasks were used to operationalize mental load (more secondary tasks = less mental load). Physiological stability was coded 1 = no change (stable) and 0 = change (unstable), so that positive correlations indicated an unchanged physiological state. AR: augmented reality; VR: virtual reality; CF: contextual factors.
4.1.1 Correlations between inputs and psychological emergent states
General mental abilities showed a moderate positive correlation with secondary tasks (ρ = .294, p < .001) and a weak negative correlation with satisfaction (ρ = -.165, p = .014). Thus, participants with higher general mental abilities experienced lower mental load but were less satisfied with working in the simulation environment.
Retentivity showed a moderate positive correlation with secondary tasks (ρ = .362, p < .001) and a weak positive correlation with task complexity (ρ = .162, p = .016), meaning that participants with higher retentivity perceived the task as more complex but also experienced less mental load.
Collective orientation affiliation and work group cohesion were weakly positively correlated (ρ = .216, p = .001), indicating that participants with higher collective orientation perceived greater work group cohesion.
Self-efficacy: Weak negative correlation with task complexity (ρ = -.151, p = .026), weak positive correlation with spatial presence (ρ = .203, p = .002), and moderately positively correlation with satisfaction (ρ = .296, p < .001). Thus, participants with higher self-efficacy expectations in relation to mastering the experimental task perceived higher satisfaction with working in the simulation environment, higher spatial presence, and less task complexity.
Interest in AR and VR were weakly positively correlated with spatial presence (AR: r = .232, p = .001; VR: r = .224, p = .001) and moderately positively correlated with satisfaction (AR: r = .325, p < .001; VR: r = .376, p < .001). Thus, participants interested in the topics of AR and/ or VR perceived higher satisfaction with working in the simulation environment and higher spatial presence throughout the experiment.
In summary, high general mental abilities correlated with low mental load and high satisfaction. Higher retentivity correlated with low mental load and higher task complexity. High affiliation (collective orientation) linked to high work group cohesion. High self-efficacy linked to low task complexity, high spatial presence, and high satisfaction. Interest in AR and/or VR linked to higher spatial presence and satisfaction.
4.1.2 Correlations between inputs and physiological emergent states
Sex showed a weak negative correlation with simulator sickness (r = -.185, p = .006) and a moderate positive correlation with physiological stability (r = .360, p < .001). Thus, male participants reported less simulator sickness and fewer perception of physiological changes due to AR use.
Interest in AR and VR were weakly positively correlated with physiological stability (AR: r = .220, p = .001; VR: r = .270, p < .001). Thus, participants interested in AR/VR were less likely to report AR-induced physiological changes.
In summary, male participants and those interested in AR/VR reported less simulator sickness and fewer perceptions of AR-induced physiological changes.
4.2 Correlations between psychological and physiological emergent states
Table 3 presents the results of the correlation analyses between psychological and physiological emergent states.
Psychological Emergent States | ||||||
Secondary Tasks | Perceived Task Complexity | Spatial Presence | Satisfaction | Work Group Cohesion | ||
Physiological Emergent States | Simulator Sickness | ρ = -.133* | ρ = .205** | ρ = -.133* | ρ = -.201** | ρ = -.036 |
Physiological Stability | r = .214** | r = -.029 | r = .057 | r = .229** | r = -.051 |
The α-level was set at .05; *p < .05, **p < .01, ***p < .001. Secondary tasks were used to operationalize mental load (more secondary tasks = less mental load). Physiological stability was coded 1 = no change (stable) and 0 = change (unstable), so that positive correlations indicated an unchanged physiological state.
Simulator sickness showed weak negative correlations with secondary tasks (ρ = -.133, p = .049), spatial presence (ρ = -.133, p = .049), and satisfaction (ρ = -.201, p = .003), as well as a weak positive correlation with perceived task complexity (ρ = .205, p = .002). Thus, participants higher in simulator sickness perceived less spatial presence and less satisfaction, higher task complexity, and higher mental load (measured by secondary tasks).
Physiological stability was weakly positively correlated with secondary tasks (r = .214, p = .001) and satisfaction (r = .229, p = .001). Thus, participants with an unchanged physiological constitution perceived less mental load and higher satisfaction.
In summary, participants experienced lower mental load and higher satisfaction when they perceived no physiological changes and low simulator sickness. Moreover, lower perceived task complexity and higher spatial presence were associated with lower simulator sickness.
4.3 Correlations between emergent states and outputs
Table 4 presents the results of the correlation analyses between psychological and physiological emergent states and outputs.
Emergent States | ||||||||
Psychological | Physiological | |||||||
Secondary Tasks | Perceived Task Complexity | Spatial Presence | Satisfaction | Work Group Cohesion | Simulator Sickness | Physiological Stability | ||
Outputs | Performance Output | ρ = .340*** | ρ = .047 | ρ = .057 | ρ =.093 | ρ = .045 | ρ = -.145* | r = .104 |
Performance Time | ρ = -.355*** | ρ = -.047 | ρ = -.105 | ρ =-.082 | ρ = -.068 | ρ = .163* | r = -.122 | |
Performance Errors | ρ = -.319*** | ρ = -.085 | ρ = -.063 | ρ = -.060 | ρ = -.040 | ρ = .214** | r = -.205** |
The α-level was set at .05; *p < .05, **p < .01, ***p < .001. Secondary tasks were used to operationalize mental load (more secondary tasks = less mental load). Physiological stability was coded 1 = no change (stable) and 0 = change (unstable), so that positive correlations indicated an unchanged physiological state.
Secondary tasks showed a moderate positive correlation with performance output (ρ = .340, p < .001) and moderate negative correlations with performance time and errors (time: ρ = -.355, p < .001; errors: ρ = -.319, p < .001). Thus, participants who perceived less mental load reached higher outputs, needed less performance time, and made fewer errors.
Simulator sickness was weakly negatively correlated with performance output (ρ = -.145, p = .032), and weakly positively correlated with performance time (ρ = .163, p = .015) and performance errors (ρ = .214, p = .001). Thus, participants who reported greater simulator sickness achieved less performance output, needed more performance time, and made more errors.
Physiological stability showed a weak negative correlation with performance errors (r = -.205, p = .002), indicating that participants who perceived no physiological change made fewer errors.
In summary, participants higher in mental load and simulator sickness achieved less performance output, needed more performance time, and made more errors. Furthermore, participants who perceived no physiological change made fewer errors.
4.4 Direct links between inputs and outputs
Table 5 displays direct correlations between inputs and outputs.
Outputs | |||||
Performance Output | Performance Time | Performance Errors | |||
Inputs | Predis-positions | Age | ρ = -.318*** | ρ = .306*** | ρ = .083 |
Sex | r = .102 | r = -.112 | r = -.039 | ||
Traits & Attitudes | General Mental Abilities | ρ = .296*** | ρ = -.284*** | ρ = -.144* | |
Retentivity | ρ = .284*** | ρ = -.284*** | ρ = -.203** | ||
Collective Orientation Affiliation | ρ = -.025 | ρ = -.034 | ρ = .009 | ||
Collective Orientation Dominance | ρ = -.061 | ρ = .072 | ρ = -.056 | ||
States | Post-Training Self-Efficacy | ρ = .301*** | ρ = -.289*** | ρ = -.055 | |
Interest AR | r = .148* | r = -.151* | r = -.106 | ||
Interest VR | r = .184** | r = -.189** | r = -.086 | ||
Frequency AR Use | ρ = -.042 | ρ = .037 | ρ = -.025 | ||
Frequency VR Use | ρ = .118 | ρ = -.105 | ρ = -.057 | ||
CF | Team Partner Familiarity | r = .129 | r = -.127 | r = -.106 |
The α-level was set at .05; *p < .05, **p < .01, ***p < .001. Male sex was coded as 1, female sex was coded as 0, so positive correlations refer to relationships with males, and negative correlations to relationships with females. AR: augmented reality; VR: virtual reality; CF = contextual factors.
Performance output showed a moderate negative correlation with age (ρ = -.318, p < .001), as well as weak to moderate positive correlations with general mental abilities (ρ = .296, p < .001), retentivity (ρ = .284, p < .001), self-efficacy (ρ = .301, p < .001), and interest in AR (r = .148, p = .029) and VR (r = .184, p = .006). Thus, higher performance output was associated with higher general mental abilities, higher retentivity, higher self-efficacy, as well as interest in AR and/or VR. Furthermore, older participants achieved less performance output.
Performance time showed a moderate positive correlation with age (ρ = .306, p < .001), as well as weak to moderate negative correlations with general mental abilities (ρ = -.284, p < .001), retentivity (ρ = -.284, p < .001), self-efficacy (ρ = -.289, p < .001), and interest in AR (r = -.151, p = .026) and VR (r = -.189, p = .005). Thus, shorter performance time was associated with higher general mental abilities, higher retentivity, higher self-efficacy, interest in AR and/or VR, and younger age.
Performance errors were weakly negatively correlated with general mental abilities (ρ = -.144, p = .033) and retentivity (ρ = -.203, p = .002). Thus, participants with higher general mental abilities and retentivity made fewer errors.
In summary, younger age, high general mental abilities, high retentivity, high self-efficacy, and interest in AR and/or VR were associated with higher performance outputs and lower task execution time. Moreover, higher general mental abilities and higher retentivity were linked to fewer errors.
4.5 Summary of the results
Our findings suggest that the hypothesized input-variables age, collective orientation dominance, frequency of AR and VR use, and team partner familiarity, had no significant effects on task- and technology-mediated psychological and physiological emergent states. General mental abilities, retentivity, self-efficacy, and interest in AR/VR were linked to psychological emergent states, while sex and interest in AR/VR were (additionally) associated with physiological emergent states.
Except for work group cohesion, psychological and physiological emergent states were interconnected. Simulator sickness was linked with all psychological states (except for work group cohesion), while physiological stability was only associated with mental load and satisfaction.
Among psychological emergent states, only mental load was associated with output-variables (performance output, time, and errors). Simulator sickness showed links to all output-variables, while physiological stability was only associated with performance errors.
Additionally, there were direct correlations between inputs and outputs. Performance output and time were associated with age, general mental abilities, retentivity, self-efficacy, and interest in AR/VR. Performance errors were associated with general mental abilities and retentivity.
Figure 7 illustrates the hypothesized IMO-model for AR-mediated remote collaboration corresponding to the results.
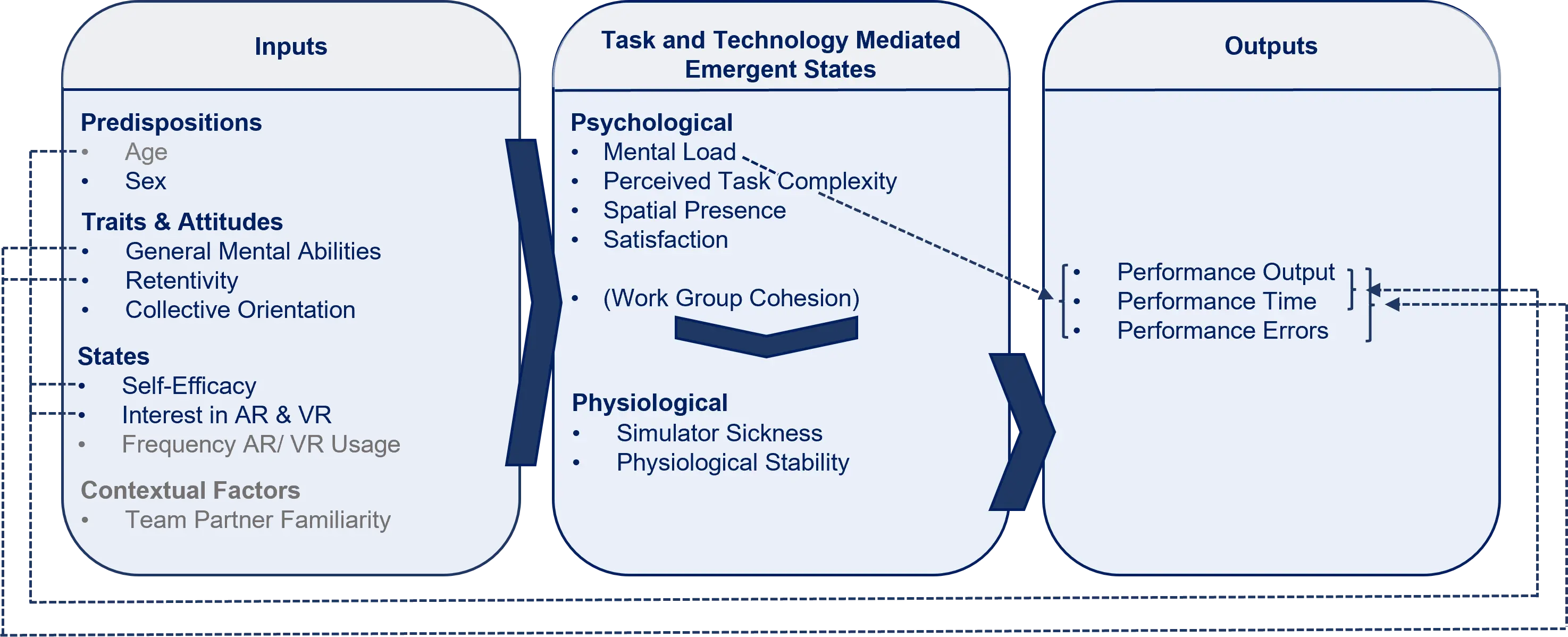
Figure 7. Result-based Input-Mediator-Output model for AR-mediated remote collaboration . Greyed-out inputs yielded no statistically significant correlations with any task- and technology-mediated emergent state. Work group cohesion is depicted in brackets as it was influenced by inputs but had no effects on outputs. Dashed arrows illustrate direct influences. AR: augmented reality; VR: virtual reality.
5. Discussion
Our results confirm that person-related inputs significantly influence emergent states and performance outcomes in AR-mediated remote collaboration. Beyond task- and technology-related effects, individual differences play a crucial role in shaping collaboration experiences and performance. These findings underscore the importance of designing AR tools that align with user characteristics and lay the groundwork for future IMO research in this field.
Our results indicate that person-related inputs affect psychological emergent states, which in turn influence physiological emergent states, which finally influence performance-related outputs. Additionally, direct associations were found between person-related inputs and performance-related outputs. In this section, we discuss our results in relation to the proposed relationships within the IMO-model (I-M and M-O relationships) and the direct links between inputs and outputs (I-O relationships).
5.1. Relationships between person-related input variables and psychological and physiological emergent states
Our results suggest that higher retentivity and general mental abilities are linked to lower mental load, indicating a buffer-function for negative effects on psychological well-being, as high mental load is linked to adverse health-related outcomes[53]. These findings are noteworthy given the limited research on the influence of person-related variables on mental load[85]. Moreover, they highlight the importance of controlling task- and technology-induced mental load, as high levels along with lower general mental abilities and retentivity could lead to mental overload.
Furthermore, we found that participants with higher self-efficacy in mastering the experimental task exhibited higher satisfaction, greater spatial presence, and perceived lower task complexity in the simulation environment. These results underline the importance of adequate training, as self-efficacy is largely influenced by how well individuals feel prepared to master a (novel or upcoming) task or situation. Thus, enhancing self-efficacy leads to a more positive experience during AR-mediated remote collaboration, improving satisfaction, spatial presence, and task complexity, resulting in enhanced psychological well-being.
Moreover, being interested in AR/VR showed positive associations with spatial presence and satisfaction. These results align with Wirth et al.'s[46] model of spatial presence, highlighting the advantages of intrinsic interest in AR/VR for enhancing experience quality during AR-mediated remote collaboration. Additionally, interest in AR/VR was linked to physiological stability throughout AR use. This might be explained by an 'expectancy-experience' match, where participants interested in AR/VR assumed, or had experienced, that they were not susceptible to AR-induced physiological changes, possibly as a positive self-fulfilling prophecy. Therefore, increasing interest in AR/VR among employees could be an easy, low-threshold strategy to enhance their experience during AR-mediated remote collaboration, improving both psychological and physiological well-being.
In line with VR-research, our results showed a link between being female and higher simulator sickness, as well as AR-induced physiological changes. It remains unclear whether men actually experience fewer symptoms or simply report discomfort less often, for example due to socialization or tolerance thresholds[86,87]. This would align with Galdas et al.[88], who found that men seek delayed help when they are sick due to 'masculine beliefs'. The same may apply to reporting simulator sickness or physiological changes in the context of AR-mediated remote collaboration. This finding emphasizes the importance of considering target group-specific differences in AR-task and technology design, to avoid disadvantaging certain user groups, such as female participants.
In line with the findings reviewed by Kilcullen et al.[40], we found a positive relation between collective orientation affiliation and work group cohesion. Interestingly, work group cohesion did not show further effects on physiological emergent states or outputs, challenging the assumed role of work group cohesion in the context of performance outcomes.
Surprisingly, participants with higher retentivity perceived the task as more complex, which seems counterintuitive since individuals with higher retentivity should theoretically retain information better, reducing perceived task complexity. However, it is possible that these individuals perceive tasks as more complex because they tend to recall a greater number of task-related details.
Participants with higher general mental abilities showed less satisfaction when working with the simulation, possibly due to their greater cognitive resources and attentional control, leading to a more critical or analytical evaluation, as their increased attention to detail might result in lower satisfaction. Additionally, these individuals may have different expectations or preferences regarding characteristics and complexity and unmet expectations could negatively impact satisfaction. These findings suggest that task and technology design should consider the levels of general mental abilities and retentivity in the target user group.
5.2. Relationships between psychological and physiological emergent states
Except for work group cohesion, all tested psychological emergent states showed relationships with simulator sickness. Higher simulator sickness was linked to reduced spatial presence and satisfaction, with the latter also associated with perceived physiological changes. This contradicts VR-related research suggesting a trade-off between presence and simulator sickness[89]. Our results indicate that AR tools should aim to maximize spatial presence and satisfaction to prevent simulator sickness.
Consistent with prior research, we found that complex AR scenarios can induce severe simulator sickness[74], as higher perceived task complexity and mental load were associated with higher simulator sickness. These findings highlight the need for task and technology designs that minimize complexity and mental load and underscore the importance of customizable technologies to accommodate individual differences. As demonstrated, besides the link to simulator sickness, perceived task complexity showed relations to self-efficacy and retentivity, and mental load to retentivity and general mental abilities. Given these individual differences, customizable technologies would be desirable to offer individually tailored support.
5.3. Relationships between emergent states and performance-related output variables
Our results highlight the benefits of low mental load and low simulator sickness on performance: participants with lower levels of either achieved higher performance output, completed tasks faster, and made fewer errors. Additionally, physiological stability was linked to fewer errors, indicating higher accuracy.
These findings emphasize the importance of careful technology and task design, as technology-related factors are known to potentially increase simulator sickness[67], while a suboptimal task design can increase the perception of task complexity[90]. Thus, poor design can increase simulator sickness and perceived task complexity, leading to serious consequences, especially when errors cause harm to people or the environment.
5.4. Relationships between person-related variables and performance-related output variables
Our results indicated several direct relationships between person-related inputs and performance-related outputs. Higher general mental abilities and retentivity were linked to higher performance output, shorter performance time, and fewer errors. These findings align with the work of Schmidt and Hunter[91] who identified general mental abilities as a strong predictor of job performance across domains, particularly in complex tasks that require rapid information processing, such as the AR-mediated interactions examined in this paper.
From a cognitive load perspective[92], individuals with higher retentivity are better equipped to manage intrinsic and extraneous cognitive load allowing them to allocate more cognitive resources to learning and performing tasks efficiently, leading to improved automation and schema acquisition. This aligns with Wickens' multiple resource theory[93], which suggests that efficient cognitive resource allocation enhances performance, particularly in multitasking environments, such as the AR-based remote task examined here.
Moreover, higher self-efficacy, younger age, and interest in AR/VR were linked to higher performance outputs and shorter performance time.
Self-efficacy and better performance: This finding aligns well with Bandura's self-efficacy theory[94], which suggests that individuals who believe in their capabilities are more likely to exert effort and persist in challenging tasks. Thus, higher self-efficacy may not only foster confidence in immersive environments but also encourage proactive problem-solving, which are key drivers of enhanced performance[95]. Moreover, studies from the field of gaming suggest that higher self-efficacy is related to better in-game performance[96] and task completion[97]. These findings suggest that self-efficacy is not only a crucial predictor of success in digital environments but also a key factor in optimizing user engagement and performance in AR-mediated tasks.
Younger age and better performance: Our findings indicate a direct effect of age on performance output and time. Specifically younger participants achieved higher performance outputs and completed tasks more quickly. This aligns with previous findings that younger individuals generally exhibit better performance in technology-mediated tasks, potentially due to higher digital literacy and quicker adaptation[12,28]. The latter might be based on age-related declines in several cognitive skills, such as motor responses, spatial awareness, and overall cognitive processing speed, especially in time-critical tasks[98,99]. These findings highlight the importance of designing systems that account for age-related cognitive differences to ensure usability and accessibility across diverse age groups.
Interest in AR/VR and better performance: Participants with a pre-existing interest in AR/VR demonstrated higher performance outputs and shorter completion times. This finding might be explained by the intrinsic motivation theory[100], which posits that interest and enjoyment in a task enhance engagement and effort, leading to superior performance outcomes. This suggests that fostering user interest in AR/VR through engaging onboarding experiences or prior exposure could significantly enhance user performance and technology adoption in professional contexts. Organizations might consider early-stage training or gamification elements to increase intrinsic motivation and performance outcomes.
Taken together, the findings show important implications for designing efficient AR tools for remote collaboration and for organizational training and human resource development. AR tools, used to enhance productivity and effectiveness, should be individually tailored or adaptable to different target groups, as they need to accommodate varying levels of general mental abilities and retentivity. Moreover, we showed that younger participants performed better. This finding once more emphasizes the need for customizable interfaces that allow users to select, e.g., specific support levels. Inadequate user demand fulfillment can lead to over- or under-demanding, both of which can have far-reaching negative consequences. For human resource development, fostering interest in AR/VR and enhancing self-efficacy through targeted training can improve performance. This underscores the importance of self-efficacy-oriented training and creating opportunities to stimulate interest in AR/VR.
5.5. Additional findings
In addition to the significant results discussed, we found that age did not influence the emergent states. This result may be surprising given previous research suggesting age-related differences in cognitive and physiological responses to AR environments[12,27,28]. Since we observed a direct effect of age on performance, we assume that distinct cognitive mechanisms may underlie these effects, mechanisms that were not directly measured in our study. Future research should investigate potential mediating factors, such as cognitive flexibility or attentional control, to better understand how age influences performance in AR-mediated remote collaboration.
Moreover, we found that frequency of AR/VR usage did not significantly affect psychological or physiological emergent states or performance. This lack of effect could be attributed to the relatively low frequency of AR/VR usage among our participants, leading to insufficient variance to detect meaningful relationships. The low usage frequency may not provide enough exposure to induce significant adaptation effects, which are necessary for noticeable impacts on cognitive and physiological states during AR usage. Future studies should consider sampling participants with a higher frequency of AR/VR usage to explore potential effects more thoroughly.
Finally, team partner familiarity also showed no significant relationships with psychological or physiological emergent states or performance outcomes. This result contrasts with some previous findings suggesting that familiarity with team partners can enhance trust and coordination, thereby improving performance[48,49]. One possible explanation for this discrepancy is the controlled experimental setup, which may have minimized the influence of familiarity by providing clear task guidelines and structured procedures. In such environments, the advantages of familiarity may be less pronounced. Additionally, participants may have quickly adapted to working with unfamiliar partners, compensating for the lack of prior familiarity. Another potential reason for the non-significant effect is the spatial separation of team members in our study. Since participants could neither see nor directly communicate with their partners, typical benefits of familiarity, such as improved coordination and shared mental models, may have been diminished. Future research should examine how communication modalities and task structure moderate the effects of team partner familiarity in remote AR collaboration settings.
6. Limitations
While our results offer valuable insights into the relationships among person-related input variables, psychological and physiological emergent states, and performance-related output variables in AR-mediated remote collaboration, several limitations should be noted.
Firstly, our participant age distribution skewed younger, which may limit the generalizability of our findings to older populations. Future research should include a more diverse age range to better understand age-related differences.
Secondly, most observed correlations were weak to moderate. While informative, these correlations may not fully capture the complexity of the relationships, suggesting other factors not examined may contribute to the observed outcomes. Future studies should explore additional variables or use more advanced statistical techniques to clarify underlying mechanisms driving the observed associations.
Thirdly, our focus on individual-level variables overlooked contextual factors like organizational culture, team characteristics and dynamics, task characteristics, and technological features. Future research should incorporate these factors for a more comprehensive understanding of AR-mediated remote collaboration dynamics.
Fourthly, one key limitation of this study is its focus on dyadic collaboration, whereas real-world collaborative work often involves larger teams. In larger team settings, additional dynamics such as coordination complexities may influence the relationships observed in this study. Future research should explore how person-related inputs and emergent states function in multi-member teams to enhance the generalizability of individual-level influence in AR-mediated remote collaboration, offering valuable insights for further research on more complex team structures.
Additionally, the correlational nature of our study design prevents causal inferences. While we identified significant associations among variables, we cannot ascertain the directionality or rule out unmeasured confounding variables. Experimental or longitudinal studies are needed to establish causal pathways and understand the temporal dynamics of the relationships studied.
Lastly, our study exclusively examined AR-mediated remote collaboration, limiting generalizability to other remote collaboration forms or technological platforms. Different technologies, such as VR or Mixed Reality, may elicit distinct relationship patterns. Future research should explore these relationships across diverse technological contexts to better understand human factors in remote collaboration.
7. Conclusion
This study provides evidence that individual differences significantly impact psychological and physiological emergent states in AR-mediated remote collaboration. Factors such as general mental abilities, self-efficacy, and interest in AR/VR influenced subjective experiences, perceptions, and physiological responses. Higher levels of these variables were generally associated with positive psychological outcomes, such as lower mental load and greater satisfaction.
We identified a clear I-M-O chain, where person-related variables exerted cascading effects on emergent states, which in turn influenced performance-related outcomes. This underscores the need for integrated approaches in designing AR tools for remote collaboration, taking individual characteristics into account to promote well-being.
Understanding these mutual influences is crucial for human-centered AR tool design, especially for organizational implementation to promote employee well-being. As AR tools for remote collaboration continue to develop, our findings can guide early-stage design processes and help organizations implement appropriate human resource measures to protect employees from AR-induced harm.
Our study also suggests future research directions. Replication studies are essential to validate our results and explore potential mediating or moderating roles of emergent states in the I-M-O chain. Integrating existing research on task- and technology-mediated effects will further elucidate their influence on emergent states and outputs.
This study presents a significant step in IMO research for AR-mediated remote collaboration by developing a comprehensive model that offers practical guidelines for creating health-promoting AR tools.
In conclusion, our study provides a foundational framework for understanding interactions among individuals, tasks, and technology in AR-mediated collaboration. Continued research and application of our model can lead to more effective and supportive AR tools, improving user experience and outcomes in various AR-mediated remote work environments.
Supplementary material
The supplementary material for this article is available at: Supplementary materials.
Acknowledgements
Our cordially thanks go to our student assistants for their tireless acquisition of test persons as well as for the support during the data collection process. This article is partially based on research conducted as part of Lisa Thomaschewski's doctoral thesis.
Authors contribution
Thomaschewski L: Conceptualization, methodology, formal analysis, investigation, data curation, writing-original draft, writing-review & editing, visualization, project administration.
Weyers B: Software, resources, writing-reviews & editing, supervision, funding acquisition.
Kluge A: Conceptualization, resources, writing-review & editing, supervision, funding acquisition.
Conflicts of interest
The authors declare no conflicts of interest.
Ethics approval
This study was approved by the local ethics committee of the Ruhr University Bochum (No. 440).
Consent to participate
All participants provided written informed consent before taking part in the study. They were fully informed about the study's purposes, procedures, potential risks, and benefits, both verbally and in writing.
Consent for publication
Not applicable.
Availability of data and materials
The data and materials could be obtained from the corresponding author upon request.
Funding
This work was supported by the DFG (Deutsche Forschungsgemeinschaft) [grant numbers KL2207/7-1, WE5408/3-1].
Copyright
© The Author(s) 2025.
References
-
1. Belen RAJ, Nguyen H, Filonik D, Del Favero, Bednarz T. A systematic review of the current state of collaborative mixed reality technologies:2013-2018. AIMS Electron Electr Eng. 2019;3(2):181-223.
[DOI] -
2. Marques B, Teixeira A, Silva S, Alves J, Dias P, Santos BS. A critical analysis on remote collaboration mediated by Augmented Reality: Making a case for improved characterization and evaluation of the collaborative process. Comput Graph. 2022;102:619-633.
[DOI] -
3. Airbus [Internet]. Mixed Reality to meet future challenges. 2023 Jun 21 [cited 2024 Mar 25]. Available from: https://www.airbus.com/en/newsroom/stories/2023-06-mixed-reality-to-meet-future-challenges
-
4. SightCall [Internet]. Accenture and SightCall help Airbus improve productivity. Available from: https://sightcall.com/de/blog/sightcall-airbus-video-technology
-
5. Henkel [Internet]. How Augmented Reality (AR) glasses are providing instant support for line management in the surface treatment. Available from: https://henkel-adhesives.com/de/en/industries/metals/metal-pretreatment.html
-
6. Jeffri NFS, Awang Rambli. A review of augmented reality systems and their effects on mental workload and task performance. Heliyon. 2021;7(3):e06277.
[DOI] -
7. Sacau A, Laarni J, Hartmann T. Influence of individual factors on presence. Comput Hum Behav. 2008;24(5):2255-2273.
[DOI] -
8. Smyth J, Birrell S, Mouzakitis A, Jennings P. Motion Sickness and Human Performance-Exploring the Impact of Driving Simulator User Trials. In: Stanton N, editor. Advances in Human Aspects of Transportation. Proceedings of the AHFE 2018 International Conference on Human Factors in Transportation; 2018 Jul 21-25; Florida, USA. Cham: Springer; 2018. p. 445-457.
[DOI] -
9. Gaines BR. Modeling and forecasting the information sciences. Inf Sci. 1991;57-58:3-22.
[DOI] -
10. Georganta E, Peus C, Niess J. Interactive technologies through the lens of team effectiveness: an interdisciplinary systematic literature review. Eur J Work Organ Psychol. 2023;10(4):172-187.
[DOI] -
11. Vovk A, Wild F, Guest W, Kuula T. Simulator Sickness in Augmented Reality Training Using the Microsoft HoloLens. In: Mandryk M, Hancock M, editors. Proceedings of the 2018 CHI Conference on Human Factors in Computing Systems; 2018 Apr 21-26; Montreal, Canada. New York: Association for Computing Machinery; 2018. p. 1-9.
[DOI] -
12. Lee LN, Kim MJ, Hwang WJ. Potential of Augmented Reality and Virtual Reality Technologies to Promote Wellbeing in Older Adults. Appl Sci. 2019;9(17):3556.
[DOI] -
13. Sackett PR, Lievens F, van Iddekinge, Kuncel NR. Individual differences and their measurement: A review of 100 years of research. J Appl Psychol. 2017;102(3):254-273.
[DOI] -
14. Hsu MH, Ju TL, Yen CH, Chang CM. Knowledge sharing behavior in virtual communities: The relationship between trust, self-efficacy, and outcome expectations. Int J Hum-Comput Stud. 2007;65(2):153-169.
[DOI] -
15. Compeau DR, Higgins CA. Computer Self-Efficacy: Development of a Measure and Initial Test. MIS Q. 1995;19(2):189.
[DOI] -
16. Chiu CM, Hsu MH, Wang ET. Understanding knowledge sharing in virtual communities: An integration of social capital and social cognitive theories. Decis Support Syst. 2006;42(3):1872-1888.
[DOI] -
17. Eason K. How to Fail When Introducing Electronic Technologies into Organisations. In: 2016 9th International Conference on Developments in eSystems Engineering (DeSE); 2016 Aug 31-2016 Sep 2; Liverpool, United Kingdom. Piscataway: IEEE; 2016. p. 3-6.
[DOI] -
18. Thomaschewski L, Feld N, Weyers B, Kluge A. The Use of Augmented Reality for Temporal Coordination in Everyday Work Context. In: Simeone A, Weyers B, Bialkova S, Lindeman RW, editors. Everyday Virtual and Augmented Reality. Human-Computer Interaction Series. Cham: Springer; 2023. p. 57-87.
[DOI] -
19. Mathieu JE, Maynard MT, Rapp T, Gilson L. Team Effectiveness 1997-2007: A Review of Recent Advancements and a Glimpse Into the Future. J Manag. 2008;34(3):410-476.
[DOI] -
20. Maynard MT, Kennedy DM, Sommer SA. Team adaptation: A fifteen-year synthesis (1998–2013) and framework for how this literature needs to "adapt" going forward. Eur J Work Organ Psychol. 2015;24(5):652-677.
[DOI] -
21. Ilgen DR, Hollenbeck JR, Johnson M, Jundt D. Teams in organizations: from input-process-output models to IMOI models. Annu Rev Psychol. 2005;56:517-543.
[DOI] -
22. Dulebohn JH, Hoch JE. Virtual teams in organizations. Hum Resour Manag Rev. 2017;27(4):569-574.
[DOI] -
23. Klostermann M, Ontrup G, Thomaschewski L, Kluge A. Something Old or Something New? Z Arb Org Psychol. 2021;65(4):215-230.
[DOI] -
24. Marlow SL, Lacerenza CN, Salas E. Communication in virtual teams: a conceptual framework and research agenda. Hum Resour Manag Rev. 2017;27(4):575-589.
[DOI] -
25. Kanse L, Stephenson EK, Klonek FE, Wee S. Interdependence in Virtual Teams—A Double-Edged Sword? Small Group Res. 2023.
[DOI] -
26. Geiser C, Götz T, Preckel F, Freund PA. States and Traits. Eur J Psychol Assess. 2017;33(4):219-223.
[DOI] -
27. Golding JF. Motion sickness susceptibility. Auton Neurosci Basic Clin. 2006;129(1):67-76.
[DOI] -
28. Mittelstaedt JM. Individual predictors of the susceptibility for motion-related sickness: A systematic review. J Vestib Res. 2020;30(3):165-193.
[DOI] -
29. Howard MC, van Zandt EC. A meta-analysis of the virtual reality problem: Unequal effects of virtual reality sickness across individual differences. Virt Real. 2021;25(4):1221-1246.
[DOI] -
30. Munafo J, Diedrick M, Stoffregen TA. The virtual reality head-mounted display Oculus Rift induces motion sickness and is sexist in its effects. Exp Brain Res. 2017;235(3):889-901.
[DOI] -
31. Bend J, Öörni A. Effects of Augmented Reality on Visuospatial Abilities of Males and Females. In: García Márquez FP, Jamil A, Ramirez IS, Eken S, Hameed AA, editors. Computing, Internet of Things and Data Analytics. Cham: Springer; 2024. p. 122-131.
[DOI] -
32. Gonzalez-Mulé E, Mount MK, Oh , IS . A meta-analysis of the relationship between general mental ability and nontask performance. J Appl Psychol. 2014;99(6):1222-1243.
[DOI] -
33. Lang JWB, Kersting M, Hulsheger UR, Lang J. General Mental Ability, Narrower Cognitive Abilities, and Job Performance: The Perspective of the Nested-Factors Model of Cognitive Abilities. Pers Psychol. 2010;63(3):595-640.
[DOI] -
34. Ree MJ, Earles JA. Intelligence Is the Best Predictor of Job Performance. Curr Dir Psychol Sci. 1992;1(3):86-89.
[DOI] -
35. Salgado JF, Anderson N, Moscoso S, Bertua C, de Fruyt, Rolland JP. A meta-analytic study of general mental ability validity for different occupations in the European community. J Appl Psychol. 2003;88(6):1068-1081.
[DOI] -
36. Kluge A, Silbert M, Wiemers US, Frank B, Wolf OT. Retention of a standard operating procedure under the influence of social stress and refresher training in a simulated process control task. Ergonomics. 2019;62(3):361-375.
[DOI] -
37. Driskell JE, Salas E, Hughes S. Collective orientation and team performance: development of an individual differences measure. Hum Factors. 2010;52(2):316-328.
[DOI] -
38. Driskell JE, Salas E. Collective Behavior and Team Performance. Hum Factors. 1992;34(3):277-288.
[DOI] -
39. Hagemann V, Ontrup G, Kluge A. Collective orientation and its implications for coordination and team performance in interdependent work contexts. Team Perform Manag. 2021;27(1/2):30-65.
[DOI] -
40. Kilcullen M, Bisbey TM, Rosen M, Salas E. Does team orientation matter? A state-of-the-science review, meta-analysis, and multilevel framework. J Organ Behav. 2023;44(2):355-375.
[DOI] -
41. Bandura A, Adams NE. Analysis of self-efficacy theory of behavioral change. Cogn Ther Res. 1977;1(4):287-310.
[DOI] -
42. Cherian J, Jacob J. Impact of Self Efficacy on Motivation and Performance of Employees. Int J Bus Manag. 2013;8(14):80-88.
[DOI] -
43. Bisschop MI, Kriegsman DMW, Beekman ATF, Deeg DJH. Chronic diseases and depression: the modifying role of psychosocial resources. Soc Sci Med. 2004;59(4):721-733.
[DOI] -
44. Caroli MED, Sagone E. Generalized Self-efficacy and Well-being in Adolescents with High vs. Low Scholastic Self-efficacy. Procedia Soc Behav Sci. 2014;141(2):867-874.
[DOI] -
45. Mousavi SMA, Powell W, Louwerse MM, Hendrickson AT. Behavior and self-efficacy modulate learning in virtual reality simulations for training: a structural equation modeling approach. Front Virtual Real. 2023;4:1658.
[DOI] -
46. Wirth W, Hartmann T, Böcking S, Vorderer P, Klimmt C, Schramm H, et al. A Process Model of the Formation of Spatial Presence Experiences. Media Psychol. 2007;9(3):493-525.
[DOI] -
47. Dużmanska N, Strojny P, Strojny A. Can Simulator Sickness Be Avoided? A Review on Temporal Aspects of Simulator Sickness. Front Psychol. 2018;9:2132.
[DOI] -
48. Morrison-Smith S, Ruiz J. Challenges and barriers in virtual teams: a literature review. SN Appl Sci. 2020;2(6):1-33.
[DOI] -
49. Jong BA, Dirks KT, Gillespie N. Trust and team performance: A meta-analysis of main effects, moderators, and covariates. J Appl Psychol. 2016;101(8):1134-1150.
[DOI] -
50. Ravaja N, Saari T, Turpeinen M, Laarni J, Salminen M, Kivikangas M. Spatial Presence and Emotions during Video Game Playing: Does It Matter with Whom You Play? Presence Teleoper Virtual Environ. 2006;15(4):381-392.
[DOI] -
51. Quinones MA, Ford JK, Teachout MS. The relationshio between work experience and job performance: A conceptual and meta-analytic review. Pers Psychol. 1995;48(4):887-910.
[DOI] -
52. Albers MJ. Tapping as a measure of cognitive load and website usability. In: Protopsaltis A, Spyratos N, editors. Proceedings of the 29th ACM international conference on Design of communication; 2011 Oct 3-5; Pisa, Italy. New York: Association for Computing Machinery. 2011. p. 25-32.
[DOI] -
53. Fallahi M, Motamedzade M, Heidarimoghadam R, Soltanian AR, Miyake S. Effects of mental workload on physiological and subjective responses during traffic density monitoring: A field study. Appl Ergonom. 2016;52:95-103.
[DOI] -
54. Maynard DC, Hakel , MD . Effects of Objective and Subjective Task Complexity on Performance. Hum. Perform. 1997;10(4):303-330.
[DOI] -
55. Main LC, Wolkow A, Chambers TP. Quantifying the Physiological Stress Response to Simulated Maritime Pilotage Tasks: The Influence of Task Complexity and Pilot Experience. J Occup Environ Med. 2017;59(11):1078-1083.
[DOI] -
56. Tremblay ML, Rethans JJ, Dolmans D. Task complexity and cognitive load in simulation-based education: A randomised trial. Med Educ. 2023;57(2):161-169.
[DOI] -
57. Slater M, Lotto B, Arnold MM, Sanchez-Vives M. How we experience immersive virtual environments: the conpet of presence and its measurement. Anu Psicol. 2009;40(2):193-210. Available from: https://www.researchgate.net/publication/228751198
-
58. Frank B, Kluge A. Development and first validation of the Presence Scale (PLBMR) for lab-based microworld research. In: Felnhofer A, Kothgassner OD, editors. Challenging Presence: Proceedings of the International Society for Presence Research; 2014. p. 31-42.
-
59. Sas C, O'Hare GMP, Reilly R. Presence and task performance: an approach in the light of cognitive style. Cogn Tech Work. 2004;6(1):53-56.
[DOI] -
60. Nash EB, Edwards GW, Thompson JA, Barfield W. A Review of Presence and Performance in Virtual Environments. Int J Hum-Comput Interact. 2000;12(1):1-41.
[DOI] -
61. Maneuvrier A, Decker LM, Ceyte H, Fleury P, Renaud P. Presence Promotes Performance on a Virtual Spatial Cognition Task: Impact of Human Factors on Virtual Reality Assessment. Front Virtual Real. 2020;1:619.
[DOI] -
62. Kosa M, Uysal A. Effects of Presence and Physical Activity on Player Well-being in Augmented Reality Games: A Diary Study. Int J Hum-Comput Interact. 2022;38(1):93-101.
[DOI] -
63. Behm-Morawitz E. Mirrored selves: The influence of self-presence in a virtual world on health, appearance, and well-being. Comput Hum Behav. 2013;29(1):119-128.
[DOI] -
64. Riordan CM, Weatherly EW. Defining and Measuring Employees' Identification with Their Work Groups. Educ Psychol Meas. 1999;59(2):310-324.
[DOI] -
65. Grossman R, Nolan K, Rosch Z, Mazer D. The team cohesion-performance relationship: A meta-analysis exploring measurement approaches and the changing team landscape. Organ Psychol Rev. 2022;12(2):181-238.
[DOI] -
66. Chardonnet JR, Mirzaei MA, Mérienne F. Features of the Postural Sway Signal as Indicators to Estimate and Predict Visually Induced Motion Sickness in Virtual Reality. Int J Hum-Comput Interact. 2017;33(10):771-785.
[DOI] -
67. Rebenitsch L, Owen C. Review on cybersickness in applications and visual displays. Virt Real. 2016;20(2):101-125.
[DOI] -
68. Jerome C, Darnell R, Oakley B, Pepe A. The Effects of Presence and Time of Exposure on Simulator Sickness. In: Proceedings of the Human Factors and Ergonomics Society Annual Meeting. Thousand Oaks: SAGE Publications; 2005. p. 2258-2262.
[DOI] -
69. Ciążyńska J, Janowski M, Maciaszek J. Effects of a Modern Virtual Reality 3D Head-Mounted Display Exergame on Simulator Sickness and Immersion Under Specific Conditions in Young Women and Men: Experimental Study. JMIR Serious Games. 2022;10(4):e41234.
[DOI] -
70. Saredakis D, Szpak A, Birckhead B, Keage HAD, Rizzo A, Loetscher T. Factors Associated With Virtual Reality Sickness in Head-Mounted Displays: A Systematic Review and Meta-Analysis. Front Hum Neurosci. 2020;14:96.
[DOI] -
71. Hussain M, Park J, Kim HK. Augmented reality sickness questionnaire (ARSQ): A refined questionnaire for augmented reality environment. Int J Ind Ergonom. 2023;97:103495.
[DOI] -
72. Nelson WT, Roe MM, Bolia RS, Morley RM. Assessing Simulator Sickness in a See-Through HMD: Effects of Time Delay, Time on Task, and Task Complexity. In: IMAGE 2000 Conference; 2000; Scottsdale, Arizona. California: SAGE Publications; 2000. Available from: https://www.researchgate.net/profile/Robert-Bolia/publication/235186544.pdf
-
73. Hughes CL, Fidopiastis C, Stanney KM, Bailey PS, Ruiz E. The Psychometrics of Cybersickness in Augmented Reality. Front Virtual Real. 2020;1:55.
[DOI] -
74. Kaufeld M, Mundt M, Forst S, Hecht H. Optical see-through augmented reality can induce severe motion sickness. Displays. 2022;74(11):102283.
[DOI] -
75. Schönbrodt F, Gollwitzer M, Abele-Brehm A. Der Umgang mit Forschungsdaten im Fach Psychologie: Konkretisierung der DFG-Leitlinien. Psychol Rundsch. 2017;68(1):20-35.
[DOI] -
76. Burkolter D, Kluge A, German S, Grauel B. Waste Water Treatment Simulation (WaTr Sim): Validation of a New Process Control Simulation Tool for Experimental Training Research. Proc Hum Factors Ergon Soc Annu Meet. 2009;53(26):1969-1973.
[DOI] -
77. Frank B, Kluge, A. Cued Recall with Gaze Guiding-Reduction of Human Errors with a Gaze-Guiding Tool. In: Hale K, Stanney K, editors. Advances in Neuroergonomics and Cognitive Engineering. Cham: Springer International Publishing; 2017. p. 3-16.
[DOI] -
78. Frank B, Kluge A. Can cued recall by means of gaze guiding replace refresher training? An experimental study addressing complex cognitive skill retrieval. Int J Ind Ergon. 2018;67(1):123-134.
[DOI] -
79. Kluge A, Frank , B . Counteracting skill decay: four refresher interventions and their effect on skill and knowledge retention in a simulated process control task. Ergonomics. 2014;57(2):175-190.
[DOI] -
80. Weyers B, Frank B, Bischof K, Kluge A. Gaze Guiding as Support for the Control of Technical Systems. Int J Inf Syst Crisis Response Manag. 2015;7(2):59-80.
[DOI] -
81. Kluge A, Borisov N, Schüffler A, Weyers B. Augmented Reality to Support Temporal Coordination of Spatial Dispersed Production Teams. In: Mensch und Computer 2018—Workshopband; 2018 Sep 2-5; Dresden, Germany. Germany: Gesellschaft für Informatik e.V.; 2018.
[DOI] -
82. Thomaschewski L, Weyers B, Kluge A. A two-part evaluation approach for measuring the usability and user experience of an Augmented Reality-based assistance system to support the temporal coordination of spatially dispersed teams. Cogn Syst Res. 2021;68(3):1-17.
[DOI] -
83. R Core Team. The R Project for Statistical Computing [Internet]. Vienna: R Foundation for Statistical Computing; 2021 [cited 2024 Mar 31]. Available from: https://www.r-project.org/
-
84. Posit team. A better way to deploy R & Python [Internet]. Boston, MA: Posit Software, PBC, 2023. Available: http://www.posit.co/
-
85. Rubio-Valdehita S, Díaz-Ramiro EM, López-Higes R, Martín-García J. Efectos de la carga de tarea y las capacidades cognitivas sobre el rendimiento y la carga mental subjetiva de una tarea de seguimiento. Ann Psychol. 2012;28(3):986-995.
[DOI] -
86. Grassini S, Laumann K. Are Modern Head-Mounted Displays Sexist? A Systematic Review on Gender Differences in HMD-Mediated Virtual Reality. Front Psychol. 2020;11:1604.
[DOI] -
87. MacArthur C, Grinberg A, Harley D, Hancock M. You're Making Me Sick: A Systematic Review of How Virtual Reality Research Considers Gender & Cybersickness. In: Proceedings of the 2021 CHI Conference on Human Factors in Computing Systems; 2021 May 8-13; Yokohama, Japan. New York: Association for Computing Machinery; 2021. p. 1-15.
[DOI] -
88. Galdas PM, Cheater F, Marshall , P . Men and health help-seeking behaviour: literature review. J Adv Nurs. 2005;49(6):616-623.
[DOI] -
89. Tanaka N, Takagi H. Virtual reality environment design of managing both presence and virtual reality sickness. J Physiol Anthropol Appl Hum Sci. 2004;23(6):313-317.
[DOI] -
90. Grund A, Fries S, Nückles M, Renkl A, Roelle J. When is Learning "Effortful"? Scrutinizing the Concept of Mental Effort in Cognitively Oriented Research from a Motivational Perspective. Educ Psychol Rev. 2024;36(1):43.
[DOI] -
91. Schmidt FL, Hunter J. General mental ability in the world of work: occupational attainment and job performance. J Pers Soc Psychol. 2004;86(1):162-173.
[DOI] -
92. Sweller J. Cognitive load during problem solving: Effects on learning. Cogn Sci. 1988;12(2):257-285.
[DOI] -
93. Wickens CD. Multiple resources and mental workload. Hum Factors. 2008;50(3):449-455.
[DOI] -
94. Bandura A. Self-efficacy: The exercise of control. 12th ed. New York: Freeman; 2012.
-
95. Judge TA, Bono JE. Relationship of core self-evaluations traits—self-esteem, generalized self-efficacy, locus of control, and emotional stability—with job satisfaction and job performance: a meta-analysis. J Appl Psychol. 2001;86(1):80-92.
[DOI] -
96. Hsu CY, Chiou GL, Tsai MJ. Visual behavior and self-efficacy of game playing: an eye movement analysis. Interact Learn Environ. 2019;27(7):942-952.
[DOI] -
97. Arztmann M, Domínguez Alfaro, Hornstra L, Jeuring J, Kester L. In-game performance: The role of students' socio-economic status, self-efficacy and situational interest in an augmented reality game. Br J Educ Technol. 2024;55(2):484-498.
[DOI] -
98. Murman DL. The Impact of Age on Cognition. Semin Hear. 2015;36(3):111-121.
[DOI] -
99. Craik FIM, Bialystok E. Cognition through the lifespan: mechanisms of change. Trends Cogn Sci. 2006;10(3):131-138.
[DOI] -
100. Deci EL, Ryan RM. Intrinsic motivation and self-determination in human behavior. In: Deci EL, Ryan RM, editors. Intrinsic Motivation and Self-Determination in Human Behavior. Boston: Springer; 1985. p. 11-40.
[DOI]
Copyright
© The Author(s) 2025. This is an Open Access article licensed under a Creative Commons Attribution 4.0 International License (https://creativecommons.org/licenses/by/4.0/), which permits unrestricted use, sharing, adaptation, distribution and reproduction in any medium or format, for any purpose, even commercially, as long as you give appropriate credit to the original author(s) and the source, provide a link to the Creative Commons license, and indicate if changes were made.
Publisher's Note
Share And Cite